Data-Driven Prediction For The Number Of Distribution Network Users Experiencing Typhoon Power Outages
IET GENERATION TRANSMISSION & DISTRIBUTION(2020)
摘要
Typhoons have substantial impacts on power systems and may result in major power outages for distribution network users. Developing prediction models for the number of users going through typhoon power outages is a high priority to support restoration planning. This study proposes a data-driven model to predict the number of distribution network users that may experience power outages when a typhoon passes by. To improve the accuracy of the prediction model, twenty six explanatory variables from meteorological factors, geographical factors and power grid factors are considered. In addition, the authors compared the application effect of five different machine learning regression algorithms, including linear regression, support vector regression, classification and regression tree, gradient boosting decision tree and random forest (RF). It turns out that the RF algorithm shows the best performance. The simulation indicates that the accuracy of the optimal model error within +/- 30% can reach up to 86%. The proposed method can improve the prediction accuracy through continuous learning on the existing basis. The prediction results can provide efficient guidance for emergency preparedness during typhoon disaster, and can be used as a basis to notify the distribution network users who are likely to lose power.
更多查看译文
关键词
storms, support vector machines, regression analysis, disasters, power grids, decision trees, power system reliability, power engineering computing, random forests, gradient methods, pattern classification, power system planning, distribution networks, distribution network users, typhoon power outages, data-driven model, power grid factors, data-driven prediction, power systems, restoration planning, meteorological factors, geographical factors, machine learning regression algorithm, linear regression, support vector regression, classification, regression tree, gradient boosting decision tree, random forest
AI 理解论文
溯源树
样例
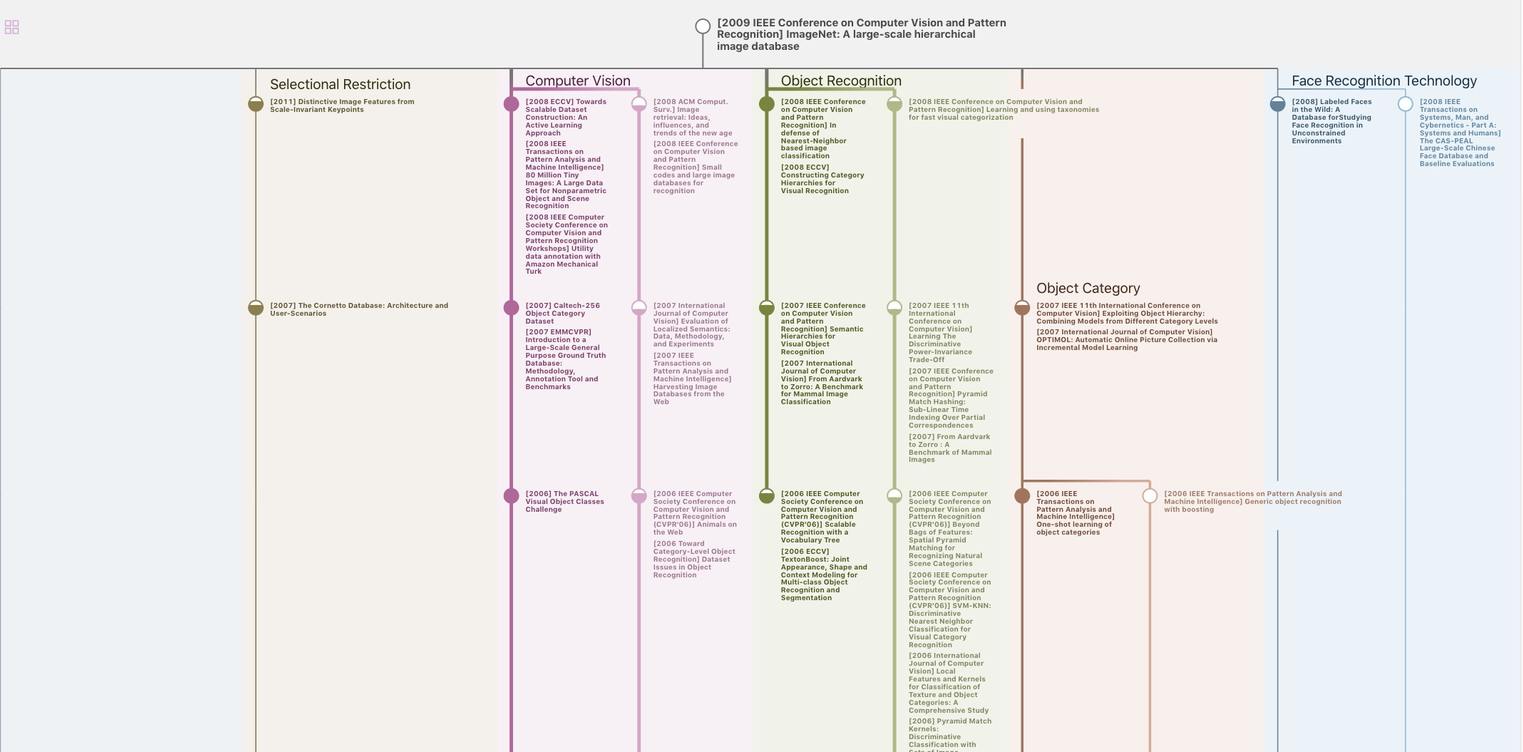
生成溯源树,研究论文发展脉络
Chat Paper
正在生成论文摘要