Qos-Aware Workload Distribution In Hierarchical Edge Clouds: A Reinforcement Learning Approach
IEEE ACCESS(2020)
摘要
Recently, edge computing is getting attention as a new computing paradigm that is expected to achieve short-delay and high-throughput task offloading for large scale Internet-of-Things (IoT) applications. In edge computing, workload distribution is one of the most critical issues that largely influences the delay and throughput performance of edge clouds, especially in distributed Function-as-a-Service (FaaS) over networked edge nodes. In this paper, we propose the Resource Allocation Control Engine with Reinforcement learning (RACER), which provides an efficient workload distribution strategy to reduce the task response slowdown with per-task response time Quality-of-Service (QoS). First, we present a novel problem formulation with the per-task QoS constraint derived from the well-known token bucket mechanism. Second, we employ a problem relaxation to reduce the overall computation complexity by compromising just a bit of optimality. Lastly, we take the deep reinforcement learning approach as an alternative solution to the workload distribution problem to cope with the uncertainty and dynamicity of underlying environments. Evaluation results show that RACER achieves a significant improvement in terms of per-task QoS violation ratio, average slowdown, and control efficiency, compared to AREA, a state-of-the-art workload distribution method.
更多查看译文
关键词
Quality of service, Cloud computing, Time factors, Task analysis, Resource management, Edge computing, Reinforcement learning, Deep reinforcement learning, edge computing, resource allocation, workload distribution
AI 理解论文
溯源树
样例
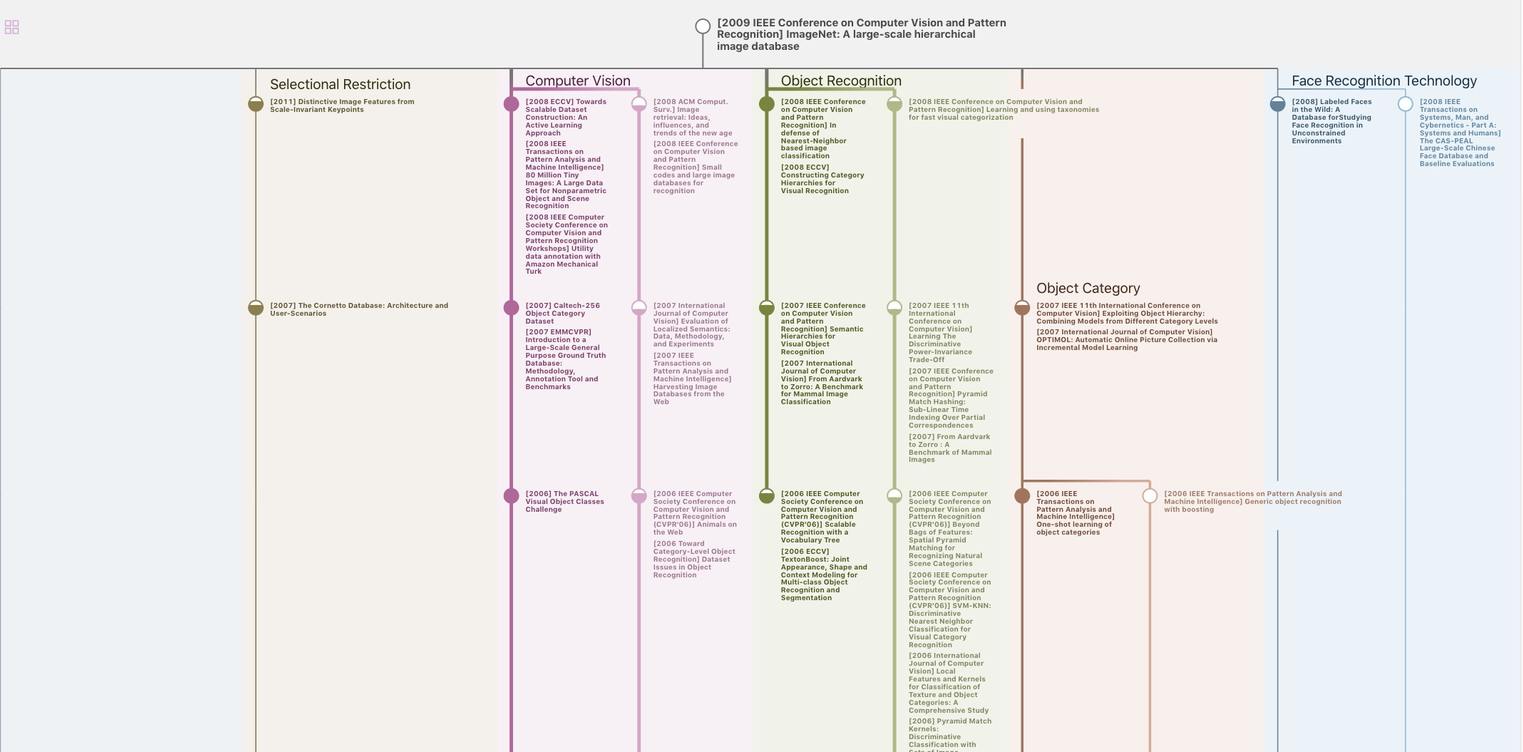
生成溯源树,研究论文发展脉络
Chat Paper
正在生成论文摘要