Dynamic pricing in profit-driven task assignment: a domain-of-influence based approach
INTERNATIONAL JOURNAL OF MACHINE LEARNING AND CYBERNETICS(2020)
摘要
The development of mobile Internet and sharing economy brings the prosperity of spatial crowdsourcing (SC). Pricing is a crucial step for SC platforms to solve the Profit-driven Task Assignment (PTA) problem to maximize their total profit. However, dynamic pricing is still large unexplored in PTA. In addition, existing works seek solutions without considering the uncertainty of workers’ acceptance for assigned tasks in the task assignment process. To deal with these challenges, we develop a two-stage task assignment framework with dynamic pricing. Specifically, we propose a novel Domain-of-Influence based dynamic pricing algorithm, which can iteratively figure out the price that represents the balance between task demand and worker supply. Then we employ hyperbolic temporal discounting function to estimate the worker’s psychological reward that indicates the acceptance or rejection of assigned task. With considering the driver’s psychological reward, we adopt an optimal algorithm to achieve the optimal task assignment and propose greedy algorithms to improve the computational efficiency. Finally, we evaluate the performance using two road network datasets of Jinan and Luoyang in China. The experimental results show the effectiveness and efficiency of our proposed approaches.
更多查看译文
关键词
Spatial crowdsourcing,Task assignment,Dynamic pricing,Domain-of-Influence,Psychological reward
AI 理解论文
溯源树
样例
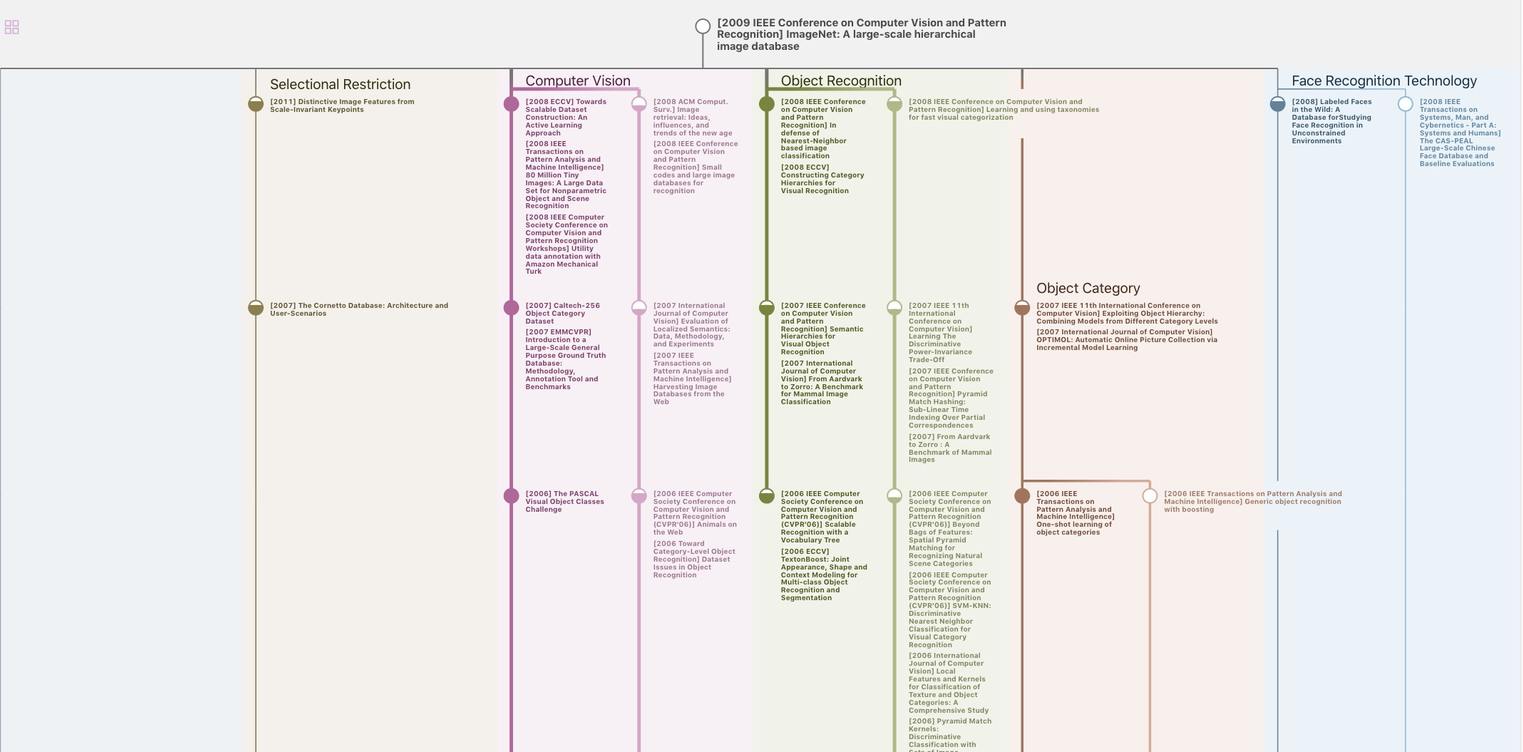
生成溯源树,研究论文发展脉络
Chat Paper
正在生成论文摘要