Infinite mixture models for operational modal analysis: An automated and principled approach
Journal of Sound and Vibration(2021)
摘要
The development of a fully automated system identifier without the need for human intervention, is a key step for real-time vibration-based Structural Health Monitoring (SHM). In this paper a novel approach based on the Dirichlet Process Gaussian Mixture Model (DP-GMM) is developed in order to analyze the stabilization diagram. The aim is to separate the true physical modes from the mathematically spurious modes in a fully automated manner, whilst eliminating the need for any manually specified parameters or thresholds. The parametric Covariance Driven Stochastic Subspace Identification (SSI-Cov) is adopted to estimate the modal parameters, and consequently establish the initial stabilization diagram. From there, the use of a two-stage algorithm involving a DP-GMM is proposed to non-parametrically perform an automated cleaning of the stabilization diagram. The contributions of the paper are five-fold: (1) A probabilistic approach based on a DP-GMM is proposed to analyze the stabilization diagram. To the best knowledge of the authors, this study presents one of the first attempts of DP-GMM for full automation of Operational Modal Analysis (OMA). The method is validated using the field test data from a large-scale operating cable-stayed bridge, which has two closely-spaced modes around 3 Hz. Not only are these two complicated scenarios consistently identified, but the performance of the method with respect to the problem of missing modes is compared against a reference method based on the conventional multi-stage clustering technique used in OMA, wherein superior performance of the proposed method is demonstrated. (2) The method does not require specification of any threshold or parameter at any stage of the algorithm for cleaning the stabilization diagram, making the approach a potential for robust and fully automated modal identification. (3) Compared to many conventional multi-stage clustering techniques, the proposed approach is computationally efficient as intelligent updates are made to the model using multiple linear algebra properties. (4) New feature vectors are developed which are justified using a combination of mathematical rigor, visual understanding, and engineering intuition. (5) Due to the probabilistic nature of the method, the identification results are accompanied with uncertainty bounds. Several mathematical proofs are presented to explain the observed behavior of the uncertainty bounds.
更多查看译文
关键词
Ambient vibration,Operational modal analysis,Stabilization diagram,Stochastic subspace identification,Dirichlet process,Bayesian non-parametric
AI 理解论文
溯源树
样例
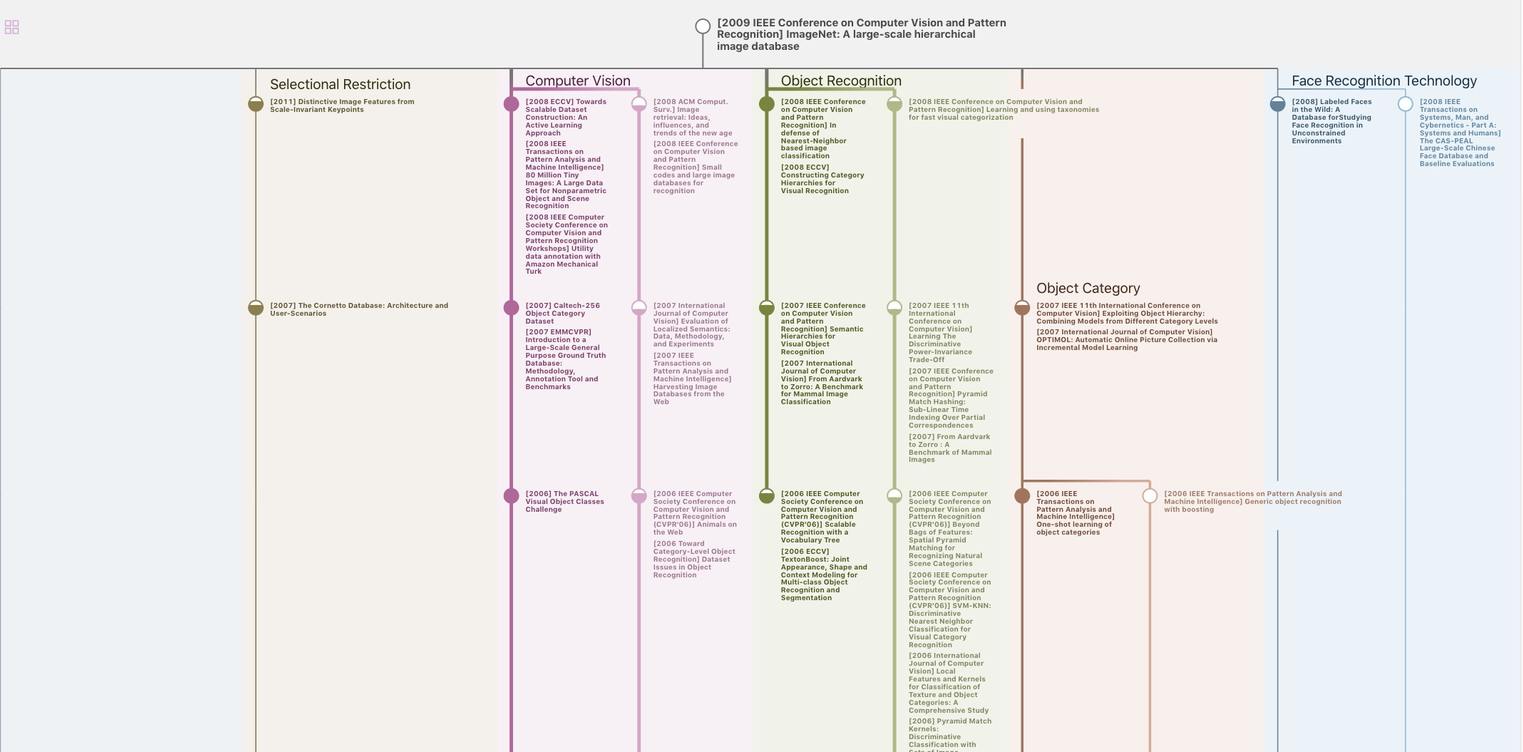
生成溯源树,研究论文发展脉络
Chat Paper
正在生成论文摘要