Dependency Parsing with Noisy Multi-annotation Data
international conference natural language processing(2020)
摘要
In the past few years, performance of dependency parsing has been improved by large margin on closed-domain benchmark datasets. However, when processing real-life texts, parsing performance degrades dramatically. Besides the domain adaptation technique, which has made slow progress due to its intrinsic difficulty, one straightforward way is to annotate a certain scale of syntactic data given a new source of texts. However, it is well known that annotating data is time and effort consuming, especially for the complex syntactic annotation. Inspired by the progress in crowdsourcing, this paper proposes to annotate noisy multi-annotation syntactic data with non-experts annotators. Each sentence is independently annotated by multiple annotators and the inconsistencies are retained. In this way, we can annotate data very rapidly since we can recruit many ordinary annotators. Then we construct and release three multi-annotation datasets from different sources. Finally, we propose and compare several benchmark approaches to training dependency parsers on such multi-annotation data. We will release our code and data at http://hlt.suda.edu.cn/~zhli/.
更多查看译文
关键词
dependency,multi-annotation
AI 理解论文
溯源树
样例
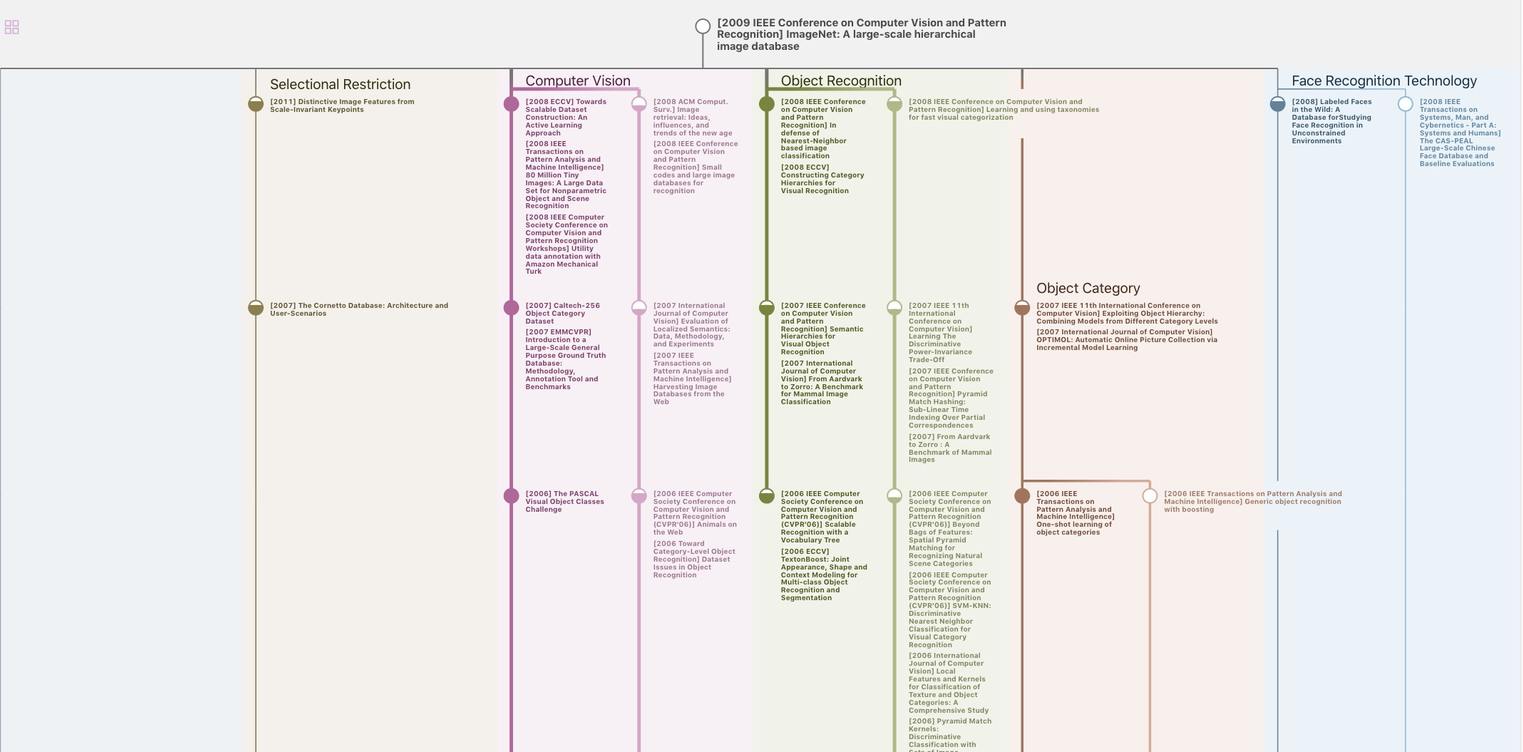
生成溯源树,研究论文发展脉络
Chat Paper
正在生成论文摘要