Deep Learning-Based Short-Term Load Forecasting For Transformers In Distribution Grid
INTERNATIONAL JOURNAL OF COMPUTATIONAL INTELLIGENCE SYSTEMS(2021)
摘要
Load of transformer in distribution grid fluctuates according to many factors, resulting in overload frequently which affects the safety of power grid. And short-term load forecasting is considered. To improve forecasting accuracy, the input information and the model structure are both considered. First, the multi-dimensional information containing numerical data and textual data is taken as the inputs of constructed deep learning model, and textual data is encoded by one-hot method. Then, for the purpose of mining the features of data better, based on the framework composed of convolutional neural network (CNN) and long short-term memory (LSTM), the modified inception structure is introduced to extract more detailed features and adaptive residual connection is added to settle the problem of gradient diffusion when the layers of model grow more. At last, the comparison is carried out and the improvements are presented after the textual data is added and the structure of model is modified. And the forecasting error is reduced, especially when the load is heavy, which is beneficial for the prevention of overload of transformer in distribution gird. (C) 2021 The Authors. Published by Atlantis Press B.V.
更多查看译文
关键词
Load forecasting, convolutional neural network (CNN), Long short-term memory (LSTM), Inception structure, Residual connection
AI 理解论文
溯源树
样例
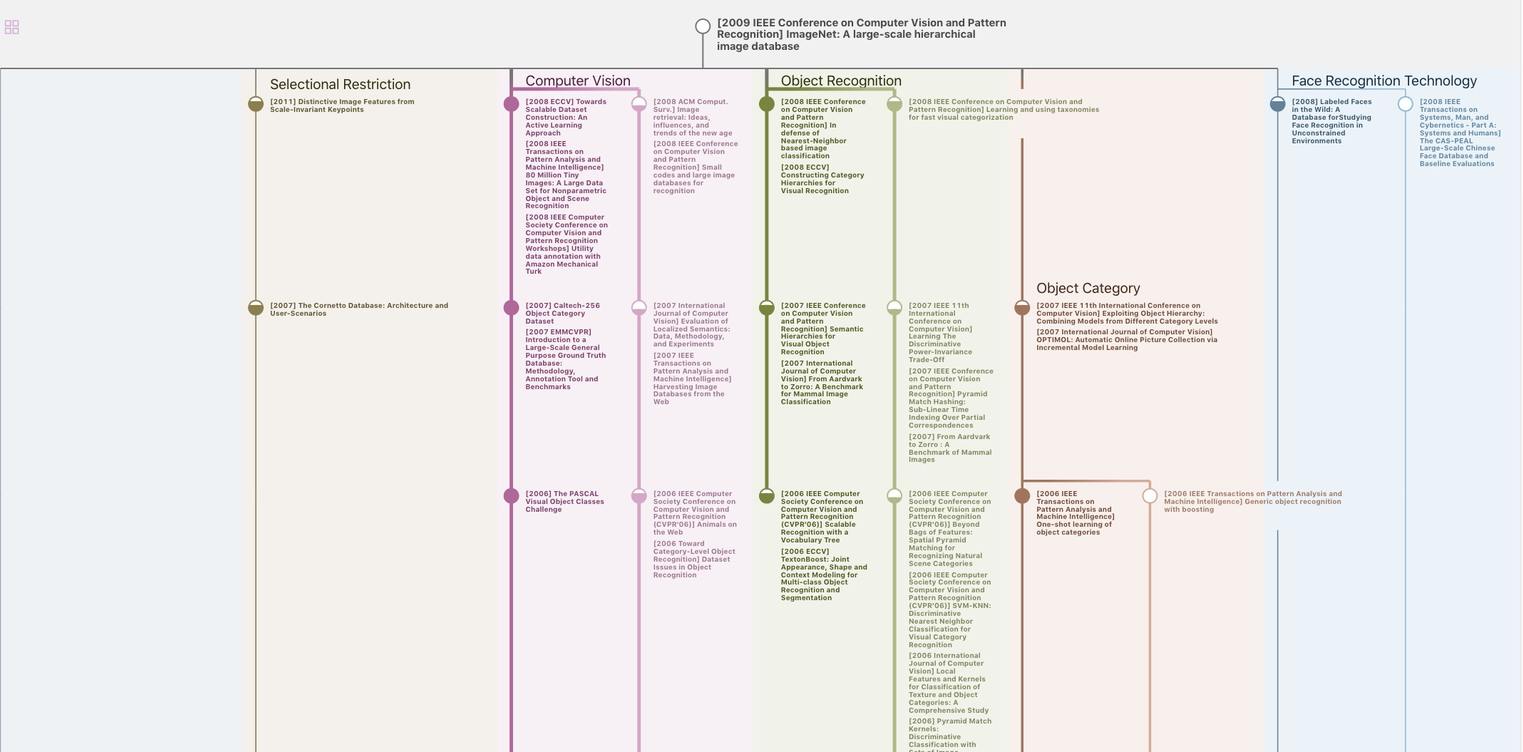
生成溯源树,研究论文发展脉络
Chat Paper
正在生成论文摘要