Adversarial BiLSTM-CRF Architectures for Extra-Propositional Scope Resolution.
international conference natural language processing(2020)
摘要
Due to the ability of expressively representing narrative structures, proposition-aware learning models in text have been drawing more and more attentions in information extraction. Following this trend, recent studies go deeper into learning fine-grained extra-propositional structures, such as negation and speculation. However, most of elaborately-designed experiments reveal that existing extra-proposition models either fail to learn from the context or neglect to address cross-domain adaptation. In this paper, we attempt to systematically address the above challenges via an adversarial BiLSTM-CRF model, to jointly model the potential extra-propositions and their contexts. This is motivated by the superiority of sequential architecture in effectively encoding order information and long-range context dependency. On the basis, we come up with an adversarial neural architecture to learn the invariant and discriminative latent features across domains. Experimental results on the standard BioScope corpus show the superiority of the proposed neural architecture, which significantly outperforms the state-of-the-art on scope resolution in both in-domain and cross-domain scenarios.
更多查看译文
关键词
scope,bilstm-crf,extra-propositional
AI 理解论文
溯源树
样例
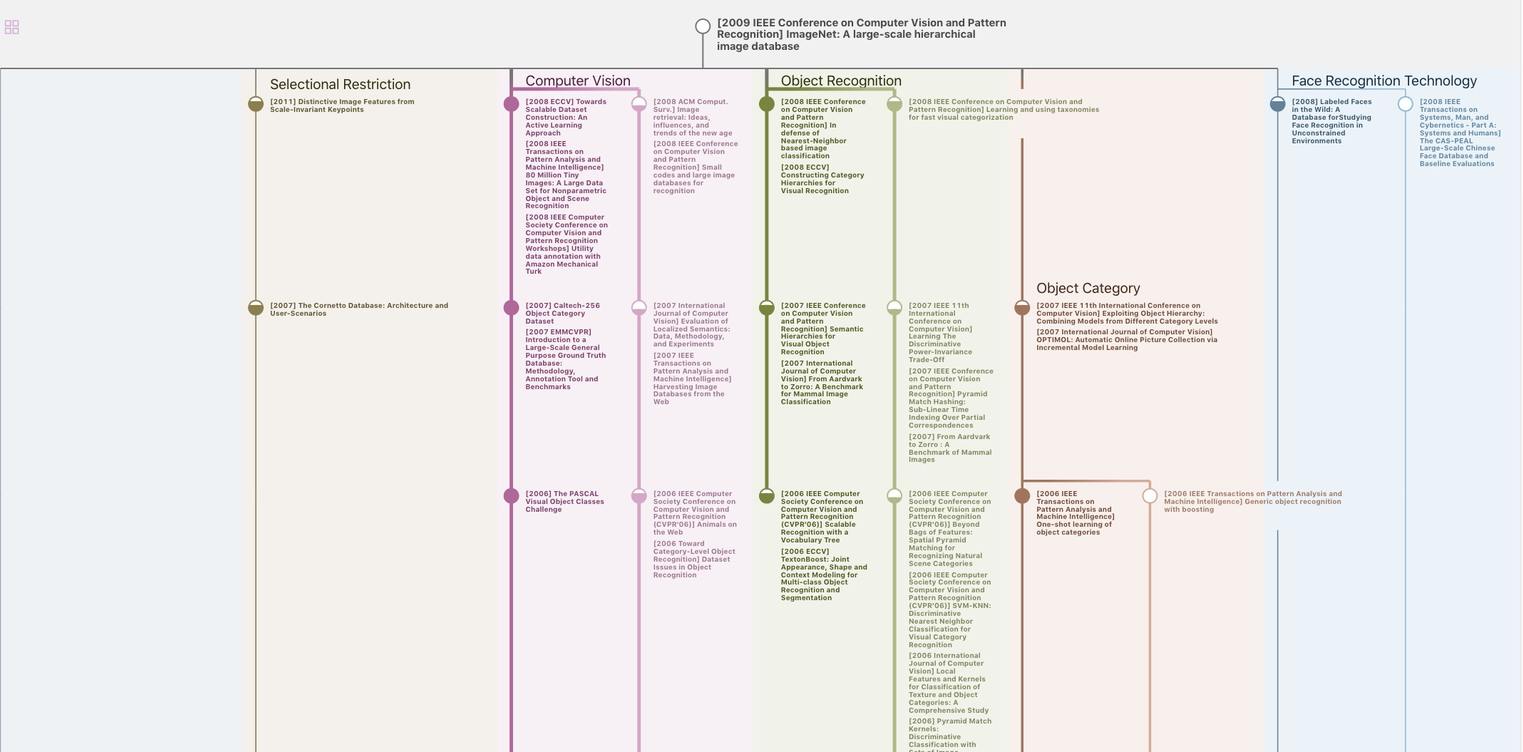
生成溯源树,研究论文发展脉络
Chat Paper
正在生成论文摘要