An Empirical Study for Adopting Machine Learning Approaches for Gas Pipeline Flow Prediction
MATHEMATICAL PROBLEMS IN ENGINEERING(2020)
摘要
As industrial control technology continues to develop, modern industrial control is undergoing a transformation from manual control to automatic control. In this paper, we show how to evaluate and build machine learning models to predict the flow rate of the gas pipeline accurately. Compared with traditional practice by experts or rules, machine learning models rely little on the expertise of special fields and extensive physical mechanism analysis. Specifically, we devised a method that can automate the process of choosing suitable machine learning algorithms and their hyperparameters by automatically testing different machine learning algorithms on given data. Our proposed methods are used in choosing the appropriate learning algorithm and hyperparameters to build the model of the flow rate of the gas pipeline. Based on this, the model can be further used for control of the gas pipeline system. The experiments conducted on real industrial data show the feasibility of building accurate models with machine learning algorithms. The merits of our approach include (1) little dependence on the expertise of special fields and domain knowledge-based analysis; (2) easy to implement than physical models; (3) more robust to environment changes; (4) requiring much fewer computation resources when it is compared with physical models that call for complex equation solving. Moreover, our experiments also show that some simple yet powerful learning algorithms may outperform industrial control problems than those complex algorithms.
更多查看译文
关键词
machine learning approaches,machine learning,prediction,flow
AI 理解论文
溯源树
样例
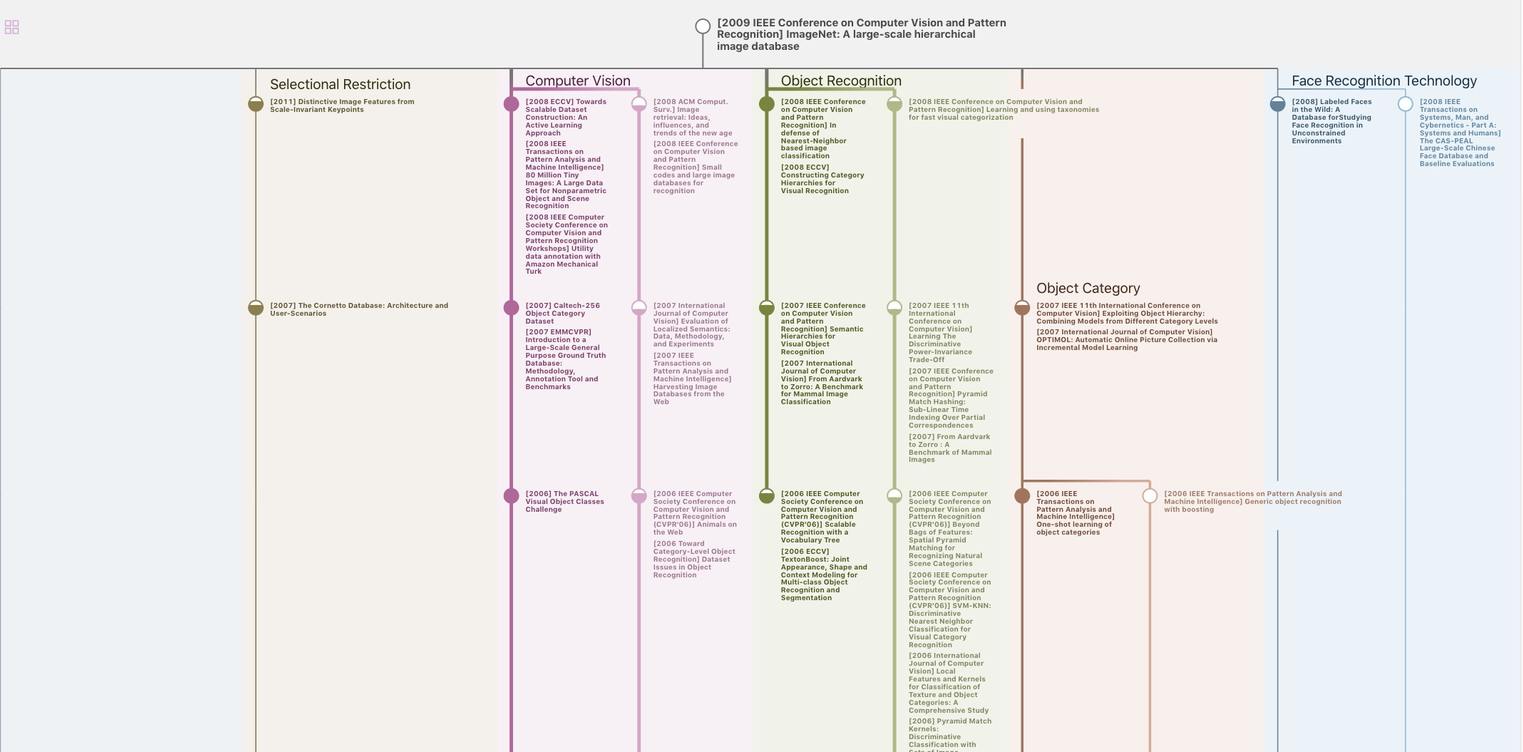
生成溯源树,研究论文发展脉络
Chat Paper
正在生成论文摘要