Strength prediction of steel CHS X-joints via leveraging finite element method and machine learning solutions
Journal of Constructional Steel Research(2021)
摘要
This study employs high-fidelity finite element method (FEM) and machine learning (ML) solutions to determine the accurate strengths of steel circular hollow section (CHS) X-joints with minimal computational effort. FE analysis methods have helped to improve the understanding of the complex structural behavior of CHS joints. However, the routine use of FE analysis imposes high computational demands and requires a thorough verification of numerical models. Alternatively, this study explores the feasibility of using ML methods for estimating the strengths of CHS X-joints. High-fidelity FE models were first built to generate about 4000 datasets. Each dataset included seven joint parameters, namely, steel grade, chord diameter, brace-to-chord diameter ratio, chord diameter-to-thickness ratio, brace-to-chord thickness ratio, chord length-to-radius ratio, and chord stress level. Two ML models; a support vector regression (SVR) and a deep neural network regression (DNNR) were formulated, extensively trained, tested, and validated via comparison with experimental data. The validated DNNR model provided a more accurate prediction of the joint strength with an average error of 0.8%. On the basis of extensive joint strength data readily available from the proposed ML solution, the joint strength margin implied in current design equations was evaluated from various perspectives via variation of key joint parameters.
更多查看译文
关键词
Circular hollow section (CHS),X-joint,Deep neural network,Machine learning,Support vector regression
AI 理解论文
溯源树
样例
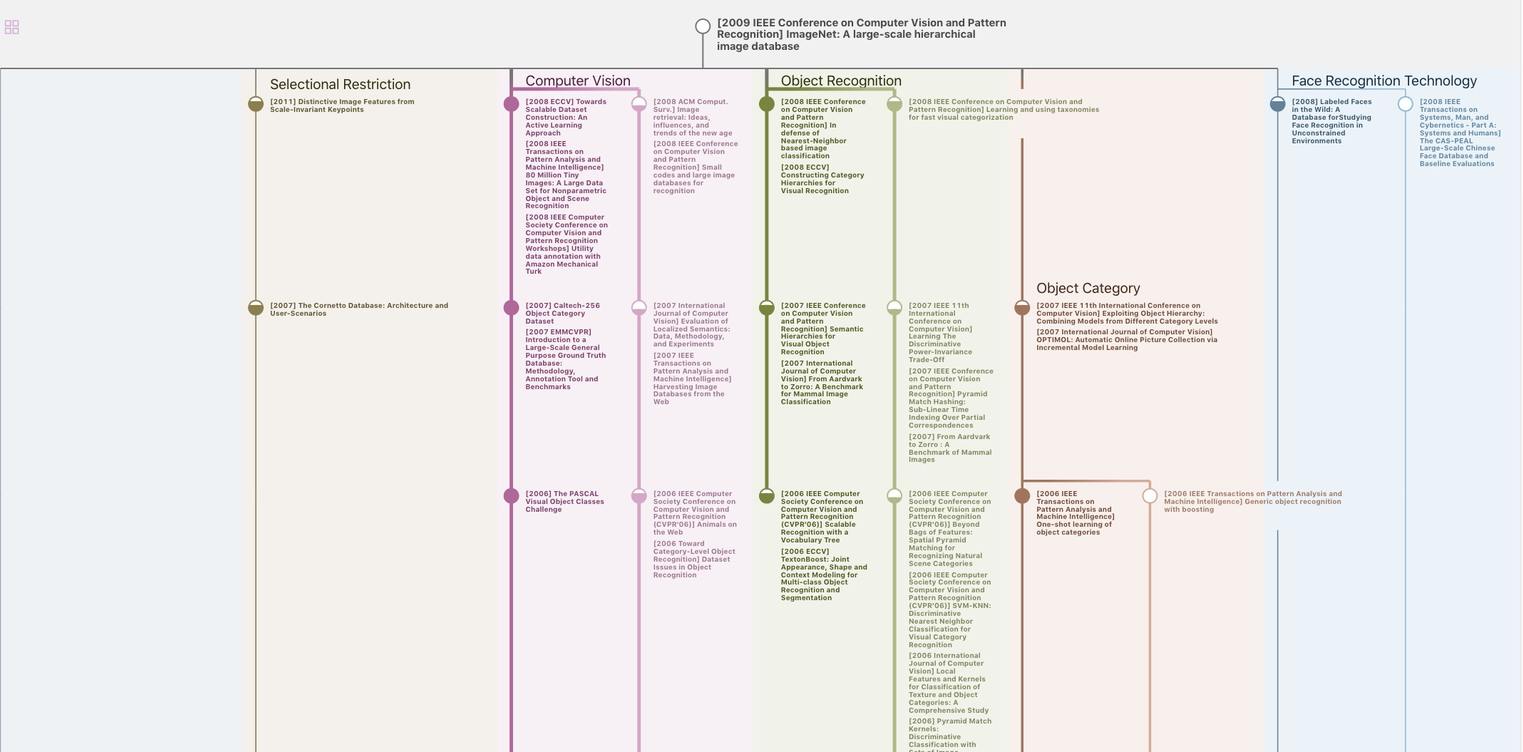
生成溯源树,研究论文发展脉络
Chat Paper
正在生成论文摘要