Semi-Supervised Learning-Based Calibration Model Building Of Nir Spectroscopy For In Situ Measurement Of Biochemical Processes Under Insufficiently And Inaccurately Labeled Samples
IEEE TRANSACTIONS ON INSTRUMENTATION AND MEASUREMENT(2021)
摘要
To deal with the typical problem of inaccurately and insufficiently labeled samples involved with real measurement of biochemical process components, a semi-supervised learning-based calibration model building method is proposed to use the up-to-date near-infrared (NIR) spectroscopy for in situ measurement of such a process. First, a supervised ladder network is designed to delete the inaccurately labeled samples, such that a cleaned training set is used for establishing the NIR calibration model. To address the issue of insufficiently labeled samples for modeling with the aim of online measurement, a semi-supervised ladder network is explored to establish a regression learning algorithm, by representing the process characteristics from all the unlabeled samples in combination with the cleaned labeled samples. In the ladder network, encoding is used to extract the features and decoding is used for denoising. Meanwhile, the skip connections allow higher layers to concentrate on those features related to the regression task, therefore facilitating the model accuracy. A numerical example and experiments on a glucose fermentation process are shown to demonstrate the effectiveness and advantage of the proposed method.
更多查看译文
关键词
Calibration model building, inaccurate samples, in situ measurement, near-infrared (NIR) spectroscopy, semi-supervised learning (SSL)
AI 理解论文
溯源树
样例
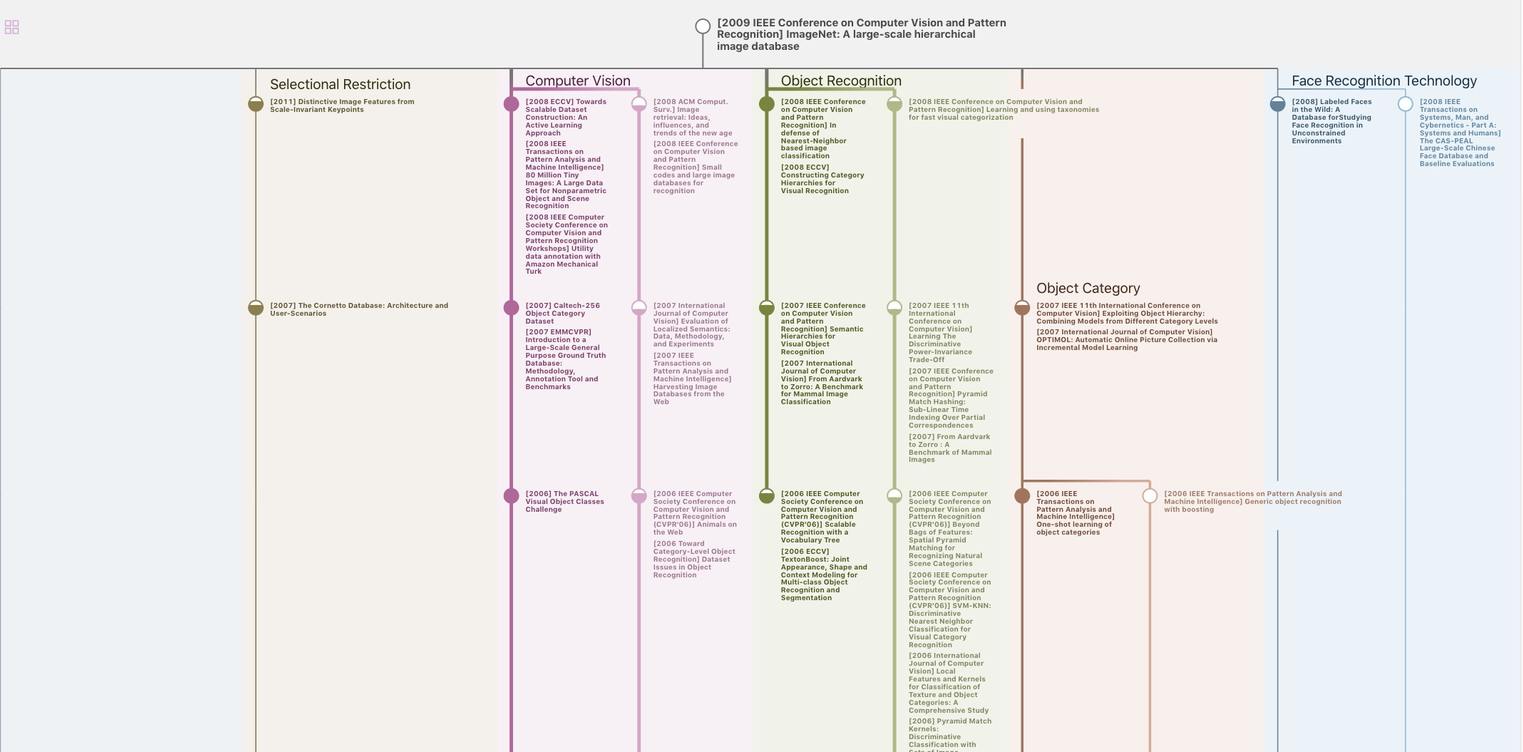
生成溯源树,研究论文发展脉络
Chat Paper
正在生成论文摘要