A Bayesian approach for inferring global points of departure from transcriptomics data
Computational Toxicology(2020)
摘要
Abstract Bayesian statistical methods allow for robust scientific inferences. Increased robustness is achieved by using prior distributions to regularise parameter estimates and by defining a model structure which accurately reflects the variance structure of the dataset of interest. We develop a Bayesian model to describe transcriptomics concentration–response data. This model is designed to infer gene-level points of departure and a method to derive a global point of departure from these thousands of gene level estimates is presented. We believe such estimates may prove useful for characterising maximum no effect concentrations for the purposes of hazard identification in next generation risk assessment.
更多查看译文
关键词
Bayesian,Transcriptomics,Concentration–response,Point-of-departure,NAM,NGRA
AI 理解论文
溯源树
样例
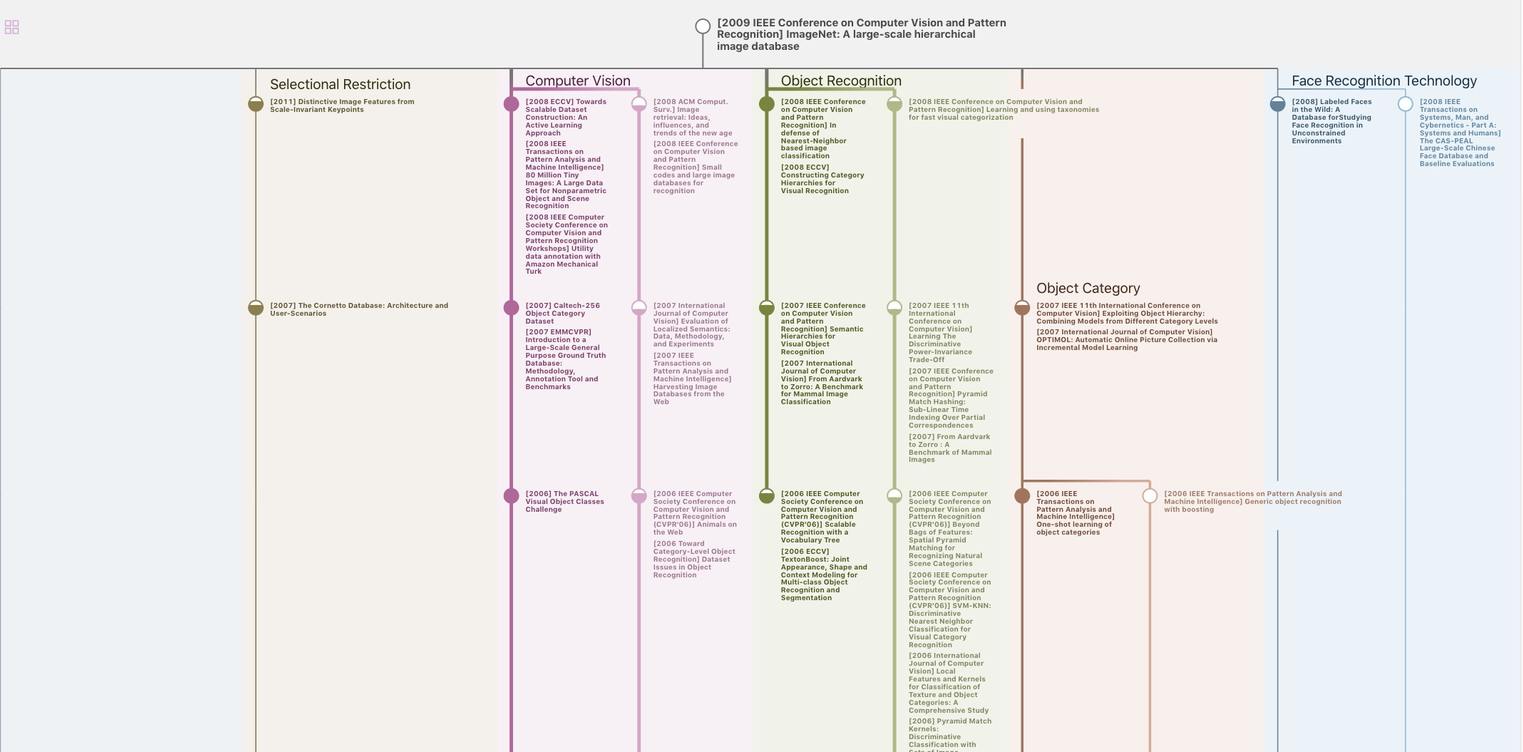
生成溯源树,研究论文发展脉络
Chat Paper
正在生成论文摘要