Deviance Tests For Graph Estimation From Multi-Attribute Gaussian Data
IEEE TRANSACTIONS ON SIGNAL PROCESSING(2020)
摘要
We consider the problem of inferring the conditional independence graph (CIG) of Gaussian vectors from multi-attribute data. Most existing methods for graph estimation are based on single-attribute models where one associates a scalar random variable with each node. In multi-attribute graphical models, each node represents a random vector. For single-attribute graphical models, considerable body of work exists where one first tests for exclusion of each edge from the saturated model, and then infers the CIG. In this paper, we propose and analyze a deviance test based on generalized likelihood ratio, for edge exclusion in multi-attribute Gaussian graphical models. The null distribution of the test statistic is derived to allow analytical calculation of the test threshold. An alternative computationally fast version of the deviance test statistic is also derived. Numerical results based on synthetic as well as real data are presented.
更多查看译文
关键词
Multi-attribute data, graphical model selection, undirected graph, generalized likelihood ratio test, deviance test
AI 理解论文
溯源树
样例
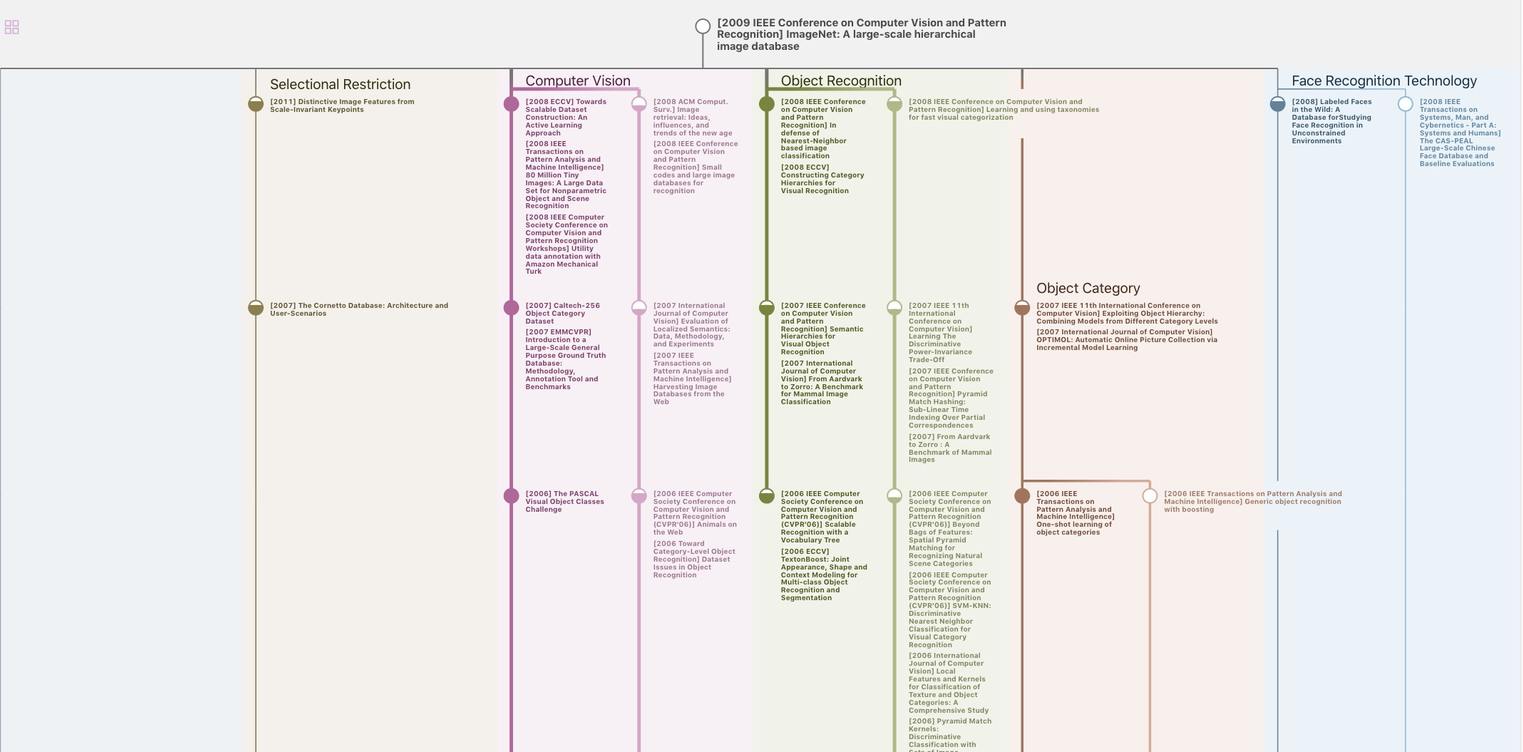
生成溯源树,研究论文发展脉络
Chat Paper
正在生成论文摘要