Automatic Tooth Segmentation and Dense Correspondence of 3D Dental Model.
medical image computing and computer-assisted intervention(2020)
摘要
In this paper, we propose an end-to-end coupled 3D tooth segmentation and dense correspondence network (c-SCN) for annotation of individual teeth and gingiva of clinically-obtained 3D dental models. The proposed model can be stacked on an existing graph convolutional network (GCN) for feature extraction from dental meshes. We devise a branch network for the instance-aware geodesic maps with respect to virtual tooth crown centroids for feature enhancement. The geodesic map encodes the spatial relationship of an individual tooth with the remaining dental model, and is concatenated with the GCN-based vertex-wise feature fields for simultaneous tooth segmentation and labeling. Furthermore, the label probability matrix from the multi-category classifier, indicating individual tooth regions and boundaries, is used to enhance the inference of dense correspondence. By utilizing the smooth semantic correspondence with the preservation of geometric topology, our approach addresses the attribute transfer-based landmark location. The qualitative and quantitative evaluations on the clinically-obtained dental models of orthodontic patients demonstrate that our approach achieves effective tooth annotation and dense correspondence, outperforming the compared state-of-the-art.
更多查看译文
关键词
Tooth annotation, Dense correspondence, Instance-aware geodesic map
AI 理解论文
溯源树
样例
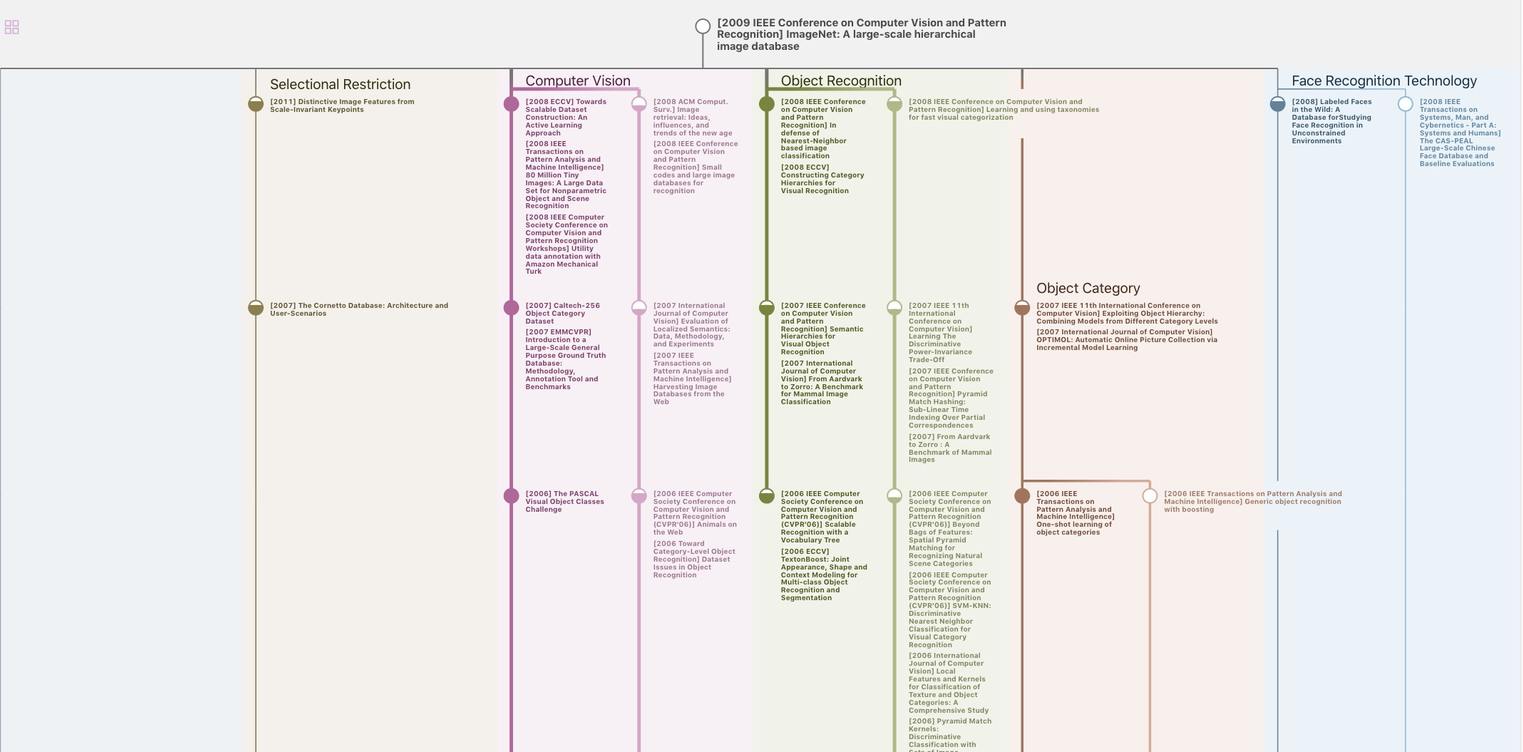
生成溯源树,研究论文发展脉络
Chat Paper
正在生成论文摘要