Knowledge-driven graph similarity for text classification
INTERNATIONAL JOURNAL OF MACHINE LEARNING AND CYBERNETICS(2020)
摘要
Automatic text classification using machine learning is significantly affected by the text representation model. The structural information in text is necessary for natural language understanding, which is usually ignored in vector-based representations. In this paper, we present a graph kernel-based text classification framework which utilises the structural information in text effectively through the weighting and enrichment of a graph-based representation. We introduce weighted co-occurrence graphs to represent text documents, which weight the terms and their dependencies based on their relevance to text classification. We propose a novel method to automatically enrich the weighted graphs using semantic knowledge in the form of a word similarity matrix. The similarity between enriched graphs, knowledge-driven graph similarity , is calculated using a graph kernel. The semantic knowledge in the enriched graphs ensures that the graph kernel goes beyond exact matching of terms and patterns to compute the semantic similarity of documents. In the experiments on sentiment classification and topic classification tasks, our knowledge-driven similarity measure significantly outperforms the baseline text similarity measures on five benchmark text classification datasets.
更多查看译文
关键词
Automatic text classification, Document similarity measure, Graph-based text representation, Graph enrichment, Graph kernels, Supervised term weighting, SVM
AI 理解论文
溯源树
样例
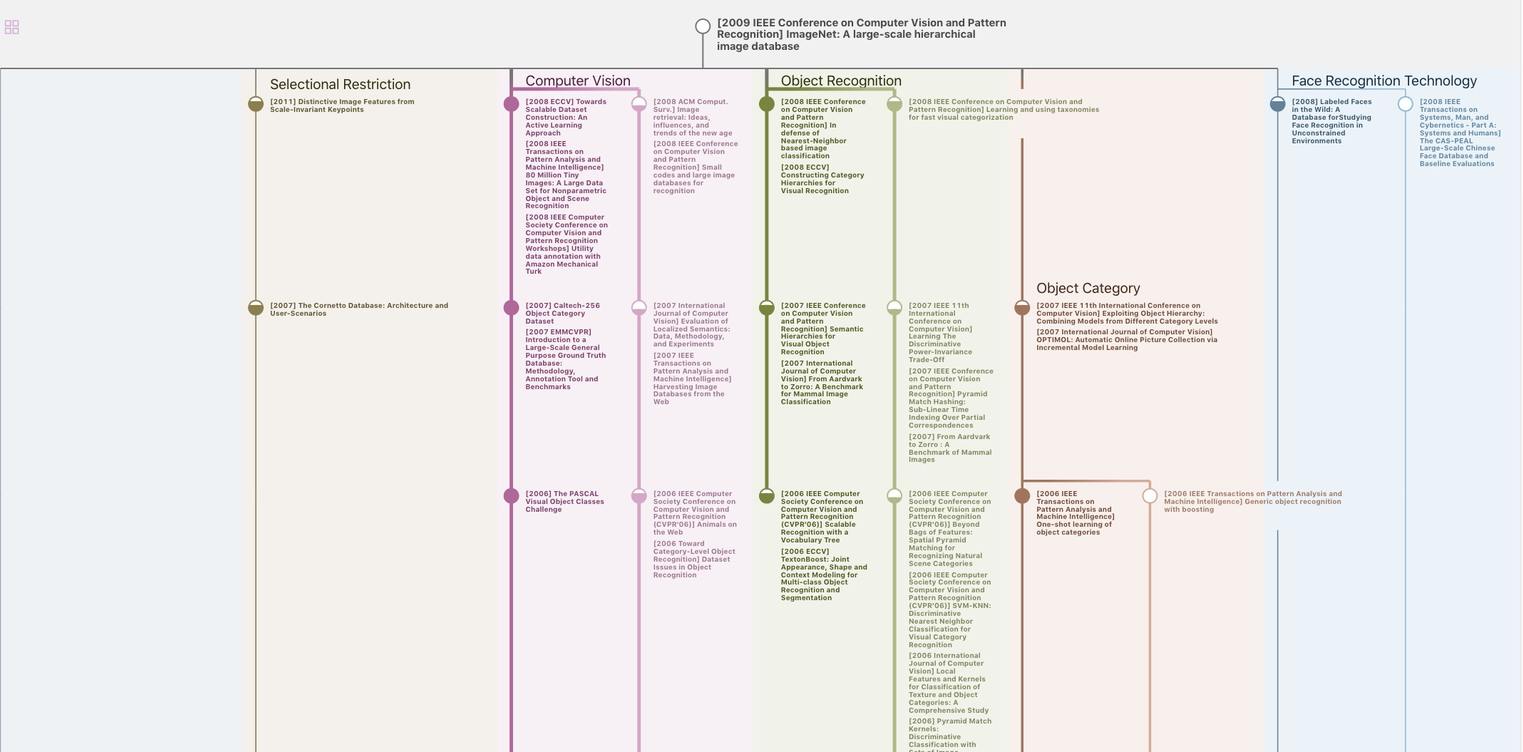
生成溯源树,研究论文发展脉络
Chat Paper
正在生成论文摘要