County level maize yield estimation using artificial neural network
MODELING EARTH SYSTEMS AND ENVIRONMENT(2020)
摘要
Maize yield estimates are useful for county food security preparedness. Techniques such as regression and simulation have been used by various studies to model and predict maize yield. This study used a feed-forward, back propagation artificial neural network with levenberg-marquardt algorithm for training. Artificial neural networks framework was chosen because its a data driven method that is relatively less widely used in county level yield prediction. Moreover, neural networks has key merits, such as require less formal statistical training, ability to detect nonlinear relationships by identifying likely interactions between variables and the availability of multiple training algorithms. We modelled historical maize yield between 2005–2016 as function of satellite derived precipitation, temperature, reference crop evapotranspiration, soil moisture and normalized difference vegetation index (NDVI) to predict maize yields at pixel level. The data were obtained with a spatial resolution of ≈ 4 km and subsequently, the predictions was done at ≈ 4 km pixel size. The historical reference maize yield data was divided into two sets for model training and validation. The model predicted maize yield with R^2 and root mean square error of 0.76 and 0.038 MT/ha in Trans-Nzoia county and 0.86 and 0.016 MT/ha, respectively, in Nakuru county. These findings shows a promising future for applications targeting to rapidly assess county level food preparedness in Kenya because maize is a major staple food.
更多查看译文
关键词
Boruta algorithm, Artificial neural networks, Machine learning, Maize yield prediction, Modeling, Remote sensing
AI 理解论文
溯源树
样例
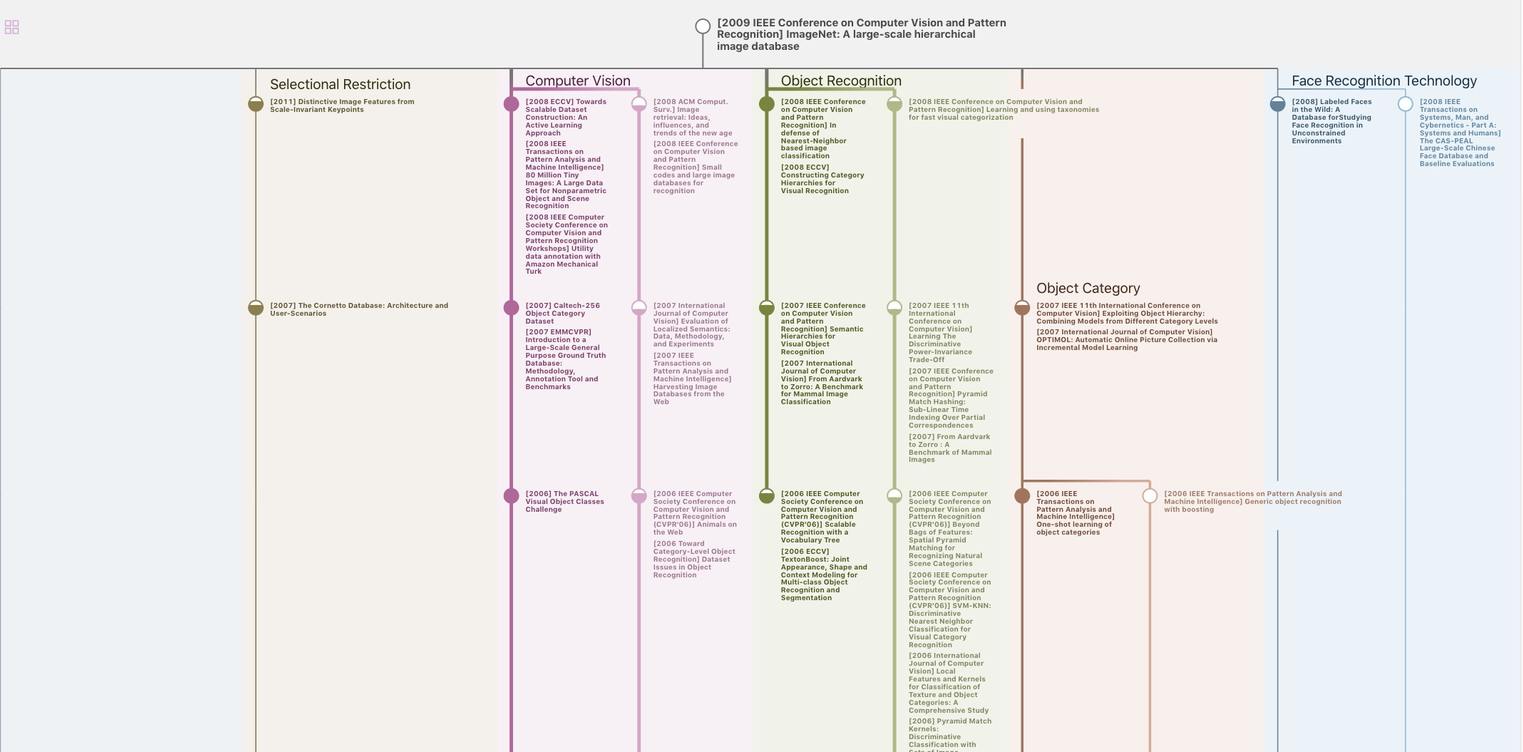
生成溯源树,研究论文发展脉络
Chat Paper
正在生成论文摘要