Instance segmentation in fisheye images
2020 Tenth International Conference on Image Processing Theory, Tools and Applications (IPTA)(2020)
摘要
In this paper we propose a data augmentation method for instance segmentation in fisheye images. A lot of progress has been made on the task of instance segmentation in the last few years, particularly for rectilinear images. In fisheye images, detection tasks have mostly been explored as a semantic segmentation task. Instance segmentation in fisheye images is challenging and has not yet been fully explored. There is also much interest in the development of deep neural networks that can handle both rectilinear and fisheye images. Indeed, this can be interesting to have control on computing resource requirements, of paramount importance for real-time systems e.g., in transportation systems. This paper aims to explore these two challenges using Mask R-CNN trained with a data augmentation method designed to provide good performance on both rectilinear and fisheye images. We show that performance on fisheye augmented images can be increased by 9% while only decreasing performance on rectilinear images by 2%, and that performance on wide angle fisheye cameras can be increased by 18.4% compared to the reference, which provides more benefits than a simple vertical flip augmentation.
更多查看译文
关键词
fisheye images,instance segmentation,deep learning,data augmentation
AI 理解论文
溯源树
样例
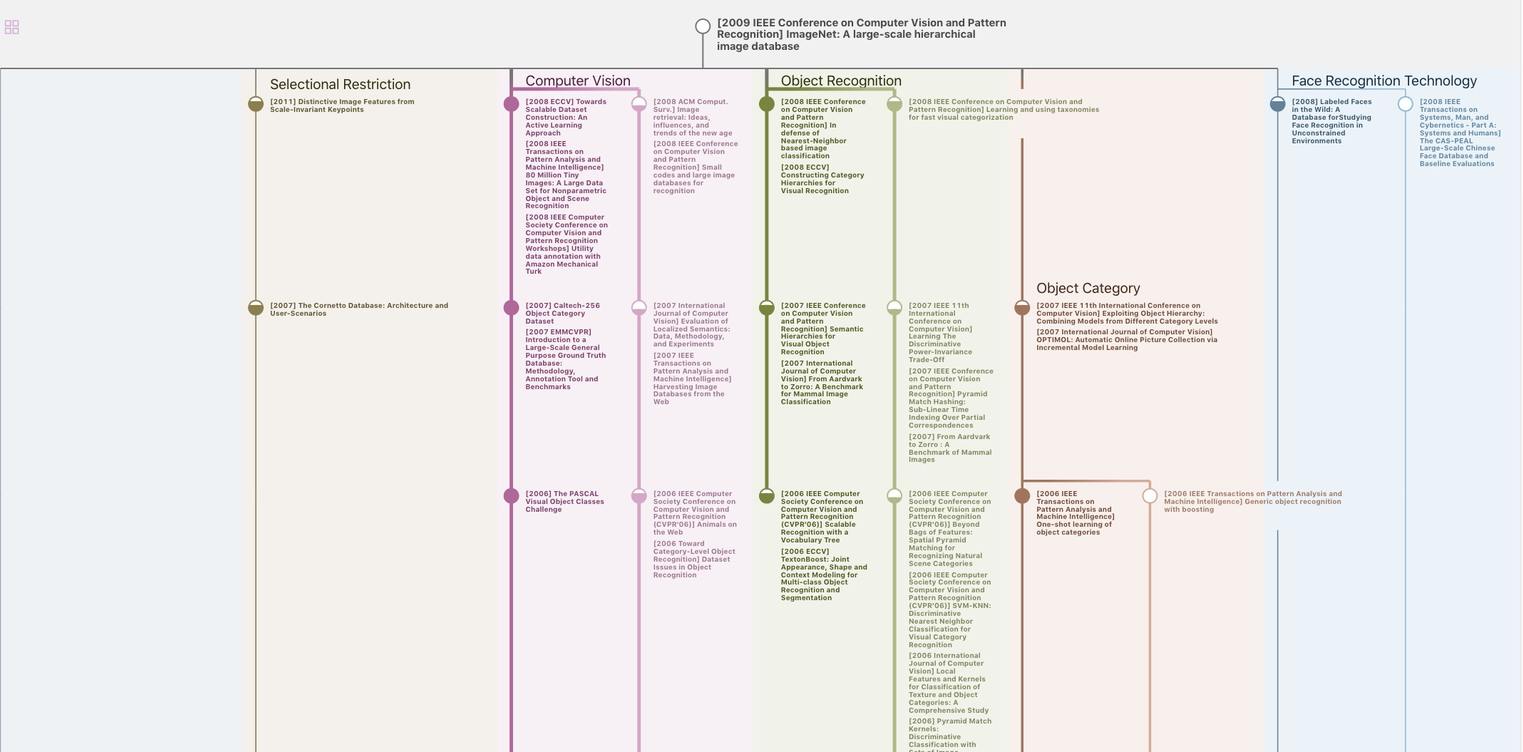
生成溯源树,研究论文发展脉络
Chat Paper
正在生成论文摘要