A Learning-Based Harmonic Mapping: Framework, Assessment, And Case Study Of Human-To-Robot Hand Pose Mapping
INTERNATIONAL JOURNAL OF ROBOTICS RESEARCH(2021)
摘要
Harmonic mapping provides a natural way of mapping two manifolds by minimizing distortion induced by the mapping. However, most applications are limited to mapping between 2D and/or 3D spaces owing to the high computational cost. We propose a novel approach, the harmonic autoencoder (HAE), by approximating a harmonic mapping in a data-driven way. The HAE learns a mapping from an input domain to a target domain that minimizes distortion and requires only a small number of input-target reference pairs. The HAE can be applied to high-dimensional applications, such as human-to-robot hand pose mapping. Our method can map from the input to the target domain while minimizing distortion over the input samples, covering the target domain, and satisfying the reference pairs. This is achieved by extending an existing neural network method called the contractive autoencoder. Starting from a contractive autoencoder, the HAE takes into account a distance function between point clouds within the input and target domains, in addition to a penalty for estimation error on reference points. For efficiently selecting a set of input-target reference pairs during the training process, we introduce an adaptive optimization criterion. We demonstrate that pairs selected in this way yield a higher-performance mapping than pairs selected randomly, and the mapping is comparable to that from pairs selected heuristically by the experimenter. Our experimental results with synthetic data and human-to-robot hand pose data demonstrate that our method can learn an effective mapping between the input and target domains.
更多查看译文
关键词
Learning and adaptive system, human-to-robot hand pose mapping, harmonic mapping, contractive autoencoder
AI 理解论文
溯源树
样例
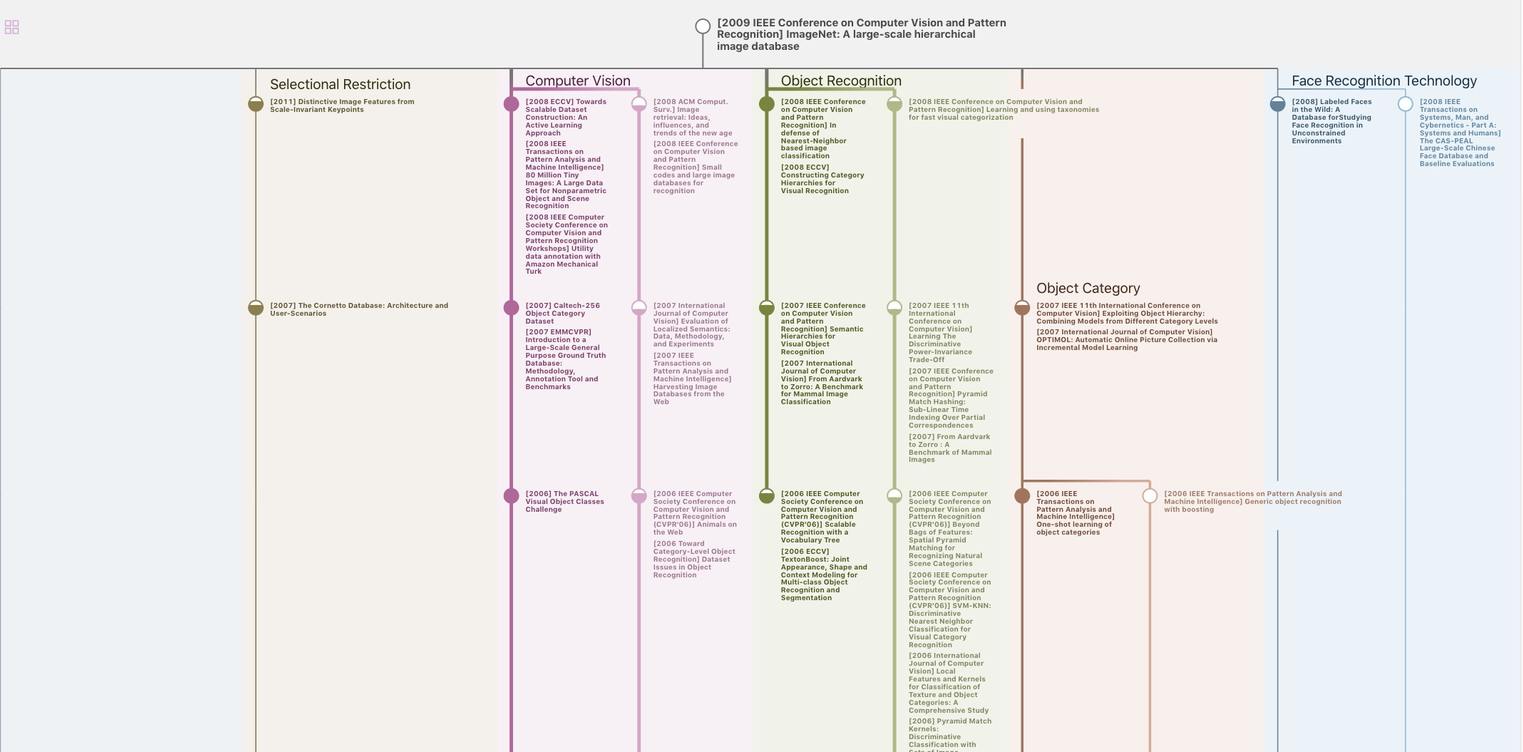
生成溯源树,研究论文发展脉络
Chat Paper
正在生成论文摘要