Improved Resection Margins in Surgical Oncology Using Intraoperative Mass Spectrometry
medical image computing and computer assisted intervention(2020)
摘要
PURPOSE: Incomplete tumor resections leads to the presence of cancer cells on the resection margins demanding subsequent revision surgery and poor outcomes for patients. Intraoperative evaluations of the tissue pathology, including the surgical margins, can help decrease the burden of repeat surgeries on the patients and healthcare systems. In this study, we propose adapting multi instance learning (MIL) for prospective and intraoperative basal cell carcinoma (BCC) detection in surgical margins using mass spectrometry. METHODS: Resected specimens were collected and inspected by a pathologist and burnt with iKnife. Retrospective training data was collected with a standard cautery tip and included 63 BCC and 127 normal burns. Prospective data was collected for testing with both the standard and a fine tip cautery. This included 130 (66 BCC and 64 normal) and 99 (32 BCC and 67 normal) burns, respectively. An attention-based MIL model was adapted and applied to this dataset. RESULTS: Our models were able to predict BCC at surgical margins with AUC as high as 91%. The models were robust to changes in cautery tip but their performance decreased slightly. The models were also tested intraoperatively and achieved an accuracy of 94%. CONCLUSION: This is the first study that applies the concept of MIL for tissue characterization in perioperative and intraoperative REIMS data.
更多查看译文
关键词
Surgical margin detection, Multiple instance learning, Rapid evaporative ionization mass spectrometry, Intraoperative tissue characterization, Non-linear analysis, Basal Cell Carcinoma
AI 理解论文
溯源树
样例
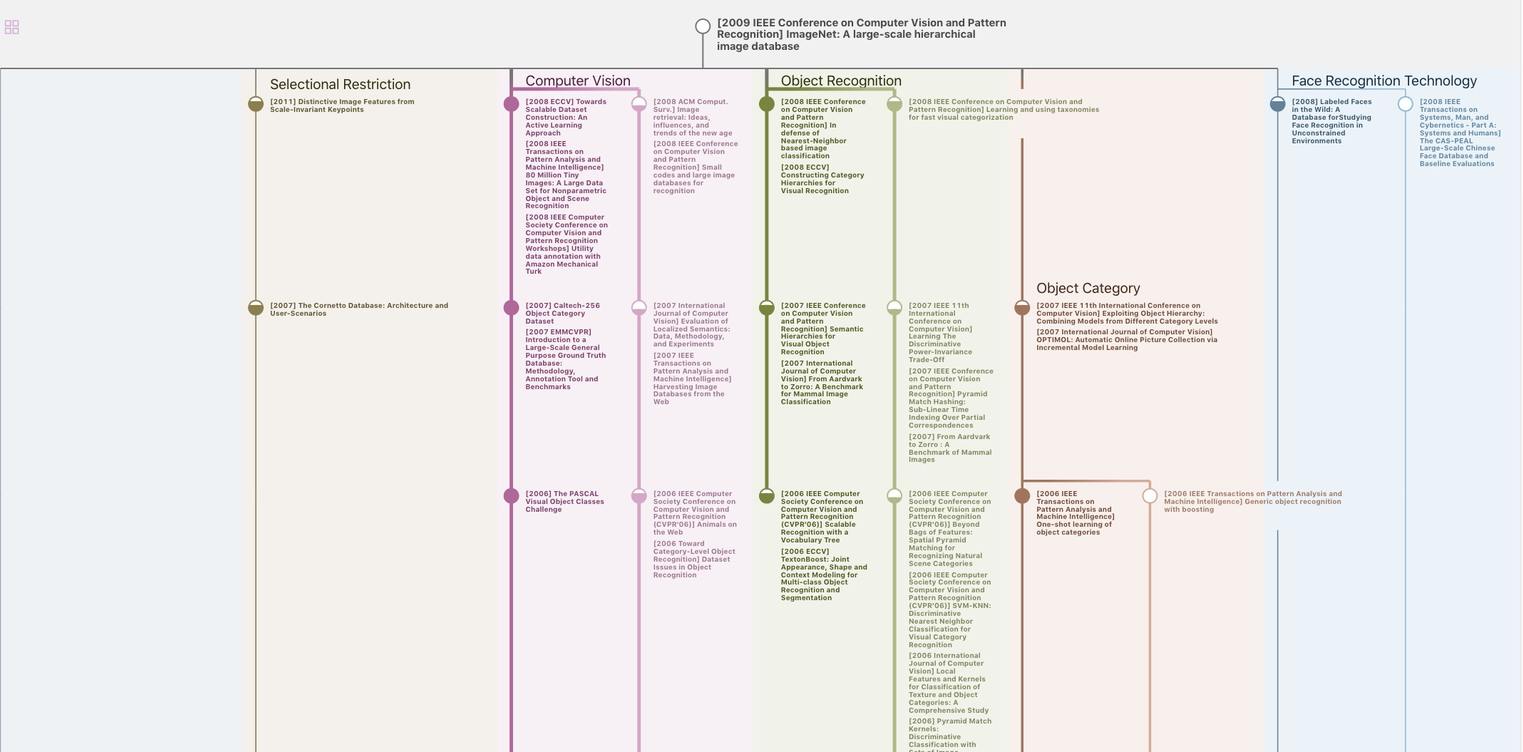
生成溯源树,研究论文发展脉络
Chat Paper
正在生成论文摘要