A Concentration-Based Drift Calibration Transfer Learning Method for Gas Sensor Array Data
IEEE Sensors Letters(2020)
摘要
The practical use of electronic nose (e-nose) systems suffers from drift issue, which alters data distribution and reduces the accuracy of classification. This letter proposes a transfer learning method called concentration-based drift calibration (CDC) for calibrating the sensor drift. Based on the sensor characteristic that sensor response is correspondent to gas concentration, transfer samples were collected in the target domain for certain gas concentrations and then used for calibration with a designed concentration-based model and CDC transfer process. This method was evaluated on a complex time-varying drift dataset. The experimental results show that the proposed method for drift calibration is effective and can be used for real-world applications. Moreover, the CDC transfer process can be applied over time with data that has been previously collected to yield a more generalized model.
更多查看译文
关键词
Sensor signal processing,calibration,drift,electronic nose (e-nose),transfer learning
AI 理解论文
溯源树
样例
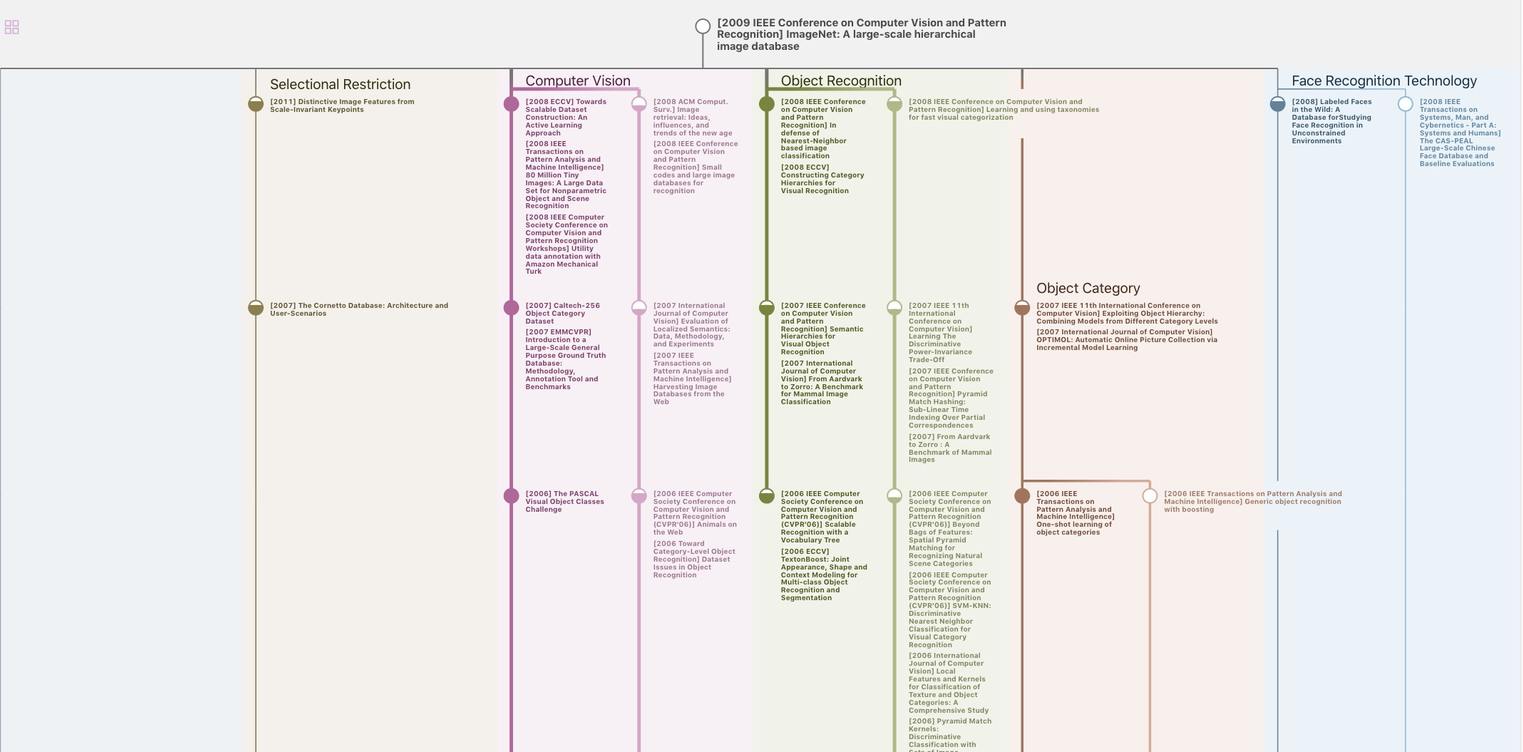
生成溯源树,研究论文发展脉络
Chat Paper
正在生成论文摘要