Hardware-Assisted Intellectual Property Protection Of Deep Learning Models
PROCEEDINGS OF THE 2020 57TH ACM/EDAC/IEEE DESIGN AUTOMATION CONFERENCE (DAC)(2020)
摘要
The protection of intellectual property (IP) rights of well-trained deep learning (DL) models has become a matter of major concern, especially with the growing trend of deployment of Machine Learning as a Service (MLaaS). In this work, we demonstrate the utilization of a hardware root-of-trust to safeguard the IPs of such DL models which potential attackers have access to. We propose an obfuscation framework called Hardware Protected Neural Network (HPNN) in which a deep neural network is trained as a function of a secret key and then, the obfuscated DL model is hosted on a public model sharing platform. This framework ensures that only an authorized end-user who possesses a trustworthy hardware device (with the secret key embedded on-chip) is able to run intended DL applications using the published model. Extensive experimental evaluations show that any unauthorized usage of such obfuscated DL models result in significant accuracy drops ranging from 73.22 to 80.17% across different neural network architectures and benchmark datasets. In addition, we also demonstrate the robustness of proposed HPNN framework against a model fine-tuning type of attack.
更多查看译文
关键词
HPNN framework,hardware-assisted intellectual property protection,hardware protected neural network,authorized end-user,public model sharing platform,deep neural network,obfuscation framework,hardware root-of-trust,deep learning models,IP,intellectual property rights,model fine-tuning type,neural network architectures,obfuscated DL model,published model,secret key,trustworthy hardware device
AI 理解论文
溯源树
样例
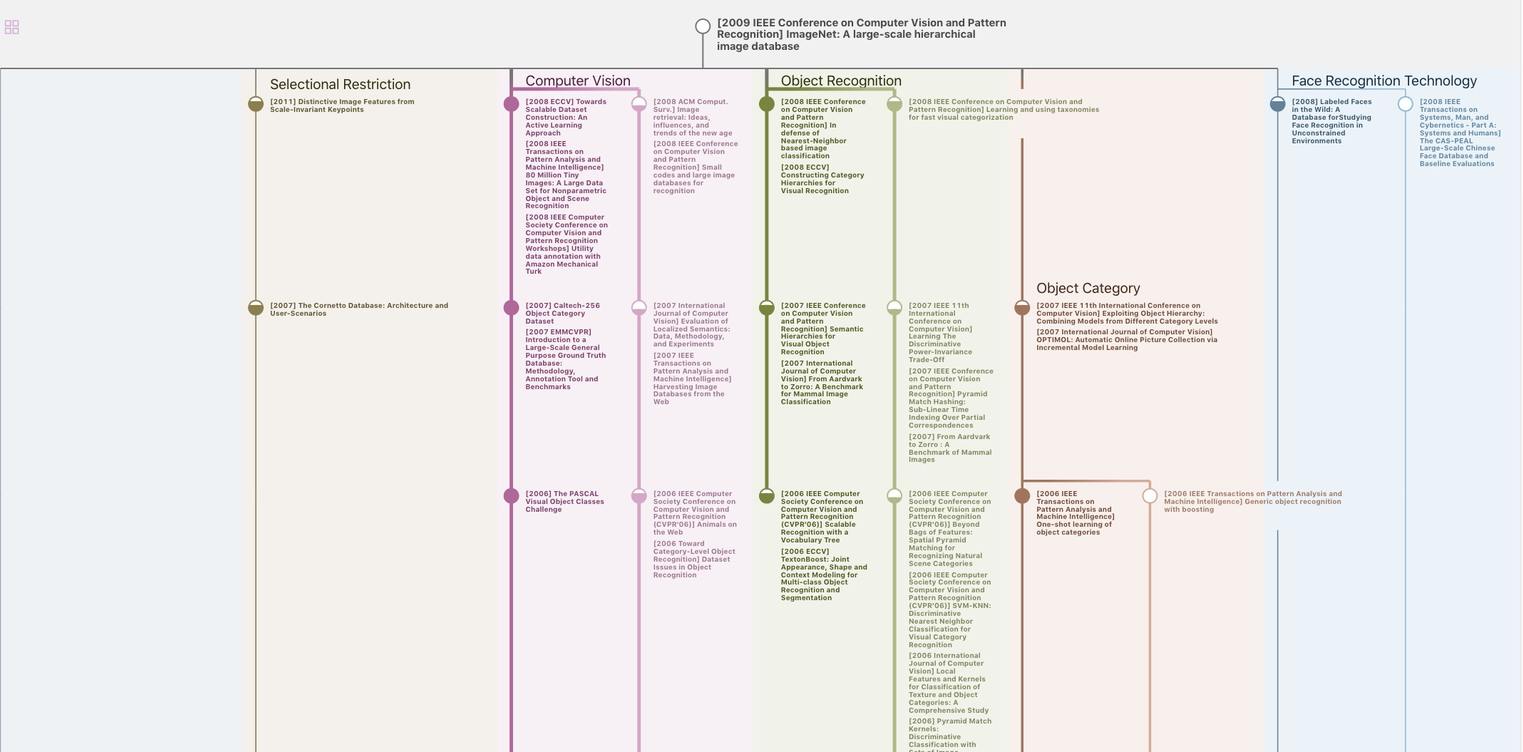
生成溯源树,研究论文发展脉络
Chat Paper
正在生成论文摘要