A Coupling Diagnosis Method For Sensor Faults Detection, Isolation And Estimation Of Gas Turbine Engines
ENERGIES(2020)
摘要
In this paper a novel fault detection, isolation, and identification (FDI&E) scheme using a coupling diagnosis method with the integration of the model-based method and unsupervised learning algorithm is proposed and developed for monitoring gas turbine sensor faults, which represents an integration of Square Root Cubature Kalman Filters (SRCKF) and an improved Density-Based Spatial Clustering of Application with Noise (DBSCAN) algorithm. A detection indicator produced by SRCKF with a specific hypothesis is used for extracting sensor fault features against process and measurement noise, as well as operating conditions. Then, an improved DBSCAN is implemented based on a voting scheme to detect and isolate the faulty sensors. Finally, a residual-based fault estimation scheme is proposed to track sensor fault evolution and help to judge the types of faults. Moreover, the observability of the model involved is analyzed to verify the stable operation of the FDI&E scheme. Various experiments for single and concurrent sensor fault scenarios in a dual-spool gas turbine prototype during a whole flight mission are conducted to demonstrate the effectiveness of the proposed FDI&E scheme. Moreover, comparative studies confirm the superiority of our proposed FDI&E scheme than the existing methods in terms of promptness and robustness of the sensor FDI.
更多查看译文
关键词
gas turbine, sensor fault diagnosis, machine learning, model based, Square Root Cubature Kalman Filter (SRCKF), Density-Based Spatial Clustering of Application with Noise (DBSCAN)
AI 理解论文
溯源树
样例
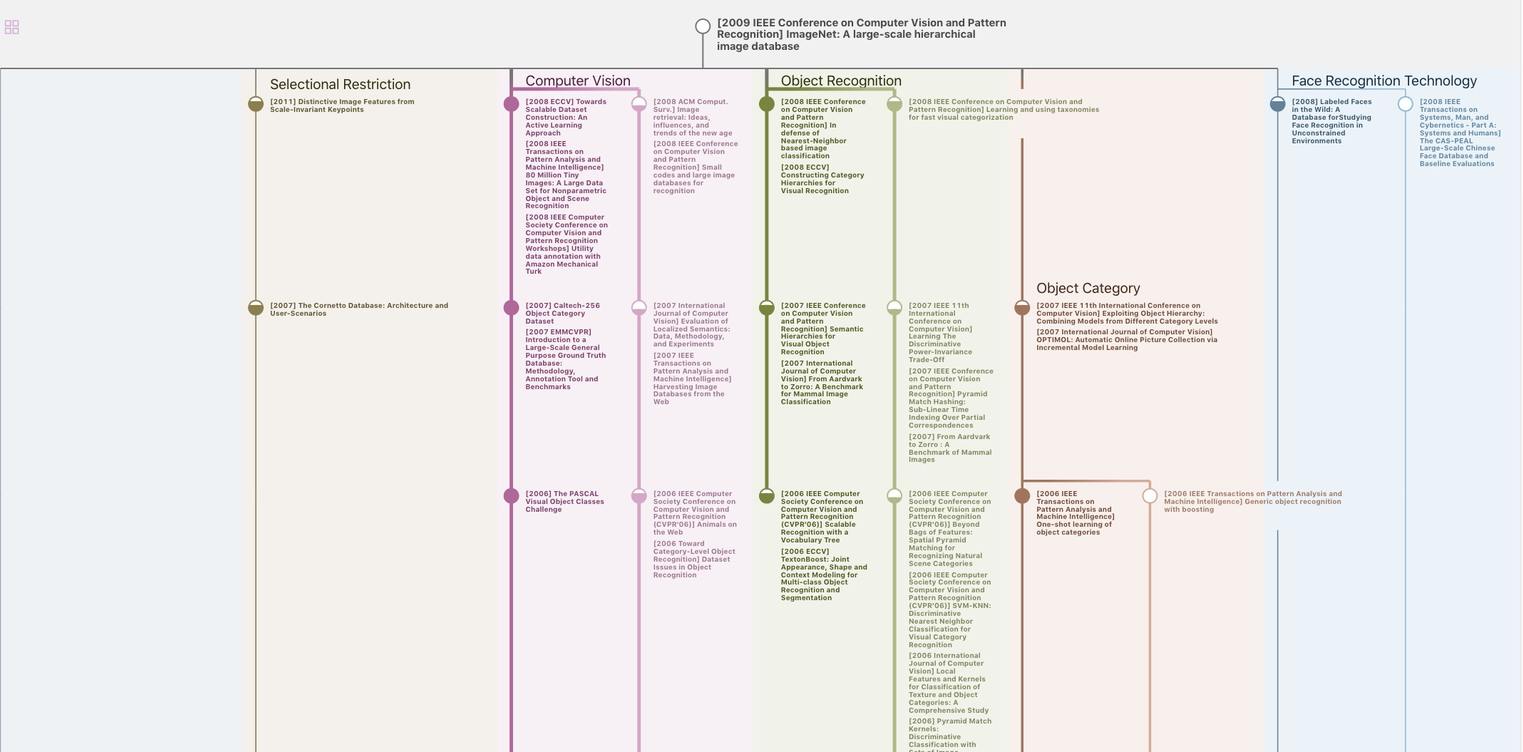
生成溯源树,研究论文发展脉络
Chat Paper
正在生成论文摘要