A Scalable Privacy Preserving Distributed Parallel Optimization For A Large-Scale Aggregation Of Prosumers With Residential Pv-Battery Systems
IEEE ACCESS(2020)
摘要
A novel scalable and privacy-preserving distributed parallel optimization that allows the participation of large-scale aggregation of prosumers with residential PV-battery systems in the market for the ancillary service (ASM) is proposed in this paper. To consider both reserve capacity and energy, day-ahead and real-time stages in the ASM are considered. A method based on hybrid Variable Neighborhood Search (VNS) and distributed parallel optimization is designed for the day ahead and real-time optimization. Different distributed optimization methods are compared and designed and a new distributed optimization method based on Linear Programming (LP) is proposed that outperforms previous methods based on integer and Quadratic programming (QP). The proposed LP-based optimization can be easily coded up and implemented on microcontrollers and connected to a designed Internet of Things (IoT) based architecture. As confirmed by simulation results, carried out considering different realistic case studies, both day-ahead and real-time proposed optimization methods, by allocating the computational effort among local resources, are highly scalable and fulfil the privacy of prosumers.
更多查看译文
关键词
Aggregator, PV-battery systems, battery energy storage systems, up and down-regulation, ancillary services market, linear programming, quadratic programming, distributed optimization, alternating direction method of multipliers (ADMM)
AI 理解论文
溯源树
样例
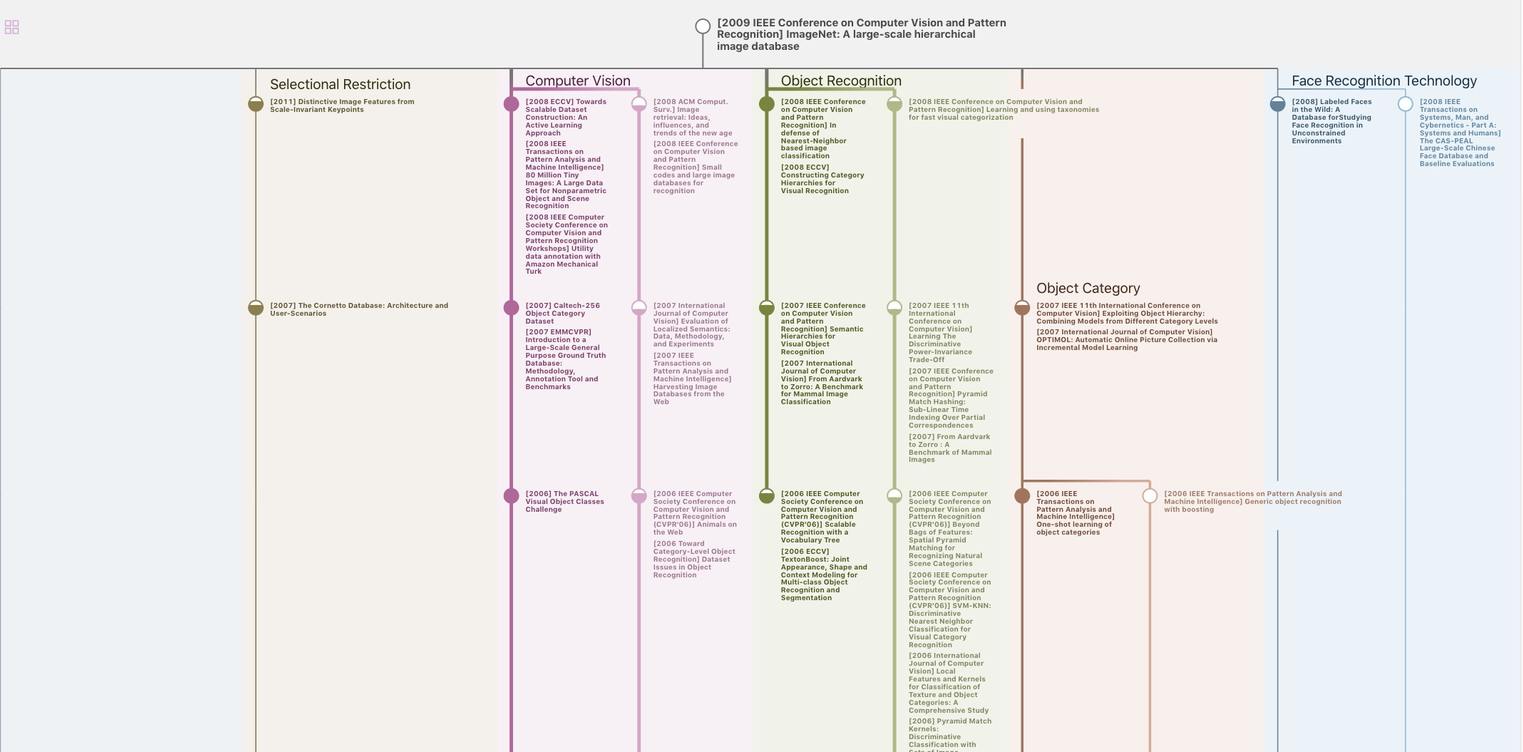
生成溯源树,研究论文发展脉络
Chat Paper
正在生成论文摘要