A Comparative Study Of Methods For A Priori Prediction Of Mcq Difficulty
SEMANTIC WEB(2021)
摘要
Successful exams require a balance of easy, medium, and difficult questions. Question difficulty is generally either estimated by an expert or determined after an exam is taken. The latter provides no utility for the generation of new questions and the former is expensive both in terms of time and cost. Additionally, it is not known whether expert prediction is indeed a good proxy for estimating question difficulty.In this paper, we analyse and compare two ontology-based measures for difficulty prediction of multiple choice questions, as well as comparing each measure with expert prediction (by 15 experts) against the exam performance of 12 residents over a corpus of 231 medical case-based questions that are in multiple choice format. We find one ontology-based measure (relation strength indicativeness) to be of comparable performance (accuracy = 47%) to expert prediction (average accuracy = 49%).
更多查看译文
关键词
Ontologies, semantic web, automatic question generation, difficulty modelling, difficulty prediction, multiple choice questions, student assessment
AI 理解论文
溯源树
样例
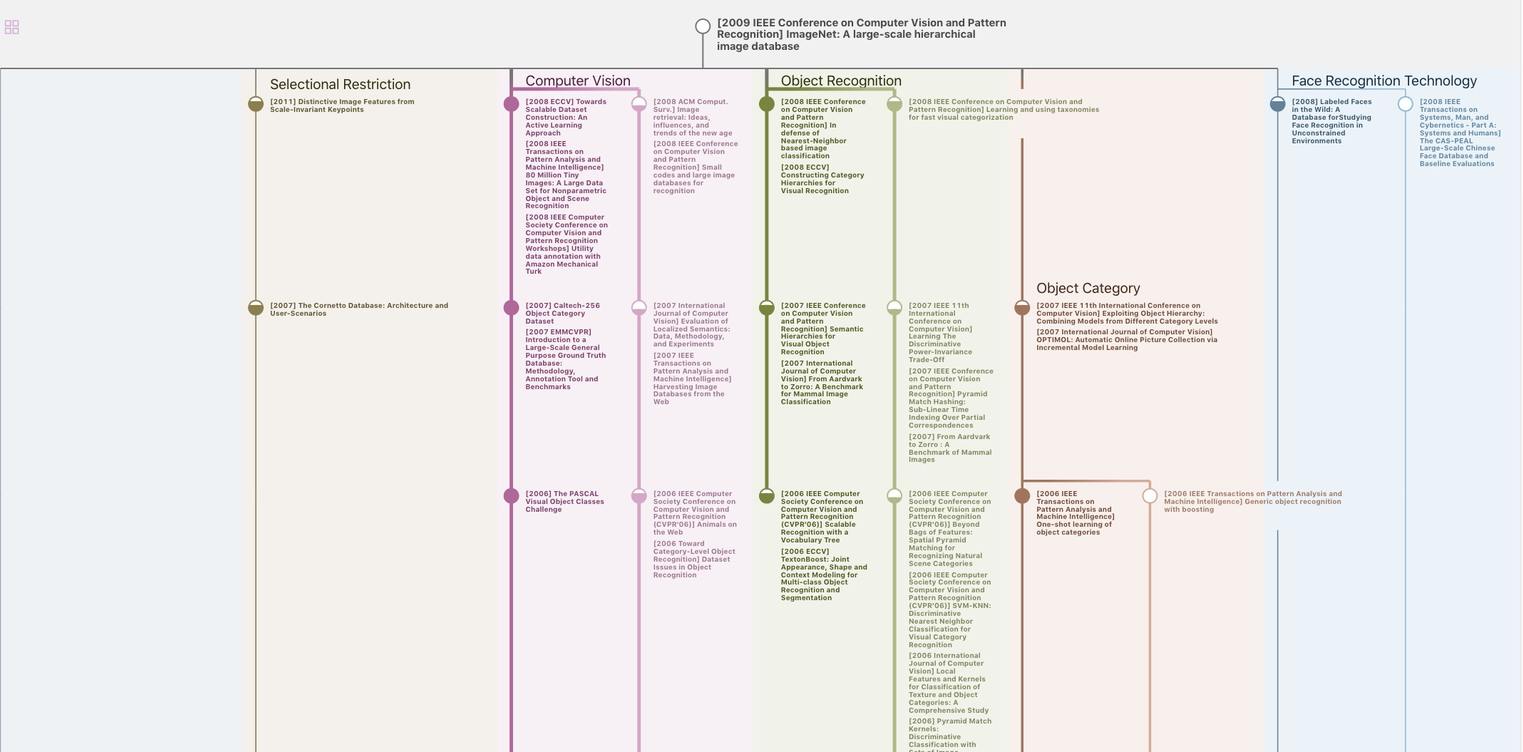
生成溯源树,研究论文发展脉络
Chat Paper
正在生成论文摘要