Soil Variability And Quantification Based On Sentinel-2 And Landsat-8 Bare Soil Images: A Comparison
REMOTE SENSING OF ENVIRONMENT(2021)
摘要
There is a worldwide need for detailed spatial information to support soil mapping, mainly in the tropics, where main agricultural areas are concentrated. In this line, satellite images are useful tools that can assist in obtaining soil information from a synoptic point of view. This study aimed at evaluating how satellite images at different resolutions (spatial, spectral and temporal) can influence the representation of soil variability over time, the percentage of bare soil areas and spatial predictions of soil properties in southeastern Brazil. We used single-date and multi-temporal images (SYSI, Synthetic Soil Images) of bare soil pixels from the Sentinel2-MultiSpectral Instrument (S2-MSI) and the Landsat-8 Operational Land Imager (L8-OLI) to conduct this research. Two SYSIs were obtained from images acquired in four years (2016-2019) for each satellite (SYSI S2-MSI and SYSI L8-OLI) and a third SYSI, named SYSI Combined, was obtained by combining the images from both satellites. The single date images for each satellite was acquired in September, when the influence of clouds was low and bare soil pixels was predominant. Single-date images and SYSIs were compared by means of their spectral patterns and ability to predict topsoil properties (clay, sand, silt, and organic matter contents and soil color) using the Cubist algorithm. We found that the SYSIs outperformed single-date images and that the SYSI Combined and SYSI L8OLI provided the best prediction performances. The SYSIs also had the highest percentage of areas with bare soil pixels (similar to 30-50%) when compared to the single-date images (similar to 20%). Our results suggest that bare soil images obtained by combining Landsat-8 and Sentinel-2 images are more important for soil mapping than spatial or spectral resolutions. Soil maps obtained via satellite images are important tools for soil survey, land planning, management and precision agriculture.
更多查看译文
关键词
Bare soil pixels, Digital soil mapping, Machine learning, Remote sensing, Spatial resolution, Spectral resolution, Time series
AI 理解论文
溯源树
样例
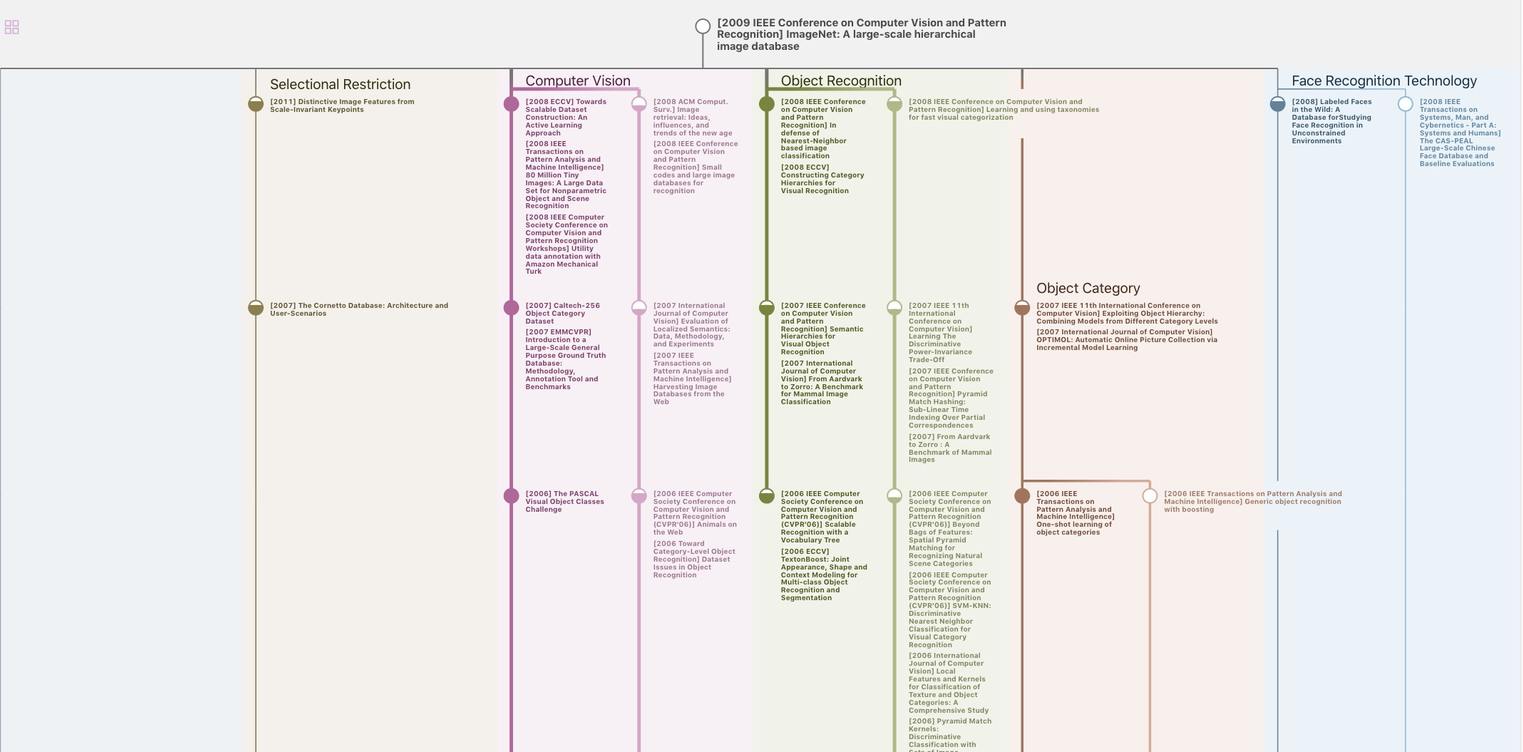
生成溯源树,研究论文发展脉络
Chat Paper
正在生成论文摘要