What Do Linguistic Expressions Tell Us about Learners’ Confusion? A Domain-Independent Analysis in MOOCs
IEEE Transactions on Learning Technologies(2020)
摘要
The substantial growth of online learning, and in particular, through massively open online courses (MOOCs), supports research into nontraditional learning contexts. Learners' confusion is one of the identified aspects which impact the overall learning process, and ultimately, course attrition. Confusion for a learner is an individual state of bewilderment and uncertainty as to how to move forward. The majority of recent works in MOOCs neglects the individual factor and measure the influence of community-related aspects (e.g., votes, views) for confusion classification. While these are useful measures, these models neglect the personalized context, such as an individual's affect or emotions. Toward this, and within the MOOC context, we propose machine learning models based solely on language and discourse features extracted from learners' discussion posts. With over 83% F1-score in all domains, it is evident that linguistic-only features are highly effective in confusion classification, with our models outperforming previous models developed for confusion classification. In this article, we also obtain good performance for cross-domain confusion classification between 70.7% and 84.5% F1-score for all domain pairs (i.e., training on one domain and testing on another domain). Primarily, this article contributes through the development of a novel linguistic feature set that is predictive for effective confusion classification.
更多查看译文
关键词
Confusion,discourse,linguistics,machine learning,massively open online course (MOOC),natural language processing.
AI 理解论文
溯源树
样例
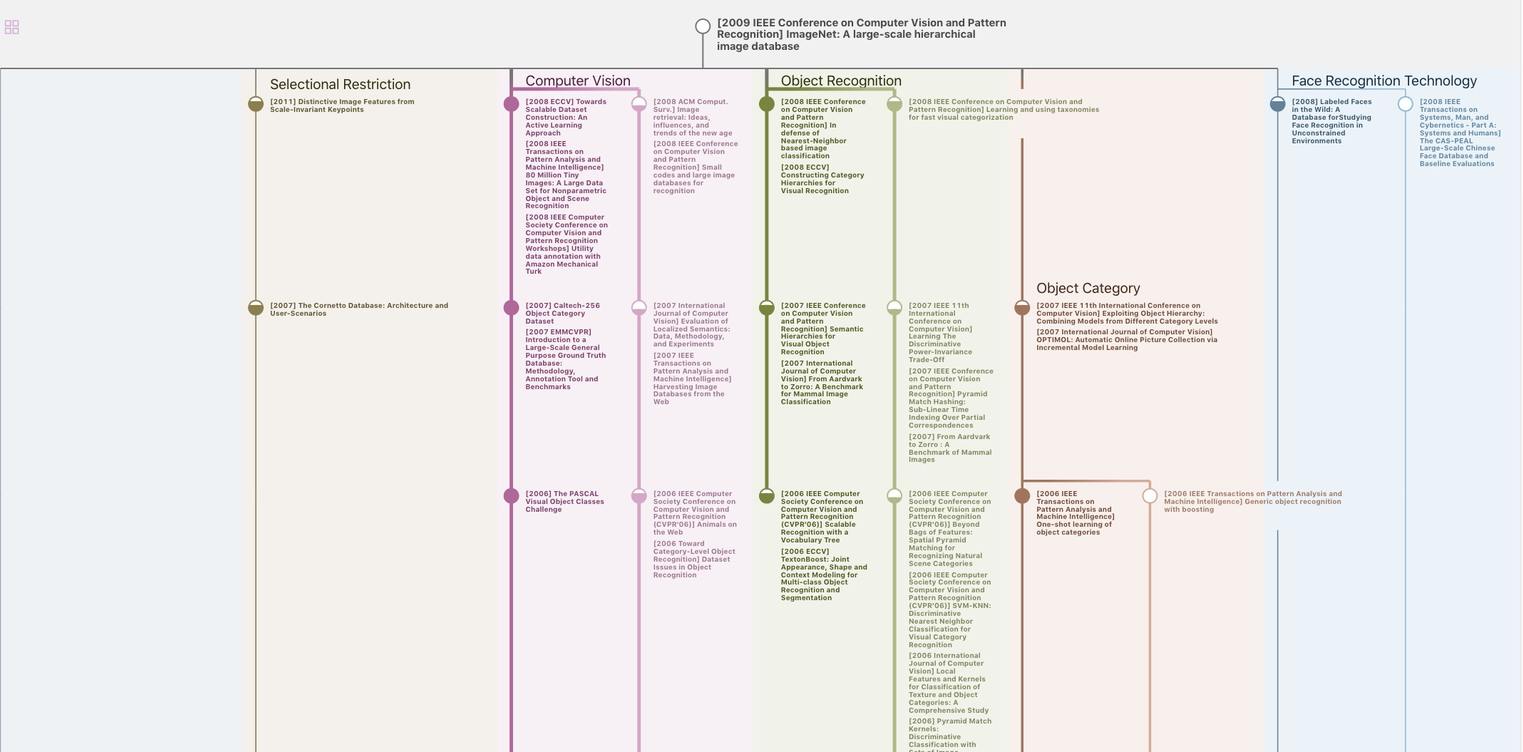
生成溯源树,研究论文发展脉络
Chat Paper
正在生成论文摘要