Statistical analysis of sparse approximate factor models
ELECTRONIC JOURNAL OF STATISTICS(2020)
摘要
We consider the problem of estimating sparse approximate factor models. In a first step, we jointly estimate the factor loading parameters and the error - or idiosyncratic - covariance matrix based on the Gaussian quasi-maximum likelihood method. Conditionally on these first step estimators, using the SCAD, MCP and Lasso regularisers, we obtain a sparse error covariance matrix based on a Gaussian QML and, as an alternative criterion, a least squares loss function. Under suitable regularity conditions, we derive error bounds for the regularised idiosyncratic factor model matrix for both Gaussian QML and least squares losses. Moreover, we establish the support recovery property, including the case when the regulariser is non-convex. These theoretical results are supported by empirical studies.
更多查看译文
关键词
Approximate factor analysis,non-convex regulariser,statistical consistency,support recovery
AI 理解论文
溯源树
样例
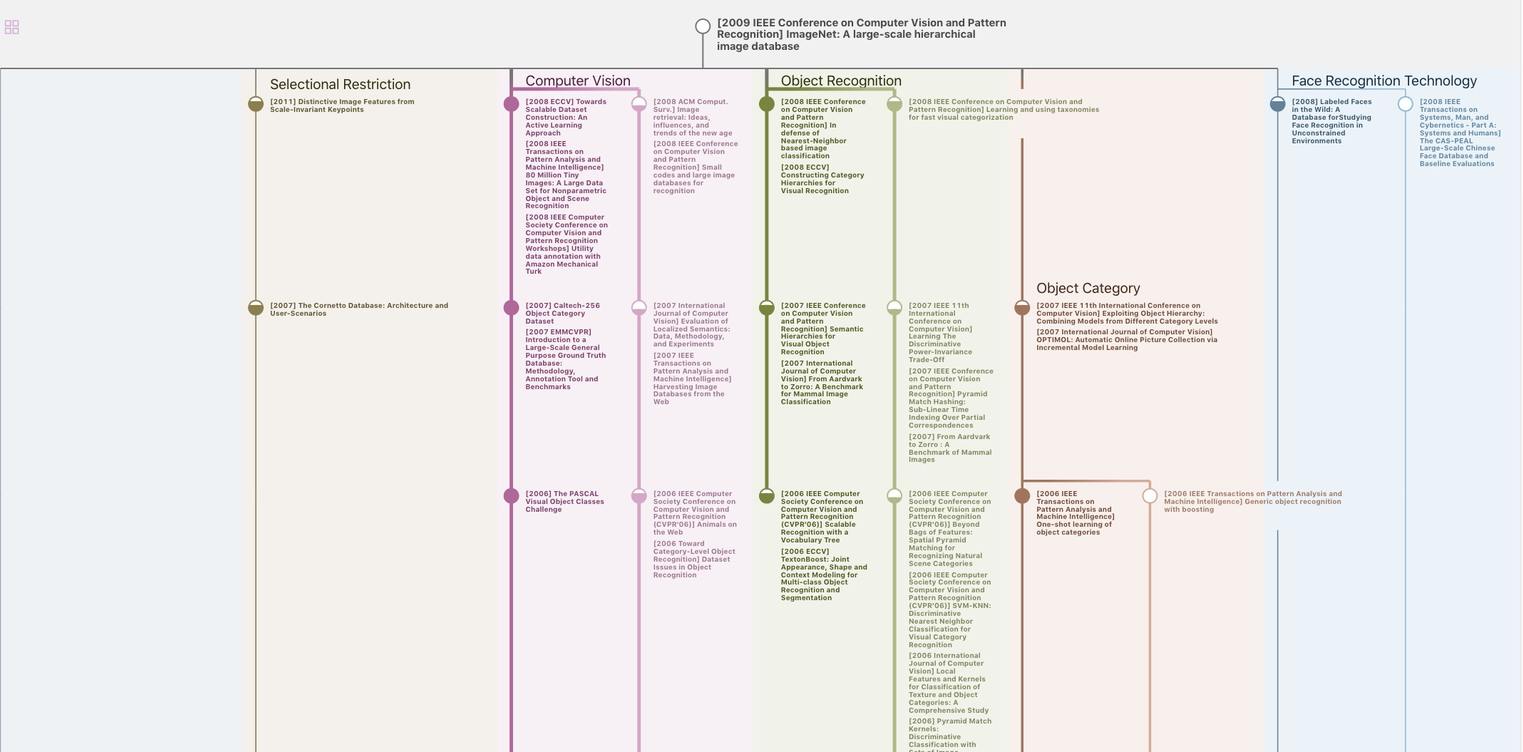
生成溯源树,研究论文发展脉络
Chat Paper
正在生成论文摘要