Fanet: Features Adaptation Network For 360 Degrees Omnidirectional Salient Object Detection
IEEE SIGNAL PROCESSING LETTERS(2020)
摘要
Salient object detection (SOD) in 360 degrees omnidirectional images has become an eye-catching problem because of the popularity of affordable 360 degrees cameras. In this paper, we propose a Features Adaptation Network (FANet) to highlight salient objects in 360 degrees omnidirectional images reliably. To utilize the feature extraction capability of convolutional neural networks and capture global object information, we input the equirectangular 360 degrees images and corresponding cube-map 360 degrees images to the feature extraction network (FENet) simultaneously to obtain multi-level equirectangular and cube-map features. Furthermore, we fuse these two kinds of features at each level of the FENet by a projection features adaptation (PFA) module, for selecting these two kinds of features adaptively. Finally, we combine the preliminary adaptation features at different levels by a multi-level features adaptation (MLFA) module, which weights these different-level features adaptively and produces the final saliencymaps. Experiments show our FANet outperforms the state-of-the-art methods on the 360 degrees omnidirectional SOD datasets.
更多查看译文
关键词
360 degrees omnidirectional image, salient object detection, equirectangular and cube-map projection, projection features adaptation, multi-level features adaptation
AI 理解论文
溯源树
样例
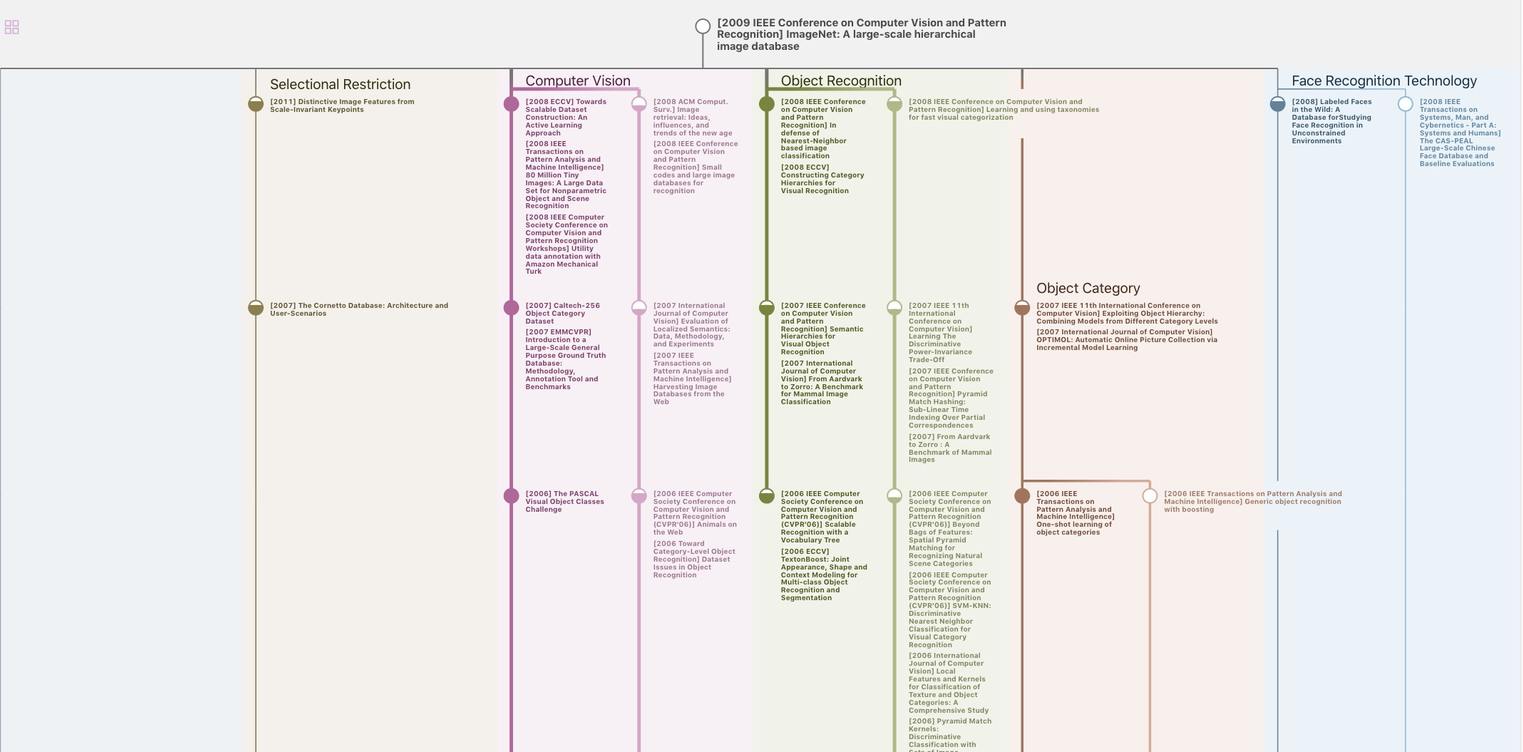
生成溯源树,研究论文发展脉络
Chat Paper
正在生成论文摘要