A New Text Classification Model Based on Contrastive Word Embedding for Detecting Cybersecurity Intelligence in Twitter
ELECTRONICS(2020)
摘要
Detecting cybersecurity intelligence (CSI) on social media such as Twitter is crucial because it allows security experts to respond cyber threats in advance. In this paper, we devise a new text classification model based on deep learning to classify CSI-positive and -negative tweets from a collection of tweets. For this, we propose a novel word embedding model, called contrastive word embedding, that enables to maximize the difference between base embedding models. First, we define CSI-positive and -negative corpora, which are used for constructing embedding models. Here, to supplement the imbalance of tweet data sets, we additionally employ the background knowledge for each tweet corpus: (1) CVE data set for CSI-positive corpus and (2) Wikitext data set for CSI-negative corpus. Second, we adopt the deep learning models such as CNN or LSTM to extract adequate feature vectors from the embedding models and integrate the feature vectors into one classifier. To validate the effectiveness of the proposed model, we compare our method with two baseline classification models: (1) a model based on a single embedding model constructed with CSI-positive corpus only and (2) another model with CSI-negative corpus only. As a result, we indicate that the proposed model shows high accuracy, i.e., 0.934 of F1-score and 0.935 of area under the curve (AUC), which improves the baseline models by 1.76 similar to 6.74% of F1-score and by 1.64 similar to 6.98% of AUC.
更多查看译文
关键词
cybersecurity intelligence,word embedding,deep learning,background knowledge,Twitter
AI 理解论文
溯源树
样例
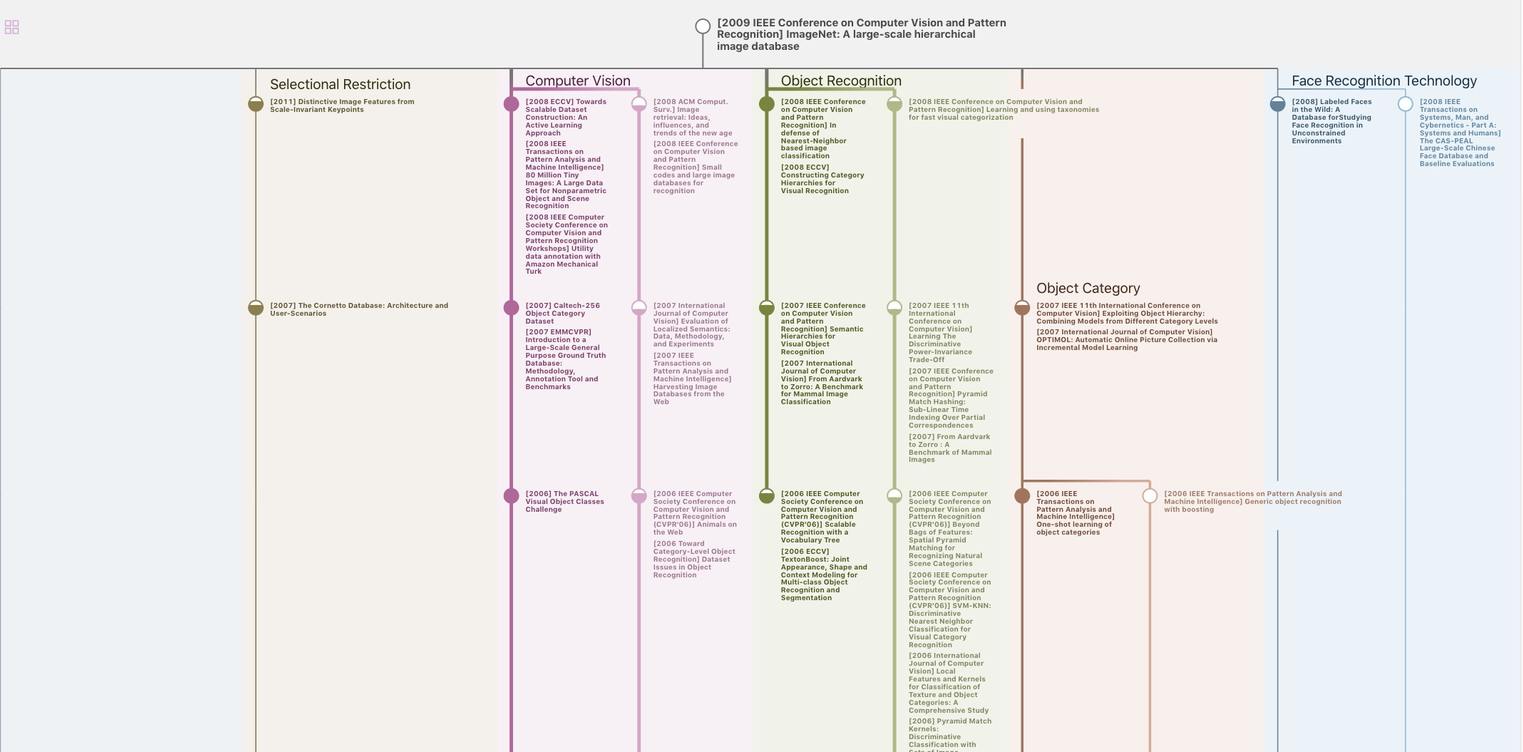
生成溯源树,研究论文发展脉络
Chat Paper
正在生成论文摘要