Potential of Recurrence Metrics from Sentinel-1 Time Series for Deforestation Mapping
IEEE JOURNAL OF SELECTED TOPICS IN APPLIED EARTH OBSERVATIONS AND REMOTE SENSING(2020)
摘要
The REDD+ framework requires accurate estimates of deforestation. These are derived by ground measurements supported by methods based on remote sensing data to automatically detect and delineate deforestations over large areas. In particular, in the tropics, optical data is seldom available due to cloud cover. As synthetic aperture radar (SAR) data overcomes this limitation, we performed a separability analysis of two statistical metrics based on the Sentinel-1 SAR backscatter over forested and deforested areas. We compared the range between the 5th and 95th temporal percentiles (PRange) and the recurrence quantification analysis (RQA) Trend metric. Unlike the PRange, the RQA Trend considers the temporal order of the SAR data acquisitions, thus contrasting between dropping backscatter signals and yearly seasonalities. This enables the estimation of the timing of deforestation events. We assessed the impact of polarization, acquisition orbit, and despeckling on the separability between forested and deforested areas and between different deforestation timings for two test sites in Mexico. We found that the choice of the orbit impacts the detectability of deforestation. In all cases, VH data showed a higher separability between forest and deforestations than VV data. The PRange slightly outperformed RQA Trend in the separation between forest and deforestation. However, the RQA Trend exceeded the PRange in the separation between different deforestation timings. In this study, C-Band backscatter data was used, although it is commonly not considered as the most suitable SAR dataset for forestry applications. Nevertheless, our approach shows the potential of dense C-Band backscatter time series to support the REDD+ framework.
更多查看译文
关键词
Forestry,Time series analysis,Synthetic aperture radar,Measurement,Market research,Backscatter,Optical sensors,Forestry,radar remote sensing,synthetic aperture radar,time series analysis
AI 理解论文
溯源树
样例
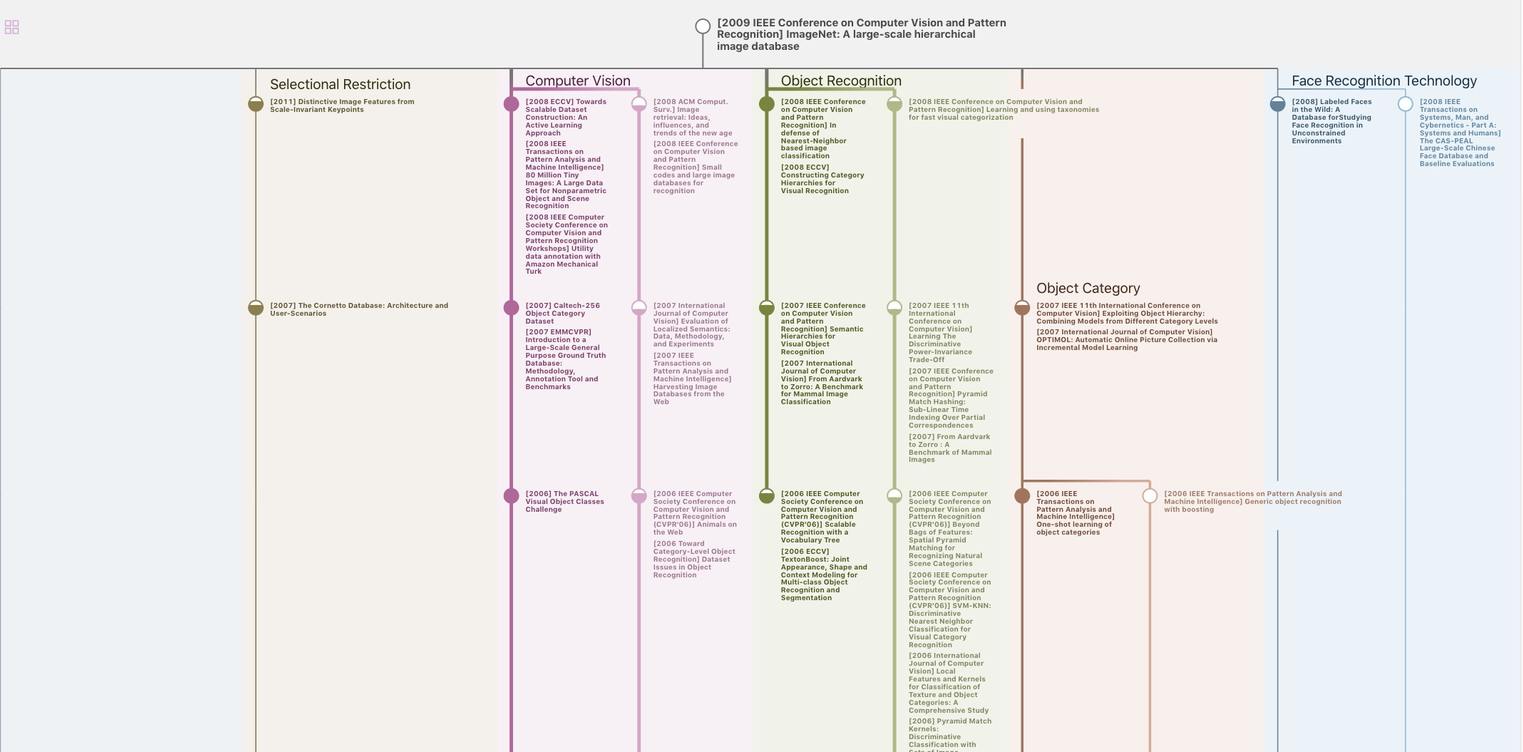
生成溯源树,研究论文发展脉络
Chat Paper
正在生成论文摘要