Attentive Rnn-Based Network To Fuse 12-Lead Ecg And Clinical Features For Improved Myocardial Infarction Diagnosis
IEEE SIGNAL PROCESSING LETTERS(2020)
摘要
The diagnosis of myocardial infarction (MI) in the presence of other cardiac diseases having similar electrocardiogram (ECG) characteristics that of MI is a challenging problem. Existing automated methods have used the standard 12-lead ECG to detect MI from healthy controls (HC). However, these methods may not provide reliable MI diagnosis in the presence of mimicking MI diseases. Therefore, in this letter, we incorporate the patients' clinical features, including age, gender, smoking, hypertension and blood lipid levels, to the 12-lead ECG features for improved classification performance. Specifically, the 12-lead ECG is summarized using weights shared recurrent neural network with intra- and inter-lead attention modules. These modules mimic the clinicians way of diagnosis by focusing on the clinically relevant information to obtain an attentive representative vector. Then, the patients' clinical features are fused with the attentive vector to obtain a discriminate high-level representation vector for a reliable diagnosis. The experimental results on the PTB database indicate that the proposed method achieves an improved overall accuracy of 98.3%. The improved diagnostic accuracy obtained by the fusion of clinical features to the 12-lead ECG makes the proposed method suitable for the pre-screening of MI patients in crowded hospitals.
更多查看译文
关键词
12-lead ECG, attention, classification, clinical features, myocardial infarction, recurrent neural network
AI 理解论文
溯源树
样例
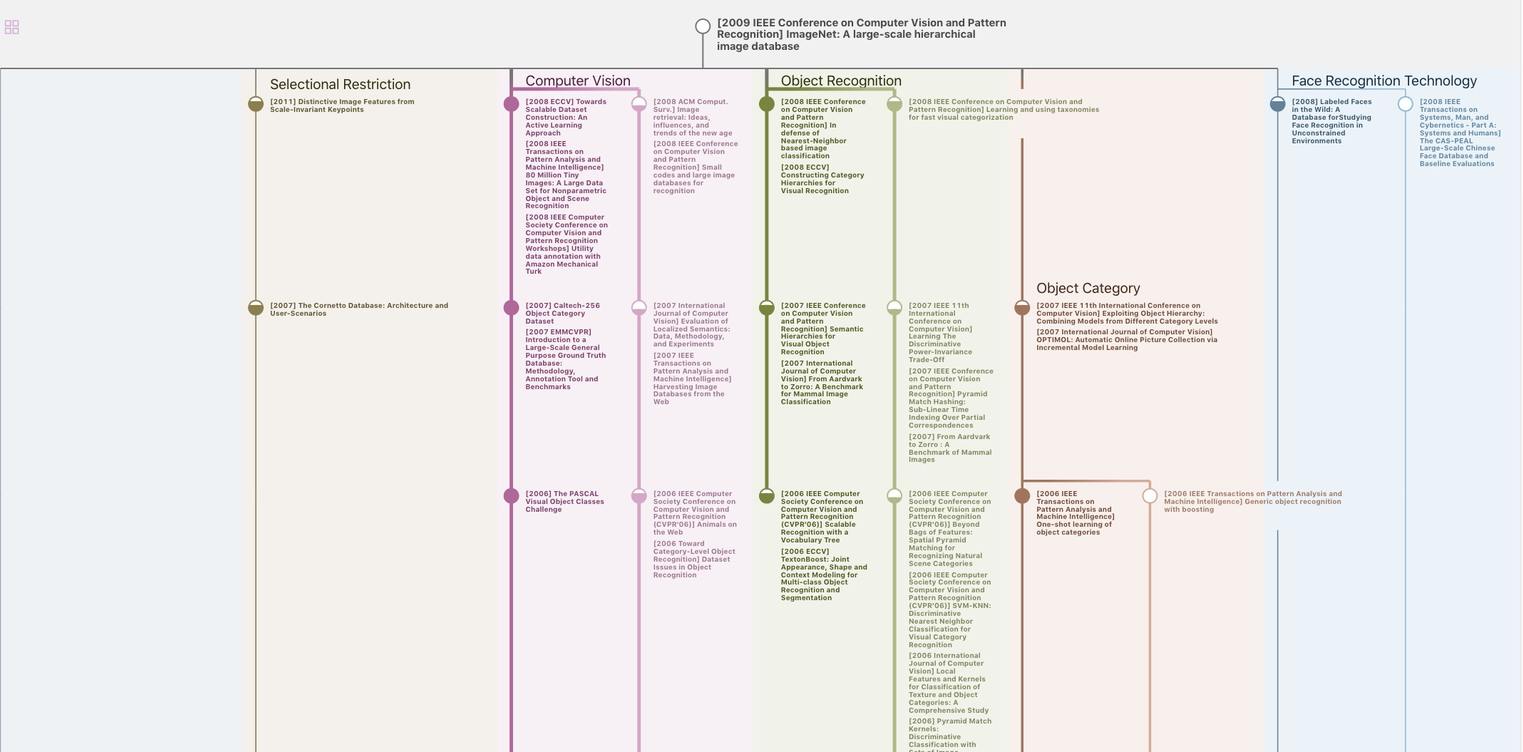
生成溯源树,研究论文发展脉络
Chat Paper
正在生成论文摘要