Addressing the Conditional and Correlated Wind Power Forecast Errors in Unit Commitment by Distributionally Robust Optimization
IEEE Transactions on Sustainable Energy(2021)
摘要
In this paper, a study of the day-ahead unit commitment problem with stochastic wind power generation is presented, which considers conditional, and correlated wind power forecast errors through a distributionally robust optimization approach. Firstly, to capture the characteristics of random wind power forecast errors, the least absolute shrinkage, and selection operator (Lasso) is utilized to develop a robust conditional error estimator, while an unbiased estimator is used to obtain the covariance matrix. The conditional error, and the covariance matrix are then used to construct an enhanced ambiguity set. Secondly, we develop an equivalent mixed integer semidefinite programming (MISDP) formulation of the two-stage distributionally robust unit commitment model with a polyhedral support of random variables. Further, to efficiently solve this problem, a novel cutting plane algorithm that makes use of the extremal distributions identified from the second-stage semidefinite programming (SDP) problems is introduced. Finally, numerical case studies show the advantage of the proposed model in capturing the spatiotemporal correlation in wind power generation, as well as the economic efficiency, and robustness of dispatch decisions.
更多查看译文
关键词
Column-and-constraint generation,distributionally robust optimization,extremal distribution,spatiotemporal correlation,wind power forecast
AI 理解论文
溯源树
样例
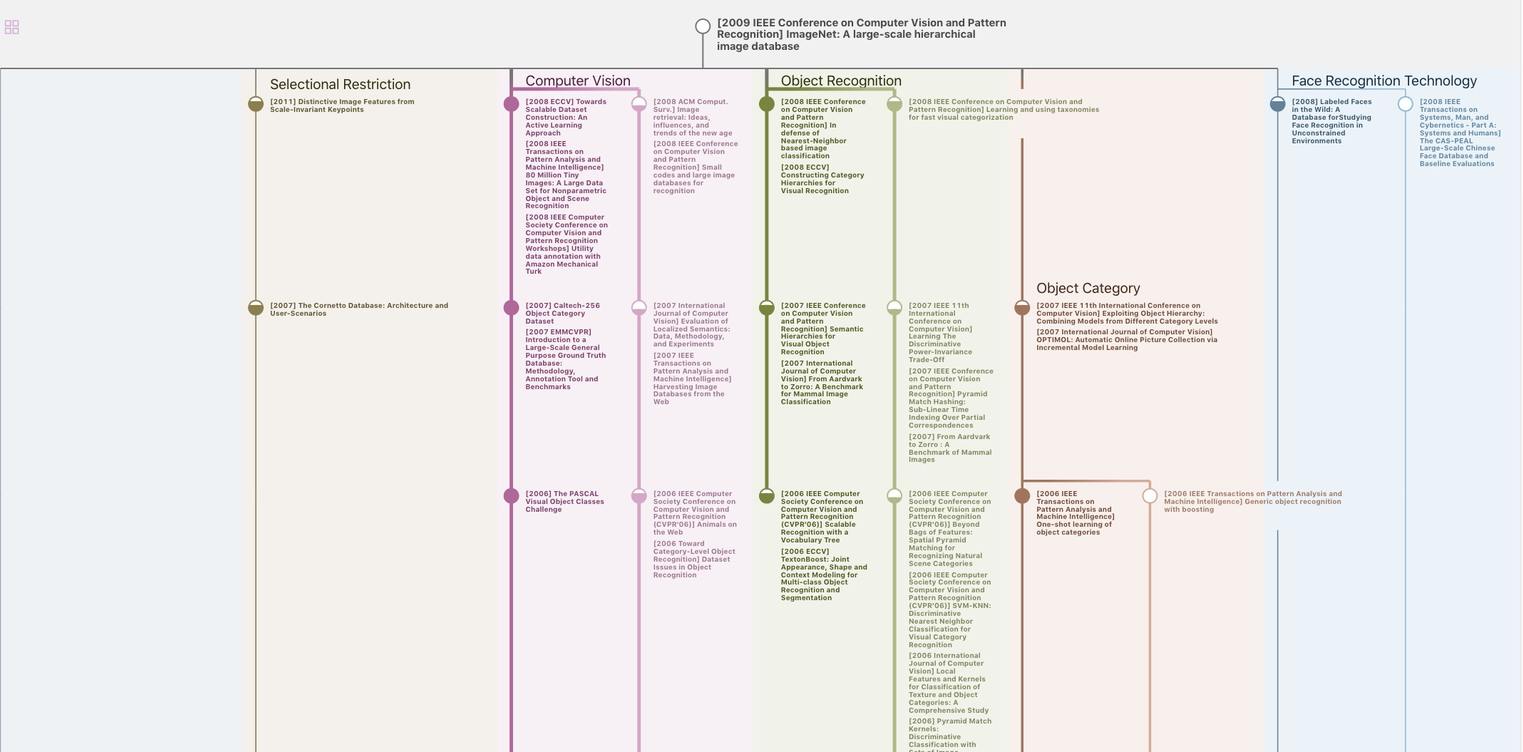
生成溯源树,研究论文发展脉络
Chat Paper
正在生成论文摘要