Fuzzy Logic-Driven Variable Time-Scale Prediction-Based Reinforcement Learning for Robotic Multiple Peg-in-Hole Assembly
IEEE Transactions on Automation Science and Engineering(2022)
摘要
Reinforcement learning (RL) has been increasingly used for single peg-in-hole assembly, where assembly skill is learned through interaction with the assembly environment in a manner similar to skills employed by human beings. However, the existing RL algorithms are difficult to apply to the multiple peg-in-hole assembly because the much more complicated assembly environment requires sufficient exploration, resulting in a long training time and less data efficiency. To this end, this article focuses on how to predict the assembly environment and how to use the predicted environment in assembly action control to improve the data efficiency of the RL algorithm. Specifically, first, the assembly environment is exactly predicted by a variable time-scale prediction (VTSP) defined as general value functions (GVFs), reducing the unnecessary exploration. Second, we propose a fuzzy logic-driven variable time-scale prediction-based reinforcement learning (FLDVTSP-RL) for assembly action control to improve the efficiency of the RL algorithm, in which the predicted environment is mapped to the impedance parameter in the proposed impedance action space by a fuzzy logic system (FLS) as the action baseline. To demonstrate the effectiveness of VTSP and the data efficiency of the FLDVTSP-RL methods, a dual peg-in-hole assembly experiment is set up; the results show that FLDVTSP-deep Q-learning (DQN) decreases the assembly time about 44% compared with DQN and FLDVTSP-deep deterministic policy gradient (DDPG) decreases the assembly time about 24% compared with DDPG.
Note to Practitioners
—The complicated assembly environment of the multiple peg-in-hole assembly results in a contact state that cannot be recognized exactly from the force sensor. Therefore, contact-model-based methods that require tuning of the control parameters based on the contact state recognition cannot be applied directly in this complicated environment. Recently, reinforcement learning (RL) methods without contact state recognition have recently attracted scientific interest. However, the existing RL methods still rely on numerous explorations and a long training time, which cannot be directly applied to real-world tasks. This article takes inspiration from the manner in which human beings can learn assembly skills with a few trials, which relies on the variable time-scale predictions (VTSPs) of the environment and the optimized assembly action control strategy. Our proposed fuzzy logic-driven variable time-scale prediction-based reinforcement learning (FLDVTSP-RL) can be implemented in two steps. First, the assembly environment is predicted by the VTSP defined as general value functions (GVFs). Second, assembly action control is realized in an impedance action space with a baseline defined by the impedance parameter mapped from the predicted environment by the fuzzy logic system (FLS). Finally, a dual peg-in-hole assembly experiment is conducted; compared with deep Q-learning (DQN), FLDVTSP-DQN can decrease the assembly time about 44%; compared with deep deterministic policy gradient (DDPG), FLDVTSP-DDPG can decrease the assembly time about 24%.
更多查看译文
关键词
Fuzzy logic system (FLS),multiple peg-in-hole,prediction learning,reinforcement learning (RL),robotic assembly
AI 理解论文
溯源树
样例
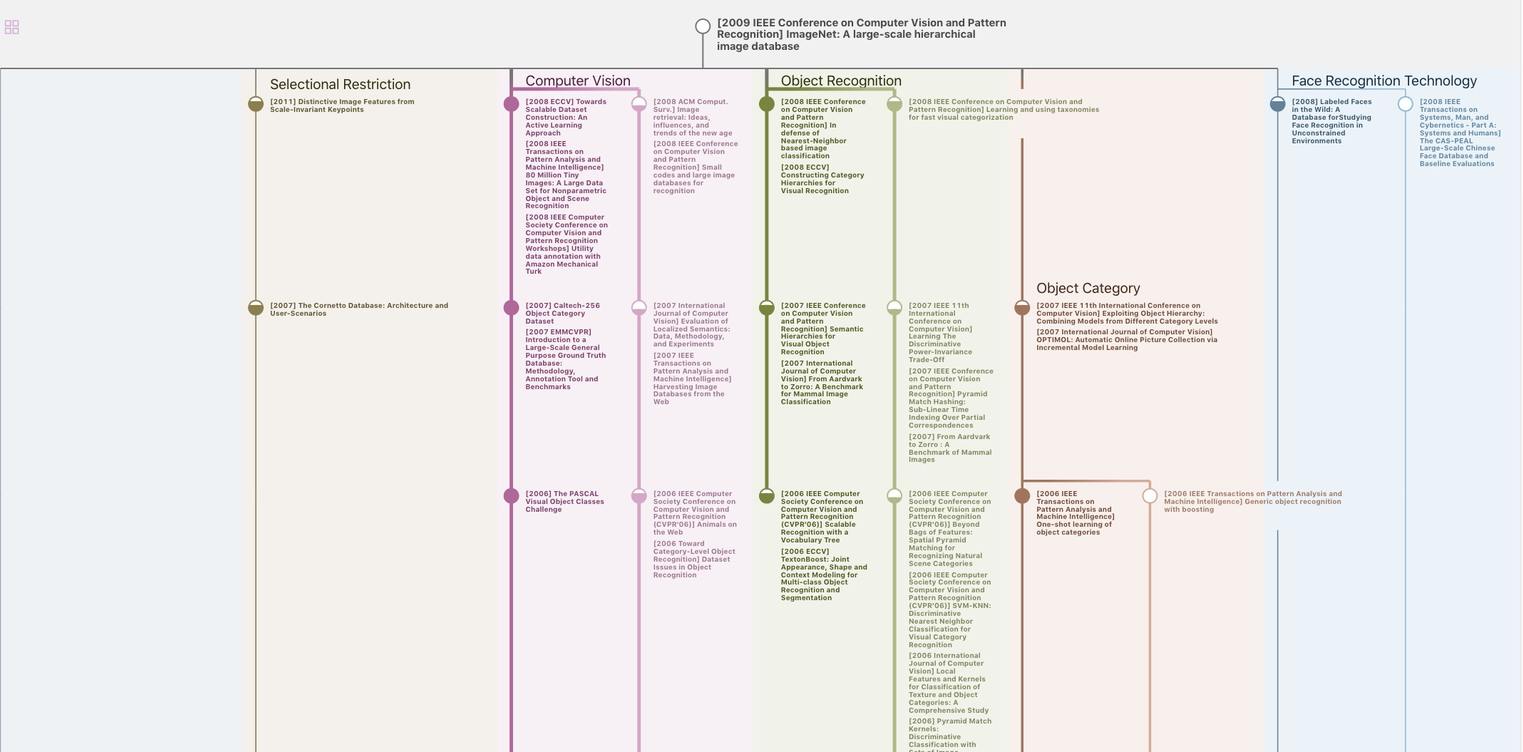
生成溯源树,研究论文发展脉络
Chat Paper
正在生成论文摘要