GDPC: generalized density peaks clustering algorithm based on order similarity
INTERNATIONAL JOURNAL OF MACHINE LEARNING AND CYBERNETICS(2020)
摘要
Clustering is a fundamental approach to discover the valuable information in data mining and machine learning. Density peaks clustering is a typical density based clustering and has received increasing attention in recent years. However DPC and most of its improvements still suffer from some drawbacks. For example, it is difficult to find peaks in the sparse cluster regions; assignment for the remaining points tends to cause Domino effect, especially for complicated data. To address the above two problems, we propose generalized density peaks clustering algorithm (GDPC) based on a new order similarity, which is calculated by the order rank of Euclidean distance between two samples. The order similarity can help us to find peaks in the sparse regions. In addition, a two-step assignment is used to weaken Domino effect. In general, GDPC can not only discover clusters in datasets regardless of different sizes, dimensions and shapes, but also address the above two issues. Several experiments on datasets, including Lung, COIL20, ORL, USPS, Mnist, breast and Vote, show that our algorithm is effective in most cases.
更多查看译文
关键词
Clustering,Order similarity,Density,Density peak,Graph
AI 理解论文
溯源树
样例
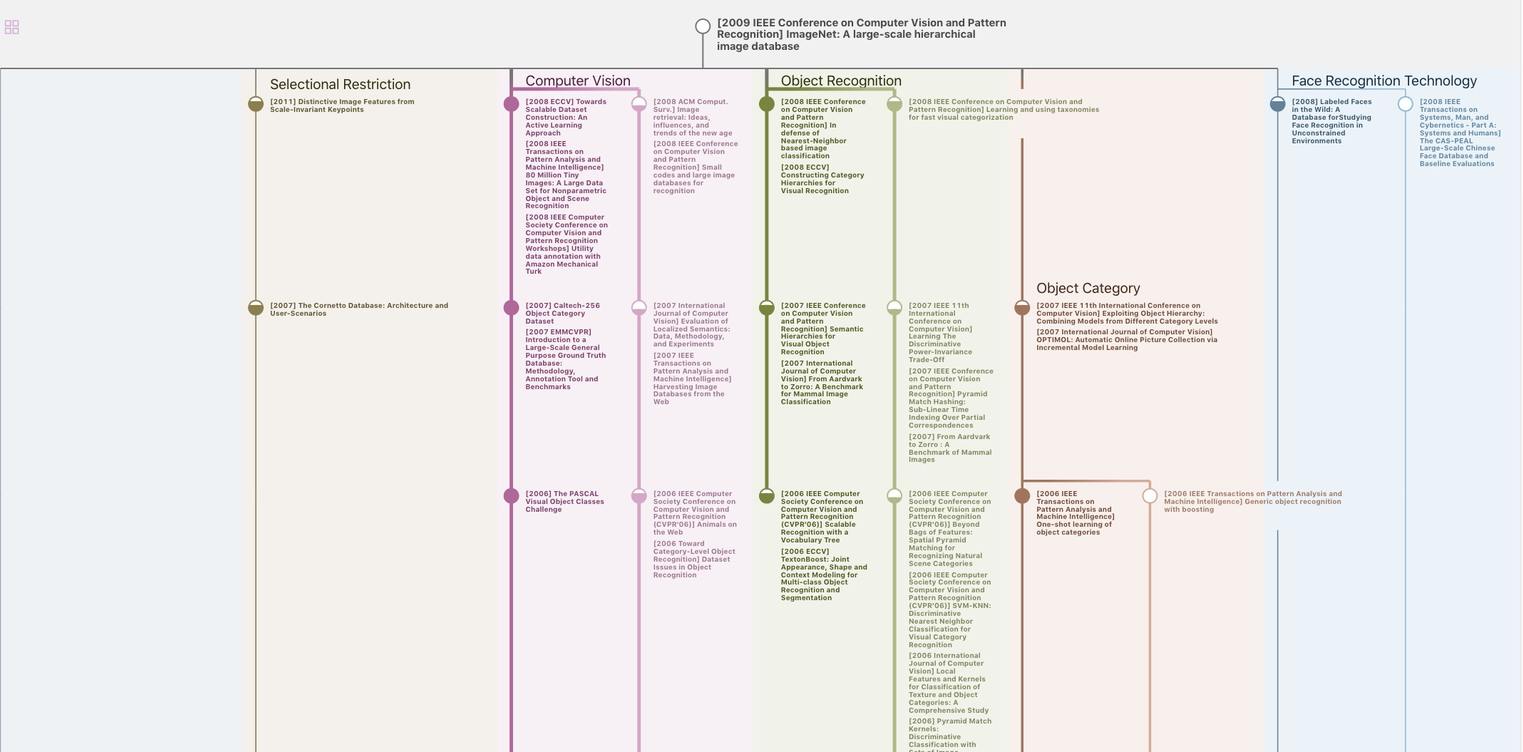
生成溯源树,研究论文发展脉络
Chat Paper
正在生成论文摘要