A Deep Learning Model With Capsules Embedded for High-Resolution Image Classification
IEEE Journal of Selected Topics in Applied Earth Observations and Remote Sensing(2021)
摘要
Classification of remote sensing (RS) images is a key technology for extracting information on ground objects using RS methods. Inspired by the success of deep learning (DL) in artificial intelligence, researchers have proposed different algorithms based on DL to improve the performance of classification. At present, a DL model represented by the convolutional neural networks (CNNs) can extract the abstract feature, but it loses the spatial context of the ground objects. To solve the problem of lack of spatial information in CNNs, the Capsule network takes the form of vectors that convey location transformation information. This article proposes using a Capsules-Unet model, which incorporates Capsules within the U-net architecture for classification of RS images. The aim is to train better models by encapsulating the multidimensional features of the objects in the form of Capsules, and to reduce parameter space by improving the dynamic routing algorithm. Experiments are conducted on ISPRS Vaihingen and Potsdam datasets. Capsules-Unet slightly outperforms all other approaches with far fewer parameters, a reduction in parameters of over 81.8% compared with U-net and over 13.8% compared with Capsule network.
更多查看译文
关键词
Capsules–Unet,classification,deep learning (DL),remote sensing (RS)
AI 理解论文
溯源树
样例
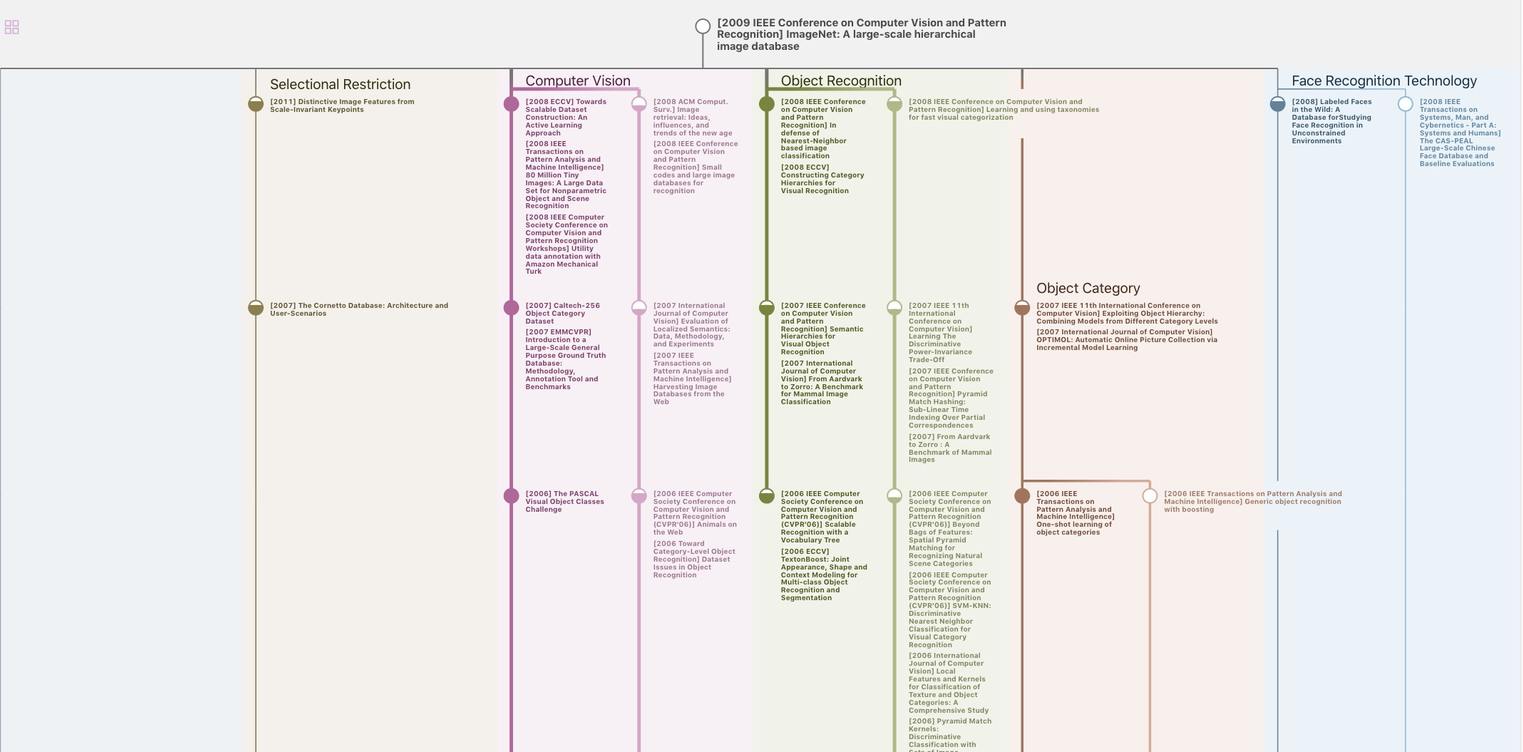
生成溯源树,研究论文发展脉络
Chat Paper
正在生成论文摘要