Conditional simulation of categorical spatial variables using Gibbs sampling of a truncated multivariate normal distribution subject to linear inequality constraints
STOCHASTIC ENVIRONMENTAL RESEARCH AND RISK ASSESSMENT(2020)
摘要
This paper introduces a method to generate conditional categorical simulations, given an ensemble of partially conditioned (or unconditional) categorical simulations derived from any simulation process. The proposed conditioning method relies on implicit functions (signed distance functions) for representing the categorical spatial variable of interest. Thus, the conditioning problem is reformulated in terms of signed distance functions. The proposed approach combines aspects of principal component analysis and Gibbs sampling to achieve the conditioning of the unconditional categorical realizations to the data. It is applied to synthetic and real-world datasets and compared to the traditional sequential indicator simulation. It appears that the proposed simulation technique is an effective method to generate conditional categorical simulations from a set of unconditional categorical simulations.
更多查看译文
关键词
Categorical spatial variable,Conditional simulation,Gibbs sampler,Implicit function,Principal component analysis
AI 理解论文
溯源树
样例
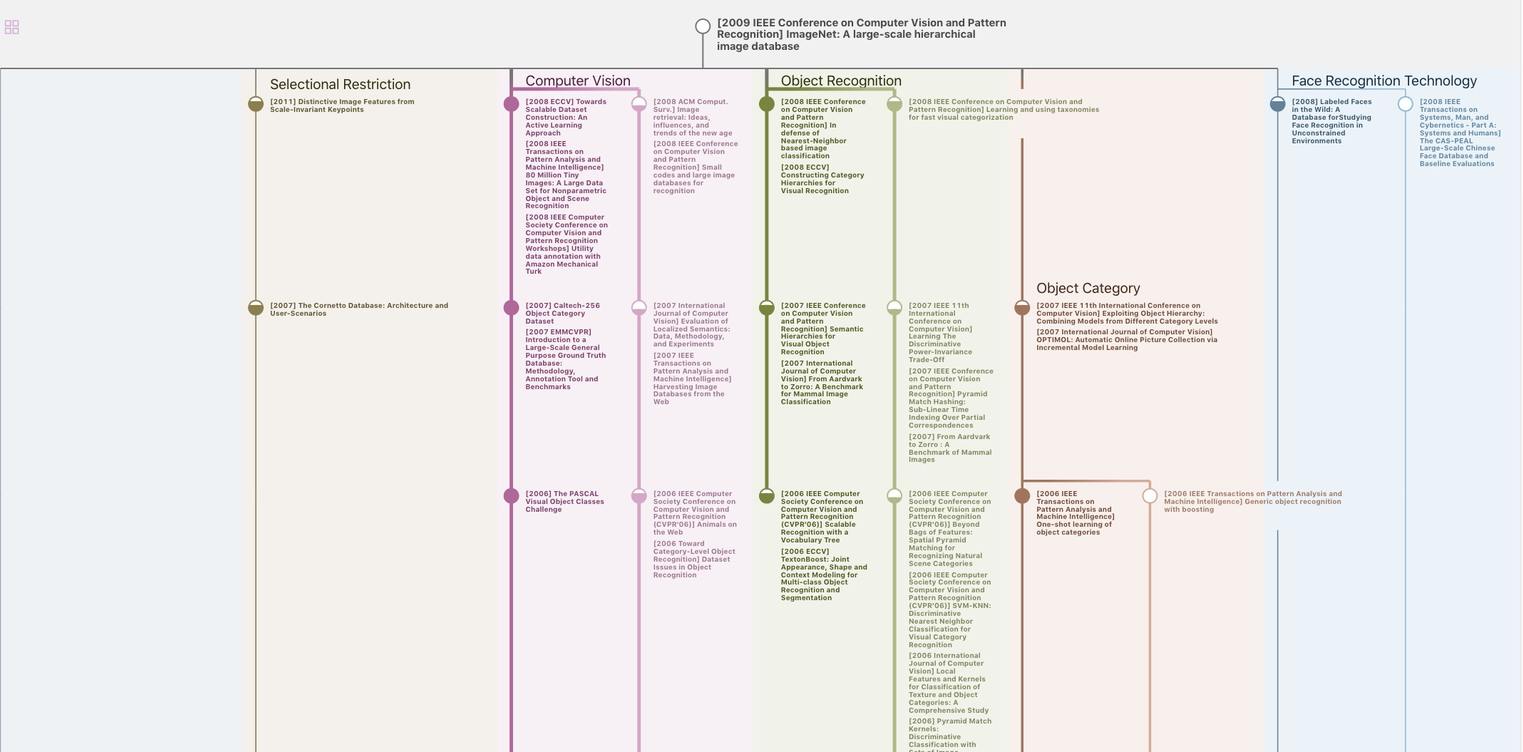
生成溯源树,研究论文发展脉络
Chat Paper
正在生成论文摘要