Bayesian inference in based-kernel regression: comparison of count data of condition factor of fish in pond systems
JOURNAL OF APPLIED STATISTICS(2022)
摘要
The discrete kernel-based regression approach generally provides pointwise estimates of count data that do not account for uncertainty about both parameters and resulting estimates. This work aims to provide probabilistic kernel estimates of count regression function by using Bayesian approach and then allows for a readily quantification of uncertainty. Bayesian approach enables to incorporate prior knowledge of parameters used in discrete kernel-based regression. An application was proposed on count data of condition factor of fish (K) provided from an experimental project that analyzed various pond management strategies. The probabilistic distribution of estimates were contrasted by discrete kernels, as a support to theoretical results on the performance of kernels. More practically, Bayesian credibility intervals ofK-estimates were evaluated to compare pond management strategies. Thus, similarities were found between performances of semi-intensive and coupled fishponds, with formulated feed, in comparison with extensive fishponds, without formulated feed. In particular, the fish development was less predictable in extensive fishpond, dependent on natural resources, than in the two other fishponds, supplied in formulated feed.
更多查看译文
关键词
Aquaculture, discrete kernel, fishpond, prior and posterior distributions, uncertainty analysis
AI 理解论文
溯源树
样例
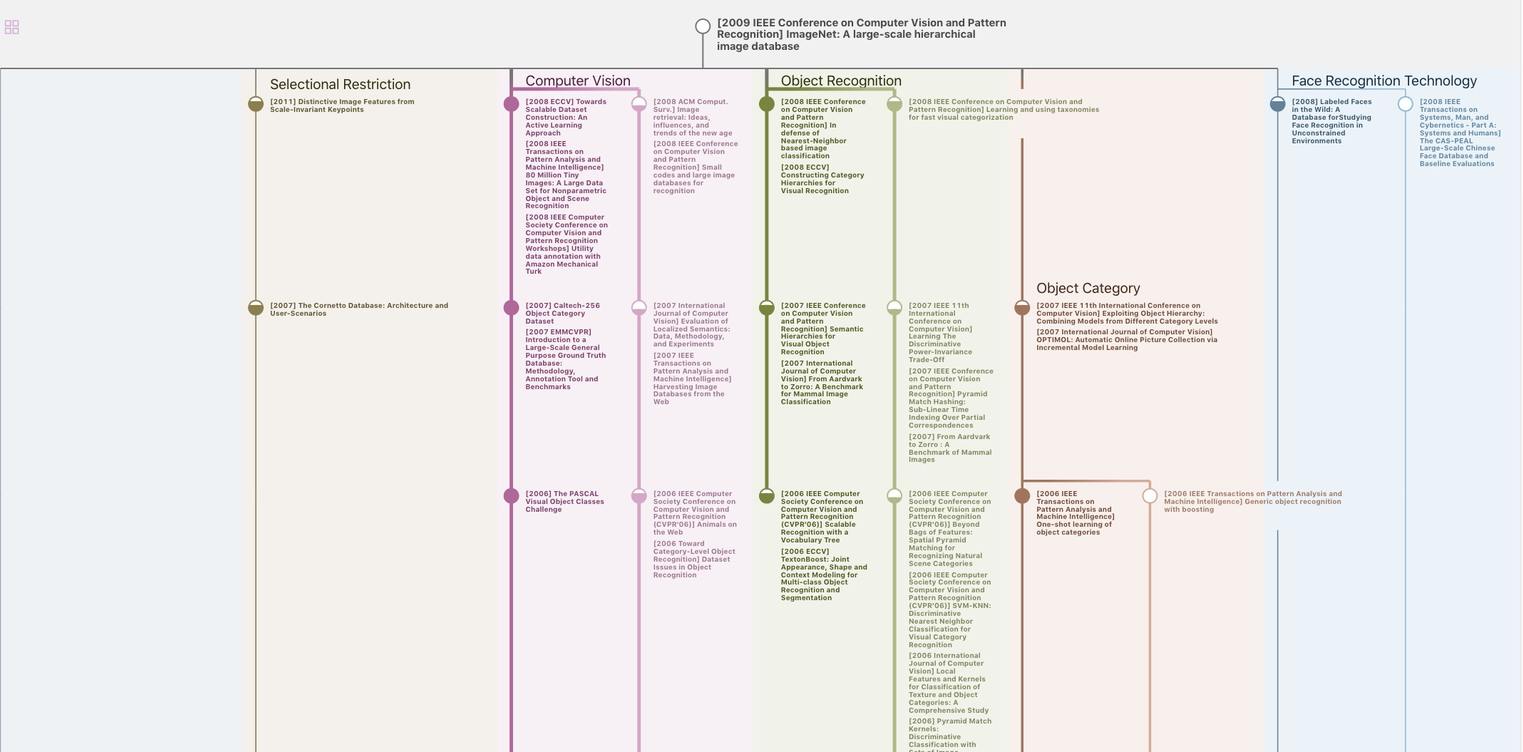
生成溯源树,研究论文发展脉络
Chat Paper
正在生成论文摘要