A kernel discriminant analysis for spatially dependent data
DISTRIBUTED AND PARALLEL DATABASES(2020)
摘要
We propose a novel supervised classification algorithm for spatially dependent data, built as an extension of kernel discriminant analysis, that we named Spatial Kernel Discriminant Analysis (SKDA). Our algorithm is based on a kernel estimate of the spatial probability density function, which integrates a second kernel to take into account spatial dependency of data. In fact, classical data mining algorithms assume that data samples are independent and identically distributed. However, this assumption is not verified when dealing with spatial data characterized by spatial autocorrelation phenomenon. To make an accurate analysis, it is necessary to exploit this rich source of information and to capture this property. We have applied our algorithm to a relevant domain, which consist of the classification of remotely sensed hyperspectral images. In order to assess the efficiency of our proposed method, we conducted experiments on two remotely sensed images datasets (Indian Pines and Pavia University) with different characteristics and scenarios. The experimental results show that our method is competitive and achieves higher classification accuracy compared to other contextual classification methods.
更多查看译文
关键词
Kernel density estimation, Kernel discriminant analysis, Spatial autocorrelation, Supervised classification, Hyperspectral images
AI 理解论文
溯源树
样例
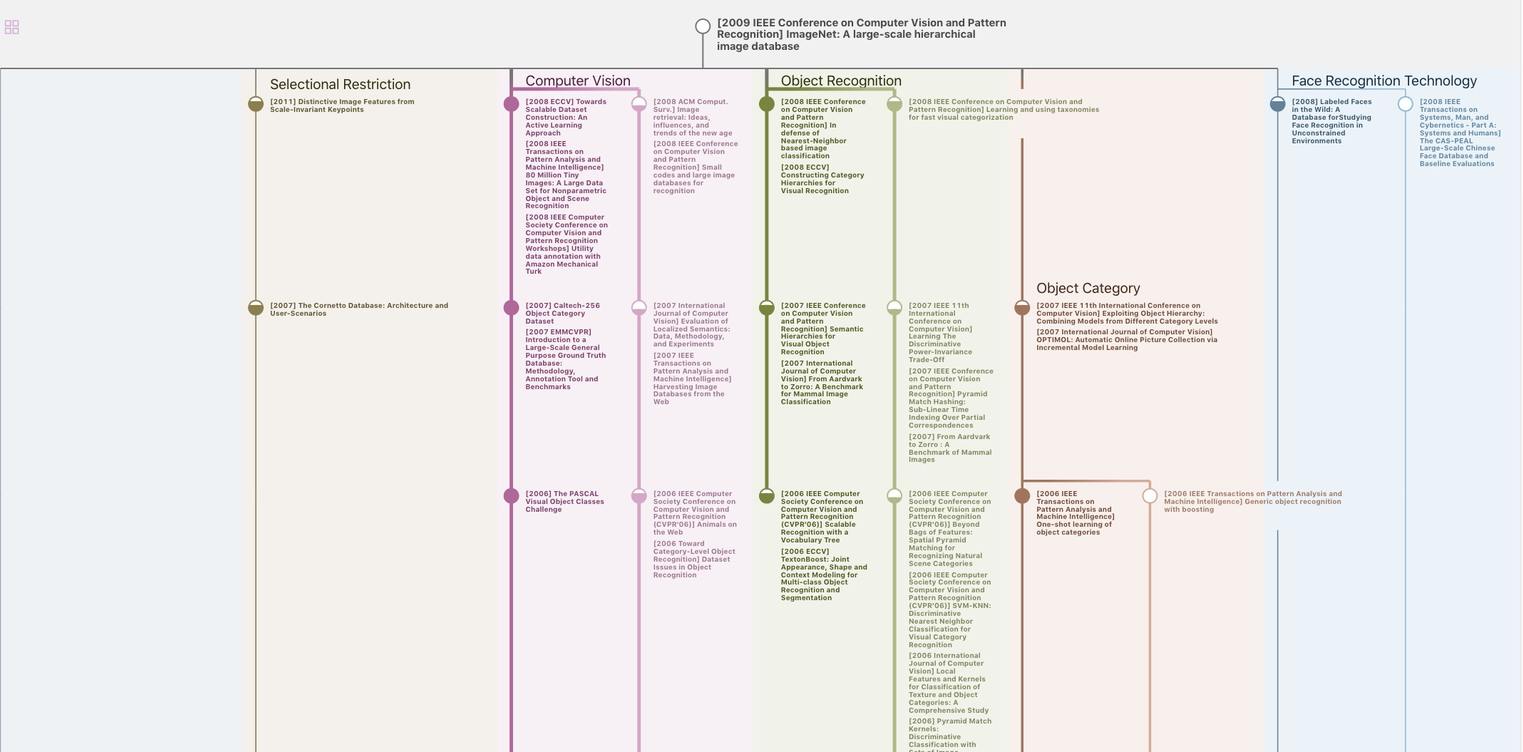
生成溯源树,研究论文发展脉络
Chat Paper
正在生成论文摘要