Distribution Adaptation Local Outlier Factor for Multimode Process monitoring
PROCEEDINGS OF THE 39TH CHINESE CONTROL CONFERENCE(2020)
摘要
In modern industrial processes, the production process includes multiple operating modes, due to changes in production goals and conditions. And the data generated in this process is a mixture of Gaussian and non-Gaussian distributions. Therefore, the data distribution of multimode processes is uncertain and complex. It is difficult to monitor multimode processes accurately by using traditional Multivariate Statistical Process Monitoring (MSPM) methods. In this case, the absence of labeled data is considered more valuable. This paper proposes a new unsupervised domain adaptive method called the Distribution Adaptation Local Outlier Factor (DALOF). This method can extract useful information from multimode data through domain adaptation, which can reduce the distribution difference of training data and improve the accuracy of modeling. In the DALOF model, data from different modes in the training set are defined as the source domain and the target domain. Weight the training data according to their correlation, and then project these data into a low-dimensional subspace. In this space, the distribution distance between the source and target domain is minimum. To solve the nonlinear characteristics of multimode data, this paper uses LOF to build a model and formed a density-based monitoring index. Numerical examples and Tennessee Eastman (TE) process simulation demonstrate the effectiveness of DALOF.
更多查看译文
关键词
Distribution Adaptation, Local Outlier Factor, Multimode Process, Process monitoring
AI 理解论文
溯源树
样例
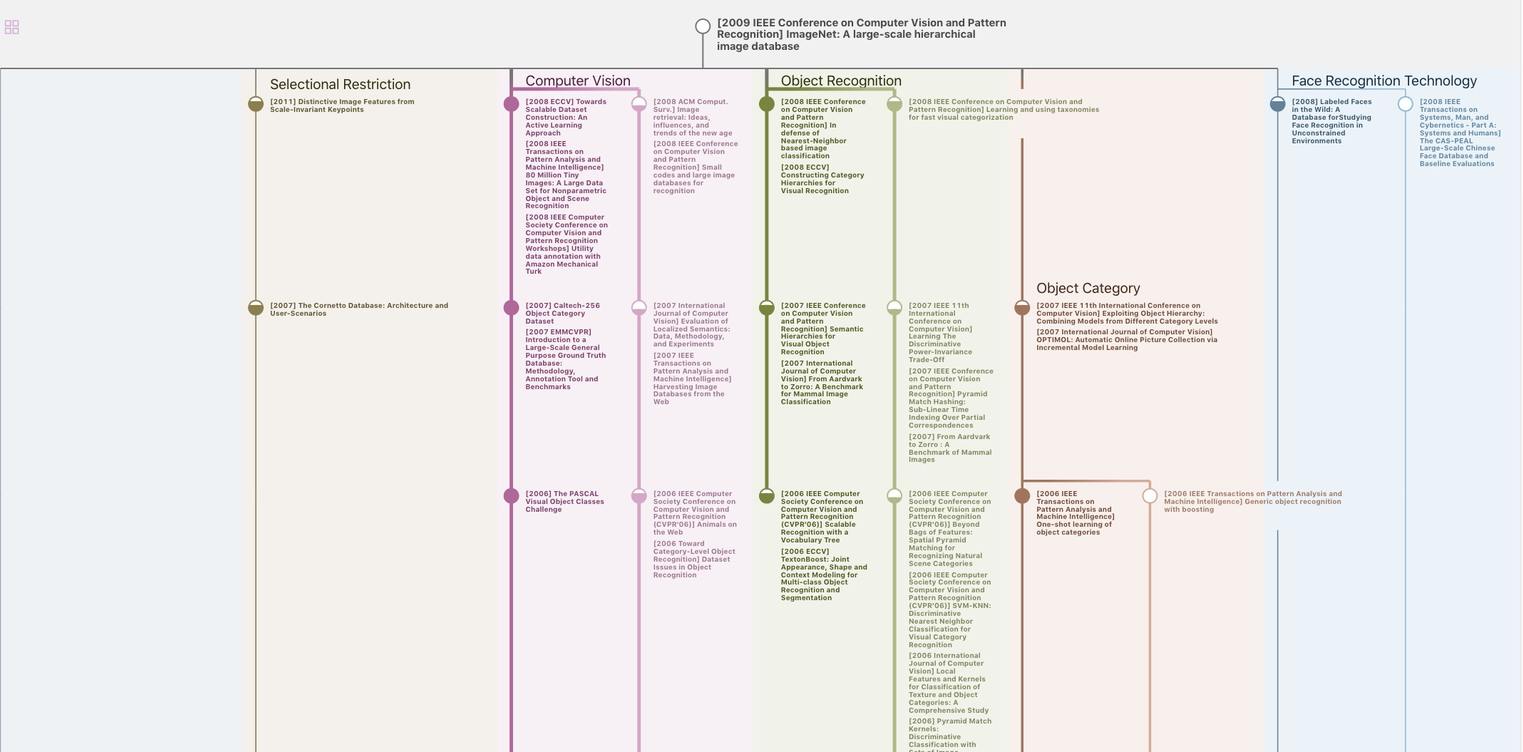
生成溯源树,研究论文发展脉络
Chat Paper
正在生成论文摘要