RL Based Network Accelerator Compiler for Joint Compression Hyper-Parameter Search
ISCAS(2020)
摘要
Although compression techniques like pruning or quantization are beneficial for accelerators' energy efficiency, the large search space makes finding the appropriate compression scheme difficult. Besides, most existing works ignore the combination of both pruning and quantization. In this paper, we propose a reinforcement learning (RL) based joint compression framework to find the appropriate pruning ratio and quantization bit-width for accelerators. By interacting with the energy model of the target accelerator, the RL agent can learn the effect of compression scheme on both accuracy and energy efficiency. Through a long trial-and-error process, the agent can finally reach an optimal trade-off between accuracy and energy efficiency. Compared with control groups whose compression hyper-parameters are not jointly optimized, the proposed framework can achieve at least 25% energy reduction with higher accuracy or much higher accuracy with small disadvantages on energy. Compared with 8-bit quantized baseline, the framework can achieve 90% and 85% energy reduction on Cifar10 and Cifar100 respectively.
更多查看译文
关键词
network accelerator,reinforcement learning,joint compression,hyper-parameter exploration
AI 理解论文
溯源树
样例
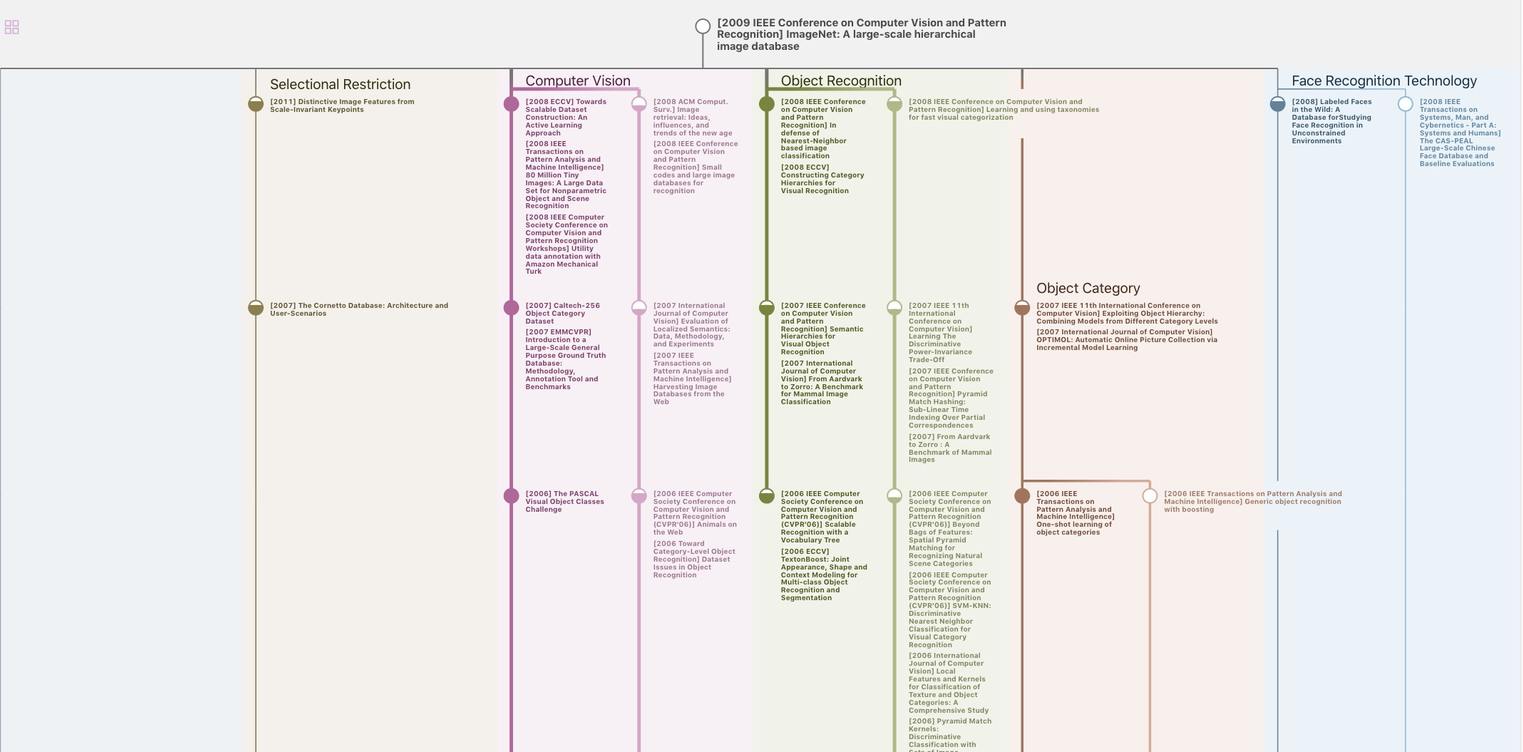
生成溯源树,研究论文发展脉络
Chat Paper
正在生成论文摘要