Machine Learning-Aided Parametrically Homogenized Crystal Plasticity Model (PHCPM) for Single Crystal Ni-Based Superalloys
JOM(2020)
摘要
This article establishes a multiscale modeling framework for the parametrically homogenized crystal plasticity model (PHCPM) for single crystal Ni-based superalloys. The PHCPMs explicitly incorporate morphological statistics of the γ -γ ' intragranular microstructure in their crystal plasticity constitutive coefficients. They enable highly efficient and accurate calculations for image-based polycrystalline microstructural simulations. The single crystal PHCPM development process involves: (1) construction of statistically equivalent RVEs or SERVEs, (2) image-based modeling with a dislocation-density crystal plasticity model, (3) identification of representative aggregated microstructural parameters, (4) selection of a PHCPM framework and (5) self-consistent homogenization. Novel machine learning tools are explored at every development phase. Supervised and unsupervised learning methods, such as support vector regression, artificial neural networks, k -means, and symbolic regression, enhanced optimization, model emulation and sensitivity analysis methods are all critical components of the multiscale modeling pipeline. The integration of machine learning tools with physics-based models enables the creation of powerful single crystal constitutive models for polycrystalline simulations.
更多查看译文
AI 理解论文
溯源树
样例
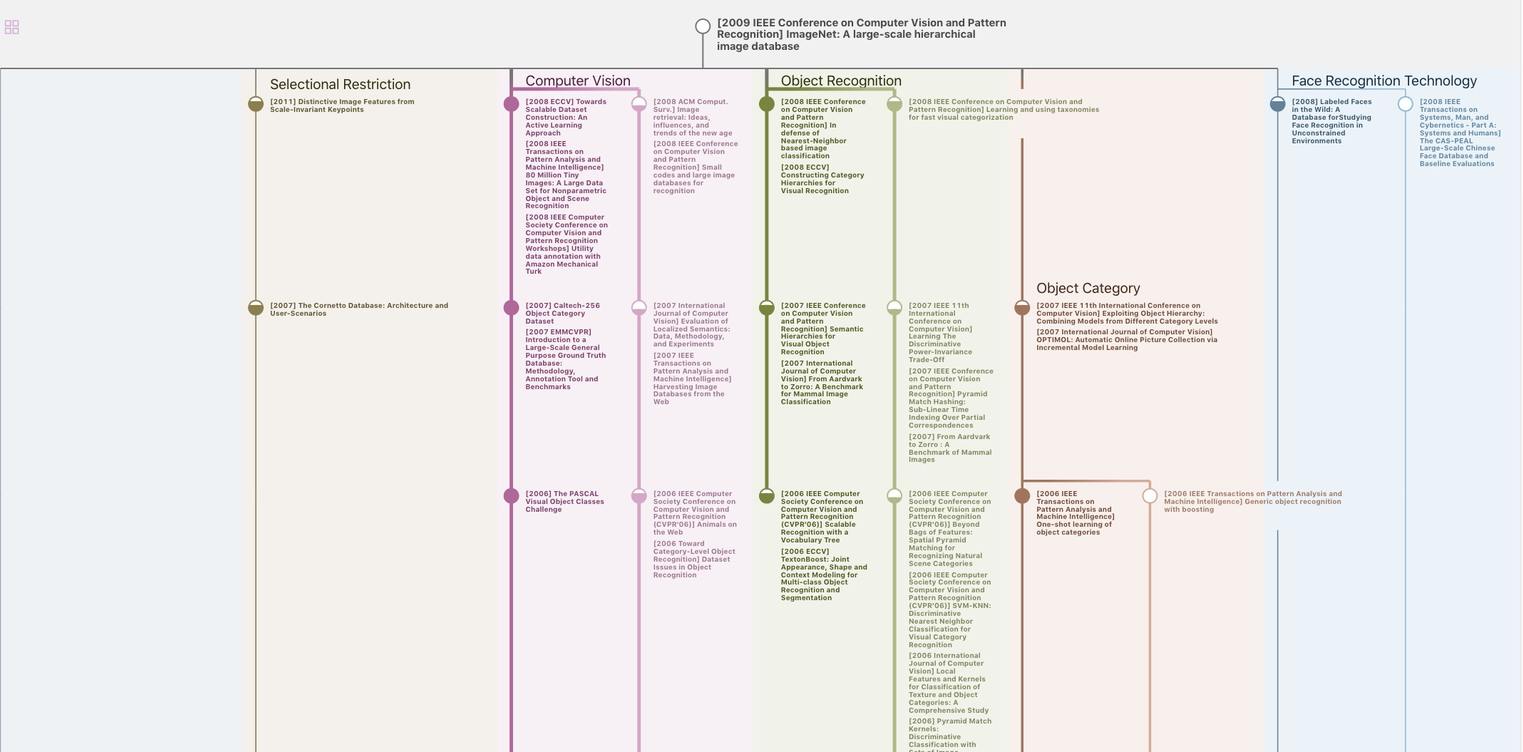
生成溯源树,研究论文发展脉络
Chat Paper
正在生成论文摘要