An FPGA-Based Hybrid Neural Network Accelerator for Embedded Satellite Image Classification
ISCAS(2020)
摘要
Spiking Neural Networks are well known for their hardware-friendliness, as their implementation enables important hardware resources and energy savings when compared to classical Neural Network accelerators. Those advantages are mostly due to their simple Integrate & Fire neuron model, and the event-based aspect of their computation. On the other hand, convolution layers and maxpooling layers enable robust feature extraction, thus yielding very high recognition accuracy in image classification applications. Unfortunately, those layer models are not well-suited to the spiking model when dealing with static image classification on FPGA. Based on this statement, we developed an innovative hybrid Neural Network embedded accelerator. Our architecture interfaces classical non-spiking convolution Neural network for feature extraction; and spiking Dense Layers for classification. The implementation showed recognition performances (87%) equivalent to its classical counterpart (88%), while reducing hardware resource intensiveness of the classification stage (12% less occupied logic cells in total, including 60% reduction on the classification stage).
更多查看译文
关键词
Spiking Neural Networks,FPGA,Machine Learning,Image Classification,Satellite,Embedded Systems
AI 理解论文
溯源树
样例
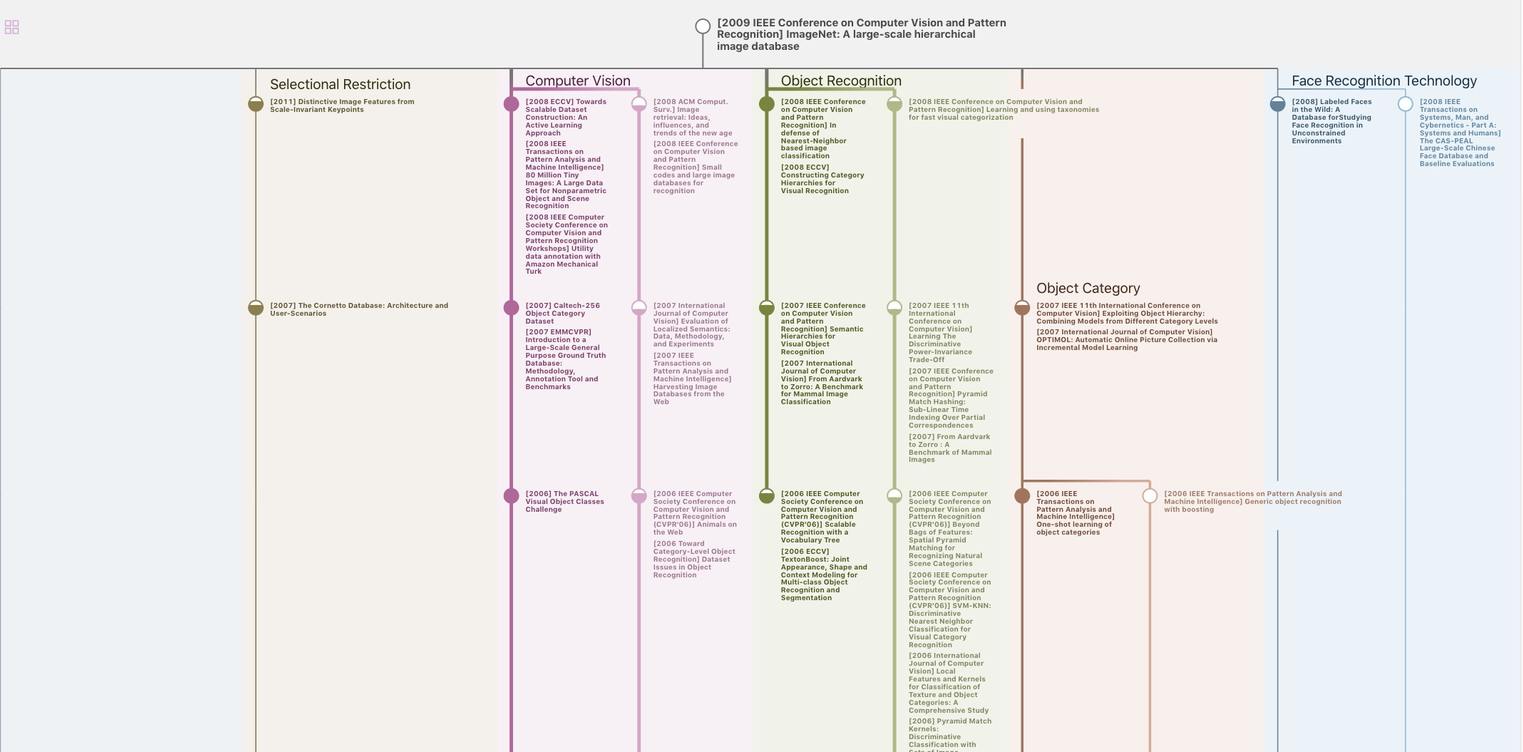
生成溯源树,研究论文发展脉络
Chat Paper
正在生成论文摘要