Dual-Channel Networks With Optimal-Band Selection Strategy for Arbitrary Cropped Hyperspectral Images Classification
IEEE GEOSCIENCE AND REMOTE SENSING LETTERS(2022)
摘要
The combination of the 2-D spatial information of the scene and 1-D spectral information proves to be an effective method for improving classification accuracy. However, the classification accuracy will be greatly reduced when the trained classification models are applied to arbitrary cropped hyperspectral images (HSIs). In an attempt to the above-mentioned problem, dual-channel networks with optimal-band selection (DNOS) is proposed. DNOS is composed of bidirectional recurrent neural network (RNN) branch for spectral features and multiscale convolutional neural network (CNN)-3D branch for spatial features. Besides, the optimal-band selection strategy is applied to distinguish characteristic bands of different ground objects. The spatial information is obtained via the texture feature method based on the optimal-band selection strategy. Texture-extracted spatial patches are sent to CNN-3D branch. Meanwhile, the spectrum is sent into the bidirectional RNN branch which can strengthen bands most relevant to ground object. The public hyperspectral data set shows that the DNOS model outperforms the other methods, especially in arbitrary cropped HSIs. Overall accuracy (OA), average accuracy (AA), and Kappa of the DNOS model are at least 8.3x0025;, 8.3x0025;, and 10.2x0025; than comparison methods in the letter.
更多查看译文
关键词
Feature extraction, Hyperspectral imaging, Recurrent neural networks, Training, Data models, Machine learning, Arbitrary cropped hyperspectral image (HSI), dual-channel deep learning (DL) networks, optimal-band selection
AI 理解论文
溯源树
样例
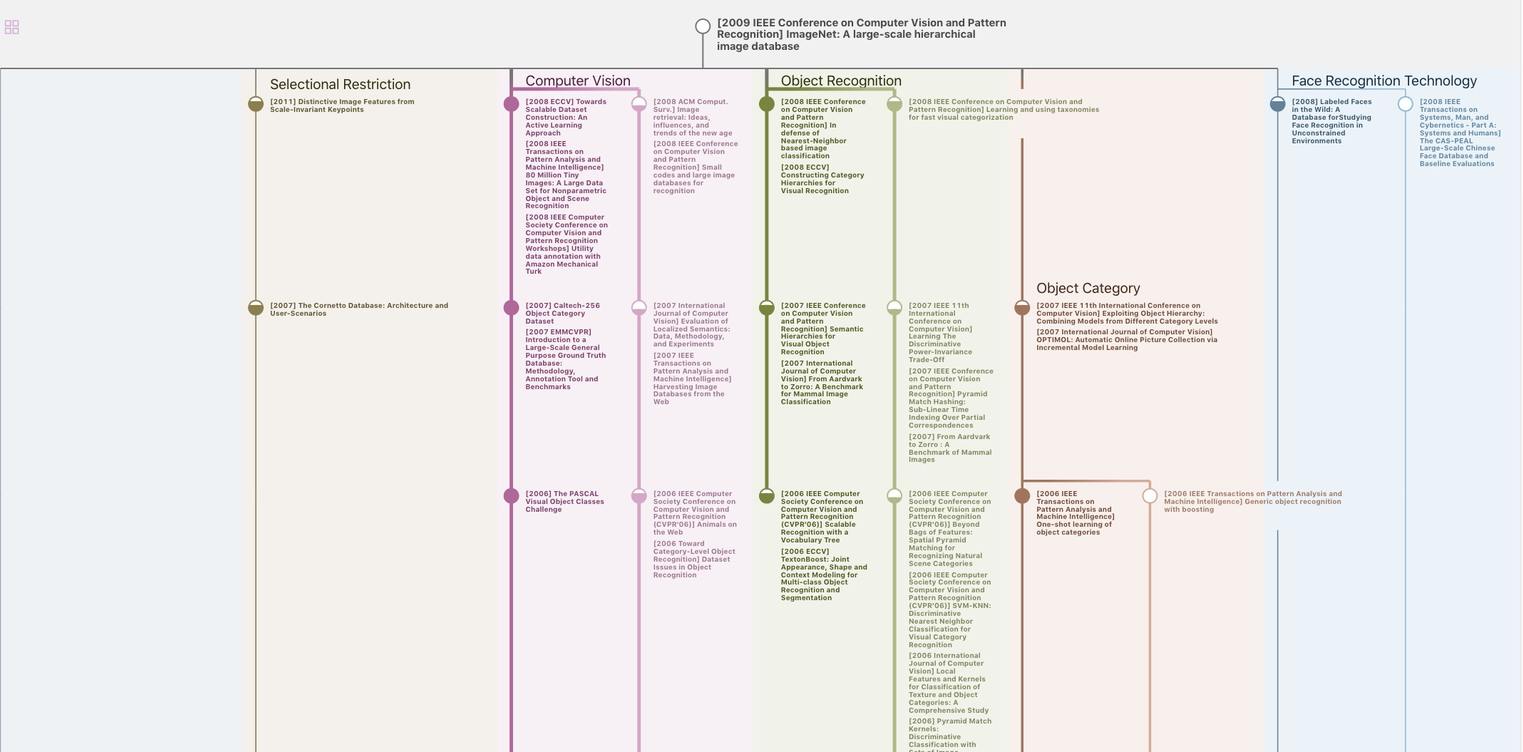
生成溯源树,研究论文发展脉络
Chat Paper
正在生成论文摘要