Extending The Beta Divergence To Complex Values
PATTERN RECOGNITION LETTERS(2021)
摘要
Various information-theoretic divergences have been proposed for the cost function in tasks such as matrix factorization and clustering. One class of divergence is called the Beta divergence. By varying a real-valued parameter beta, the Beta divergence connects several well-known divergences, such as the Euclidean distance, Kullback-Leibler divergence, and Itakura-Saito divergence. Unfortunately, the Beta divergence is properly defined only for positive real values, hindering its use for measuring distances between complex valued data points. We define a new divergence, the Complex Beta divergence, that operates on complex values, and show that it coincides with the standard Beta divergence when the data is restricted to be in phase. Moreover, we show that different values of beta place different penalties on errors in magnitude and phase. (C) 2020 Elsevier B.V. All rights reserved.
更多查看译文
关键词
Information theory, KL divergence, Objective function, Young's inequality
AI 理解论文
溯源树
样例
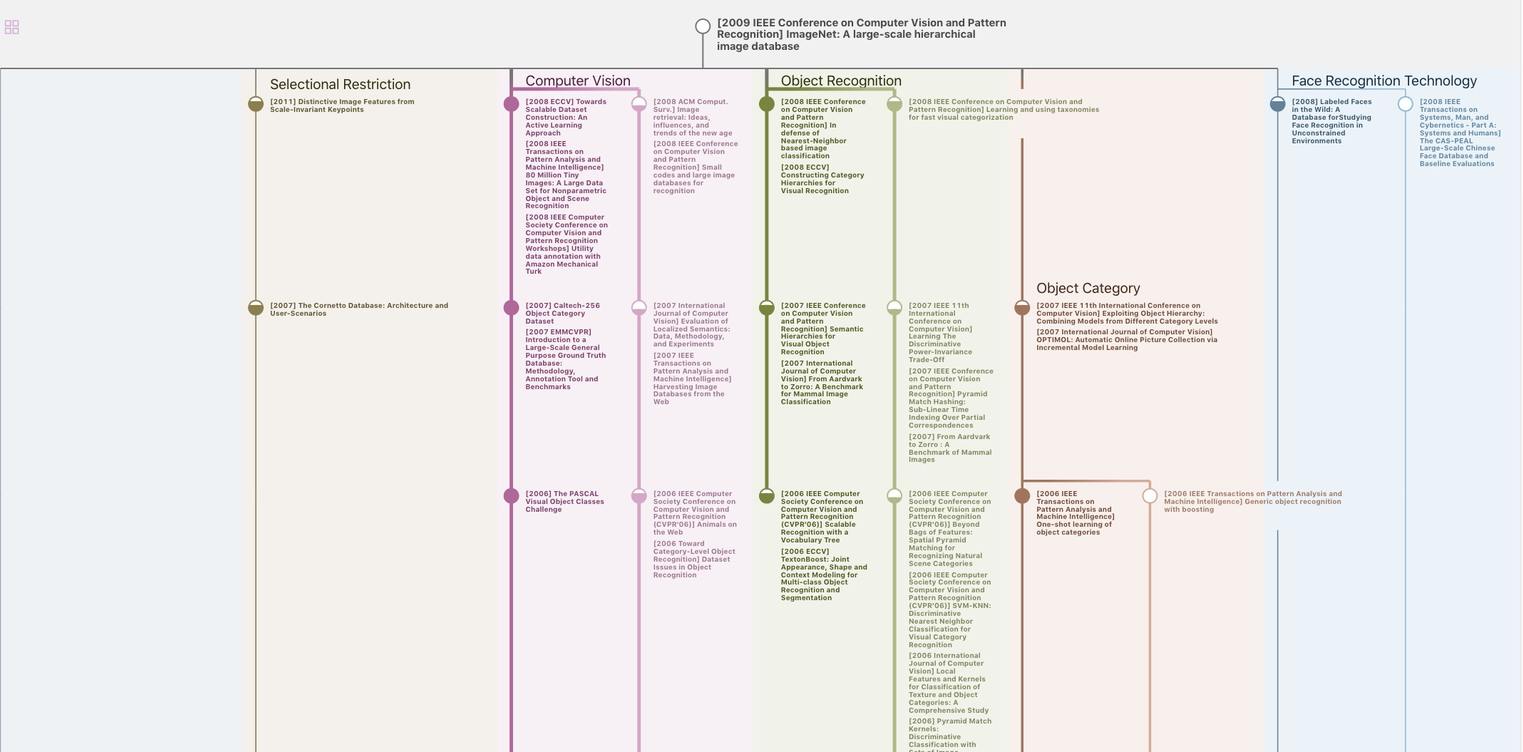
生成溯源树,研究论文发展脉络
Chat Paper
正在生成论文摘要