Regional ionospheric parameter estimation by assimilating the LSTM trained results into the SAMI2 model
SPACE WEATHER-THE INTERNATIONAL JOURNAL OF RESEARCH AND APPLICATIONS(2020)
摘要
This paper presents a study on the possibility of predicting the regional ionosphere at midlatitude by assimilating the predicted ionospheric parameters from a neural network (NN) model into the Sami2 is Another Model of the Ionosphere (SAMI2). The NN model was constructed from the data set of Jeju ionosonde (33.43 degrees N, 126.30 degrees E) for the period of 1 January 2011 to 31 December 2015 by using the long-short term memory (LSTM) algorithm. The NN model provides 24-hr prediction of the peak density (NmF2) and peak height (hmF2) of the F2 layer over Jeju. The predicted NmF2 and hmF2 were used to compute two ionospheric drivers (total ion density and effective neutral meridional wind), which were assimilated into the SAMI2 model. The SAMI2-LSTM model estimates the ionospheric conditions over the midlatitude region around Jeju on the same geomagnetic meridional plane. We evaluate the performance of the SAMI2-LSTM by comparing predicted NmF2 and hmF2 values with measured values during the geomagnetic quiet and storm periods. The root-mean-square error values of NmF2 (hmF2) from Jeju ionosonde measurements are lower by 45% and 45% (30% and 11%) than those of the SAMI2 and IRI-2016 models during the geomagnetic quiet periods. However, during the geomagnetic storm periods, the performance of the SAMI2-LSTM model does not predict positive geomagnetic storms well. Comparing the quiet and storm periods for the SAMI2-LSTM model, the root-mean-square error (RMSE) of the storm period was calculated to be 2.76 (3.2) times higher at Jeju (Icheon) than in the quiet period. From these results, we demonstrated that in this study, the combination of the NN-LSTM model and physics-based model could improve the ionosphere prediction of existing theoretical and empirical models for midlatitude regions, at least in geomagnetically quiet conditions. We strongly suggest that this attempt, which has not been reported before, could be used as one of the keys to advance the physics-based model further.
更多查看译文
关键词
ionosphere,forecast model,SAMI2 model,LSTM algorithm,assimilation technique
AI 理解论文
溯源树
样例
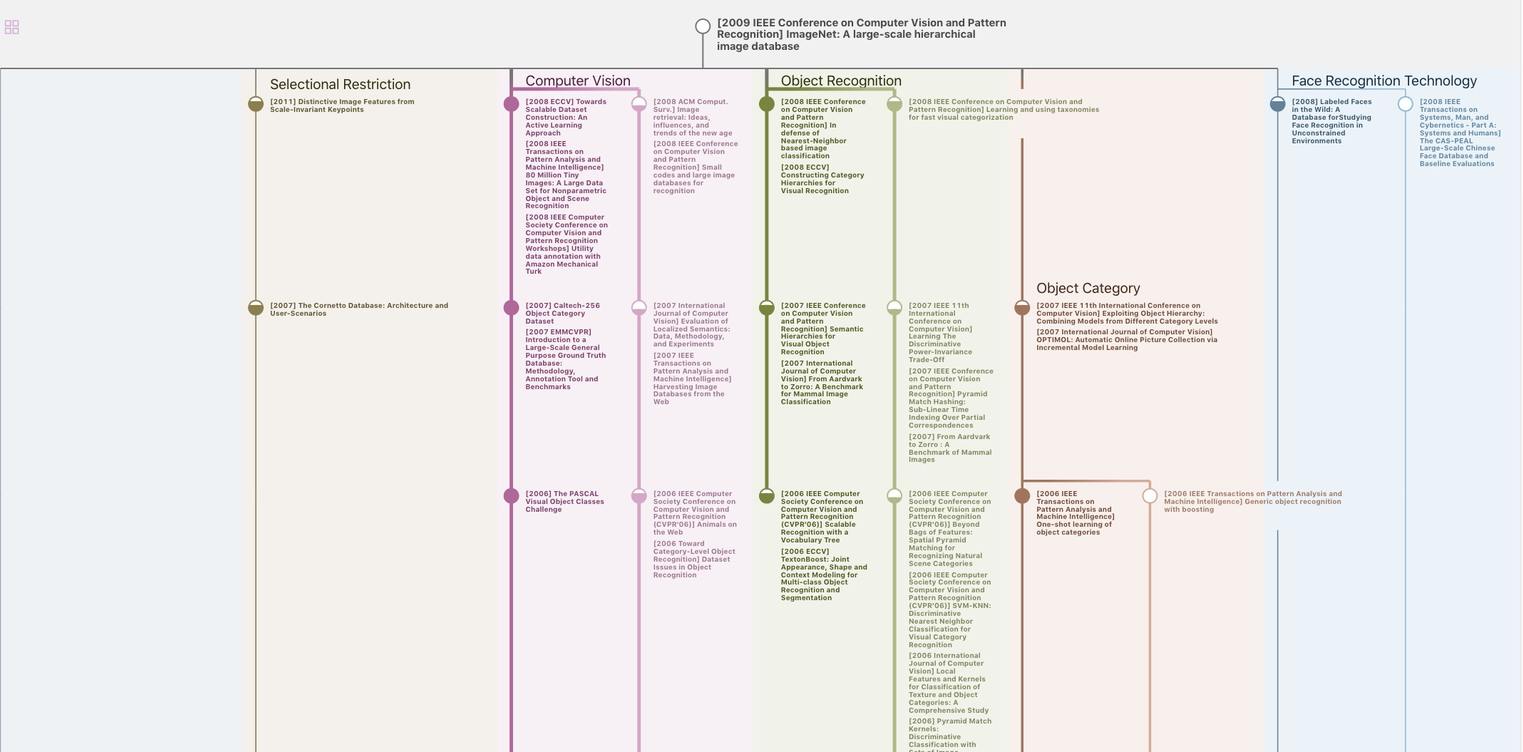
生成溯源树,研究论文发展脉络
Chat Paper
正在生成论文摘要