MoG-Based Robust Sparse Representation for Seismic Erratic Noise Suppression
IEEE GEOSCIENCE AND REMOTE SENSING LETTERS(2022)
摘要
By modeling the noise as Gaussian distribution, quite a lot of methods, such as basis pursuit denoising (BPDN), have demonstrated their great effectiveness in suppressing commonly random seismic noise. However, when it comes to complex seismic erratic noise, which designates non-Gaussian noise that consists of large isolated events with known or unknown distribution, such methods will lead to suboptimal results. In this letter, we present a mixture of Gaussian (MoG)-based robust sparse representation model for seismic erratic noise suppression. Instead of the Gaussian noise modeling, the mixture of Gaussian distribution is used to generally and perfectly fit the extremely complex distribution of seismic erratic noise, apart from the common Gaussian random noise. In addition, the Laplacian distribution is taken as a prior to model the robust and sparse representation of useful signal. In the Bayesian framework, our model can be constructed as a probabilistic maximum- posteriori probability (MAP) model and all the parameters can be easily estimated by expectation maximization (EM) and linearized Bregman (LB) algorithms. The experimental results on synthetic and real data show that the presented method can significantly suppress the erratic noise and well preserve the useful signal.
更多查看译文
关键词
Robustness, Transforms, Gaussian distribution, Laplace equations, Optimization, Noise reduction, Gaussian noise, Laplacian prior, mixture of Gaussian, noise modeling, seismic erratic noise suppression, sparse representation
AI 理解论文
溯源树
样例
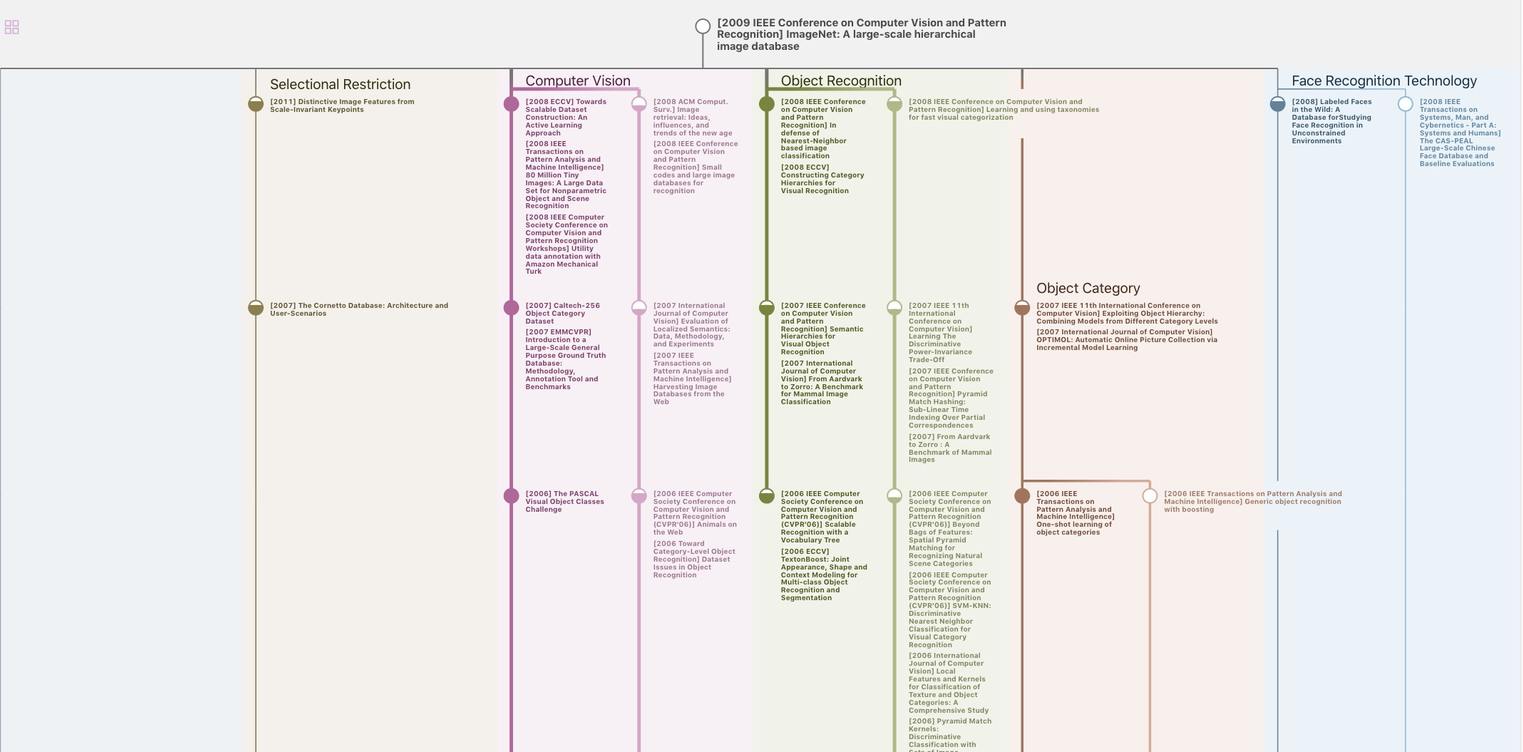
生成溯源树,研究论文发展脉络
Chat Paper
正在生成论文摘要