Identification Of Driver Braking Intention Based On Long Short-Term Memory (Lstm) Network
IEEE ACCESS(2020)
摘要
Driving intention identification is a key technology which can improve the adaptability of the intelligent driver assistance systems and the energy efficiency of electric vehicles. This article proposes a novel method for identifying the driver braking intention. In order to improve the identification accuracy of driving intention, a braking intention identification model based on Long Short-Term Memory (LSTM) Network is constructed. The data of slight braking, normal braking and hard braking that can use for offline training are obtained through tests on real vehicle at Chang'an University vehicle performance testing ground. Support vector machine - recursive feature elimination (SVM-RFE) algorithm is used to select the characteristic parameter of braking intention identification model. The random search is subsequently used to optimize the hyper-parameters of LSTM. LSTM-based and Gaussian Hidden Markov Model (GHMM)-based model under different time window are used to identify braking intention of slight braking, normal braking and hard braking respectively. The results show that the Precision, Recall, F-measure, Accuracy of the braking intention identification model which propose in this paper based on LSTM are better than that of the braking intention identification model based on GHMM. Moreover, the Recall and Accuracy of the LSTM-based braking intention identification models are above 0.95, indicating the good ability of intention identification.
更多查看译文
关键词
Hidden Markov models, Brakes, Vehicles, Support vector machines, Force, Feature extraction, Data models, Braking intention recognition, driving safety, driver assistance system, LSTM network, accuracy and real-time
AI 理解论文
溯源树
样例
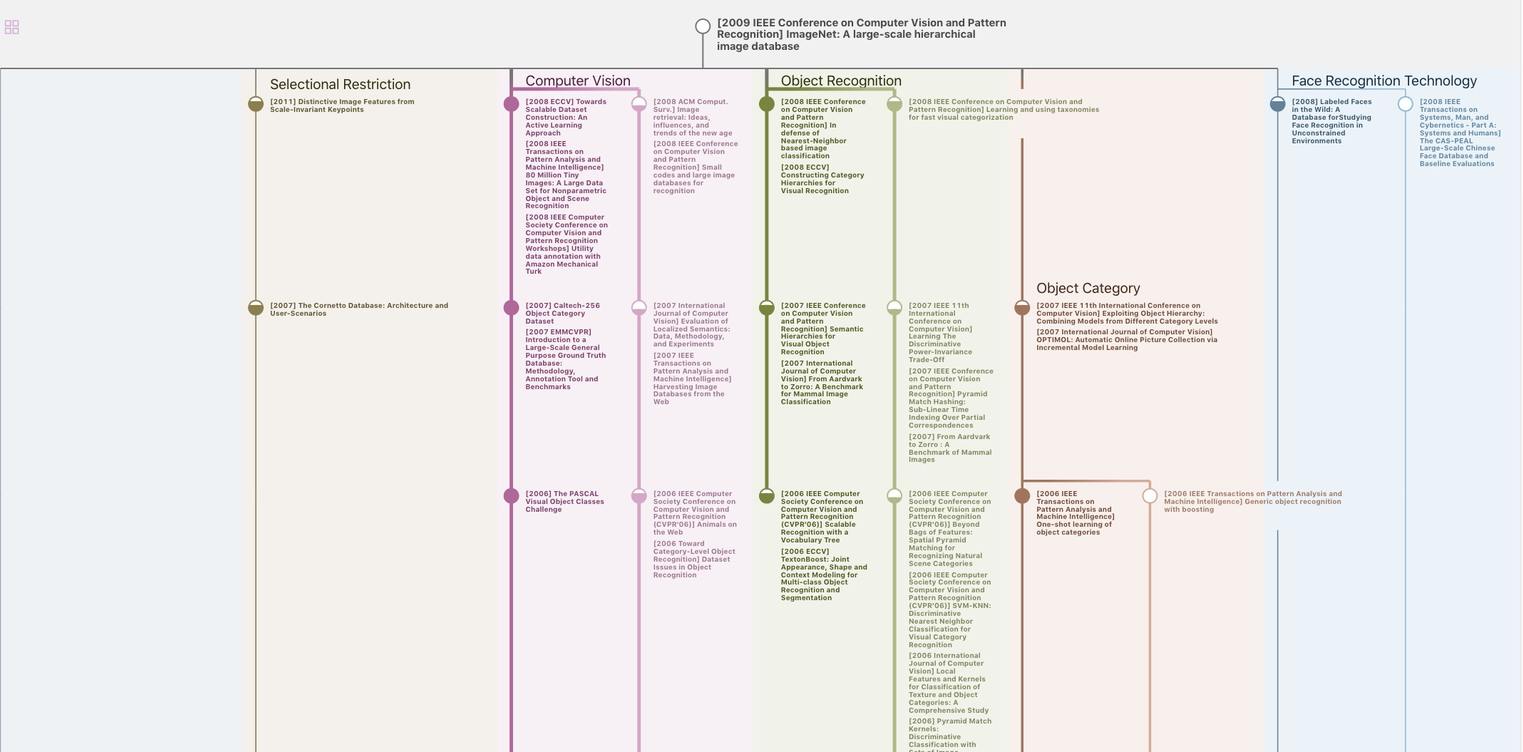
生成溯源树,研究论文发展脉络
Chat Paper
正在生成论文摘要