Dual-Level Selective Transfer Learning for Intrahepatic Cholangiocarcinoma Segmentation in Non-enhanced Abdominal CT.
medical image computing and computer-assisted intervention(2020)
摘要
Automatic and accurate Intrahepatic Cholangiocarcinoma (ICC) segmentation in non-enhanced abdominal CT images can provide significant assistance for clinical decision making. While deep neural networks offer an effective tool for ICC segmentation, collecting large amounts of annotated data for deep network training may not be practical for this kind of applications. To this end, transfer learning approaches utilize abundant data from similar tasks and transfer the prior-learned knowledge to achieve better results. In this paper, we propose a novel Dual-level Selective Transfer Learning (DSTL) model for ICC segmentation, which selects similar information at global and local levels from a source dataset and produces transfer learning using the selected hierarchical information. Besides the basic segmentation networks, our DSTL model is composed of a global information selection network (GISNet) and a local information selection network (LISNet). The GISNet is utilized to output weights for global information selection and to mitigate the gap between the source and target tasks. The LISNet outputs weights for local information selection. Experimental results show that our DSTL model achieves superior ICC segmentation performance and outperforms the original and image selection based transfer learning and joint training strategies. To the best of our knowledge, this is the first method for ICC segmentation in non-enhanced abdominal CT.
更多查看译文
关键词
Tumor segmentation, Intrahepatic Cholangiocarcinoma, Selective transfer learning
AI 理解论文
溯源树
样例
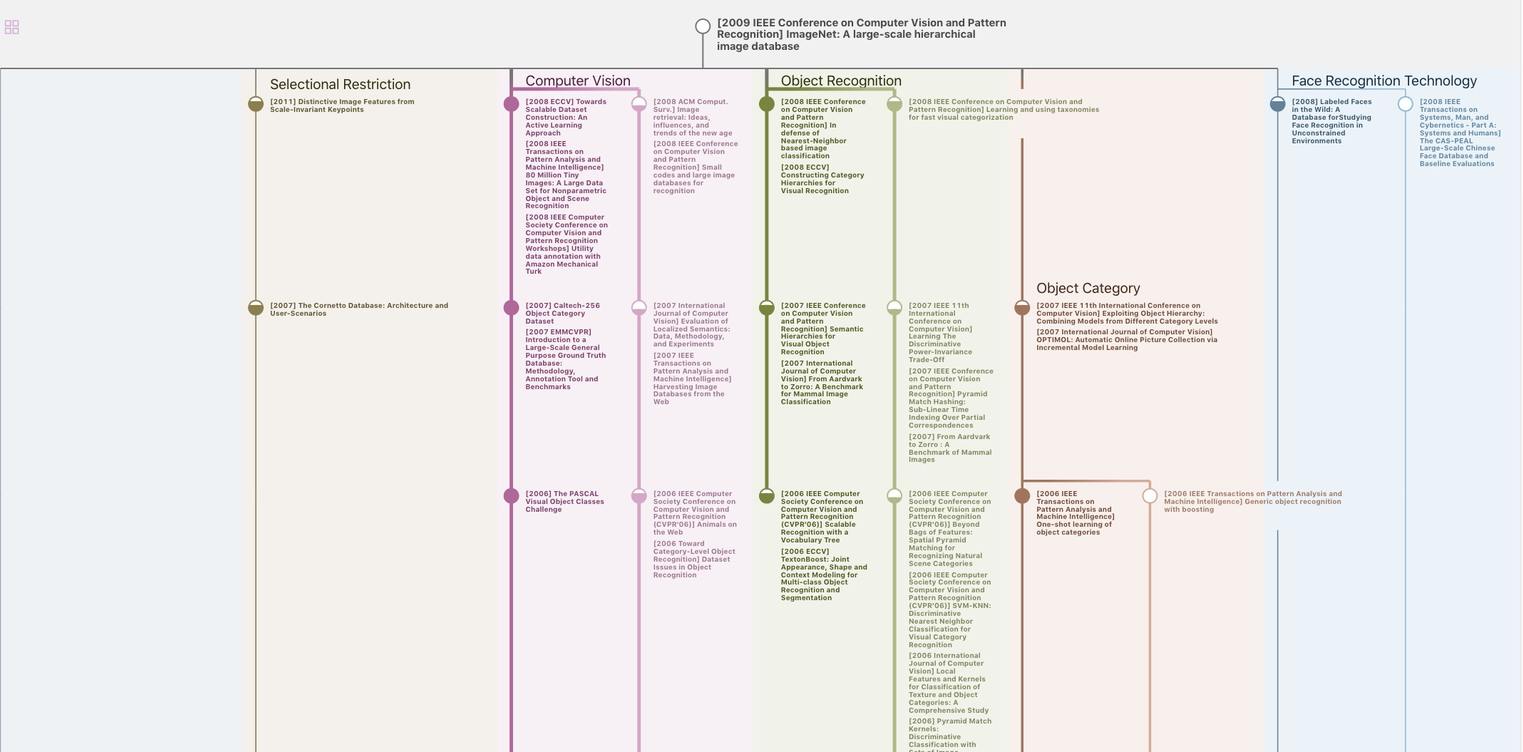
生成溯源树,研究论文发展脉络
Chat Paper
正在生成论文摘要