Postprocessing framework for land cover classification optimization based on iterative self-adaptive superpixel segmentation
JOURNAL OF APPLIED REMOTE SENSING(2020)
摘要
An increasing number of applications require land cover information from remote sensing images, thereby resulting in an urgent demand for automatic land use and land cover classification. Therefore, effectively improving the accuracy of land cover classification is a main objective in remote sensing image processing. We propose a land cover classification postprocessing framework based on iterative self-adaptive superpixel segmentation (LCPP-ISSS) for remote sensing image data. This framework can further optimize the land cover classification results obtained by neural networks without changing the network structure. First, we propose the iterative self-adaptive superpixel segmentation algorithm for high-resolution remote sensing images to extract the boundary information of different land cover classes. Then, we propose a land cover classification result optimization method based on patch complexity to optimize the classification result by combining the boundary information with the semantic information. In an experiment, we compare the classification accuracy before and after using LCPP-ISSS and with other common methods. The results show that LCPP-ISSS outperforms the dense conditional random field and provides a 4% increase in the mean intersection over union and a 10% increase in overall accuracy. (C) The Authors. Published by SPIE under a Creative Commons Attribution 4.0 Unported License.
更多查看译文
关键词
remote sensing,high resolution,land cover classification,postprocessing,iterative,self-adaptive
AI 理解论文
溯源树
样例
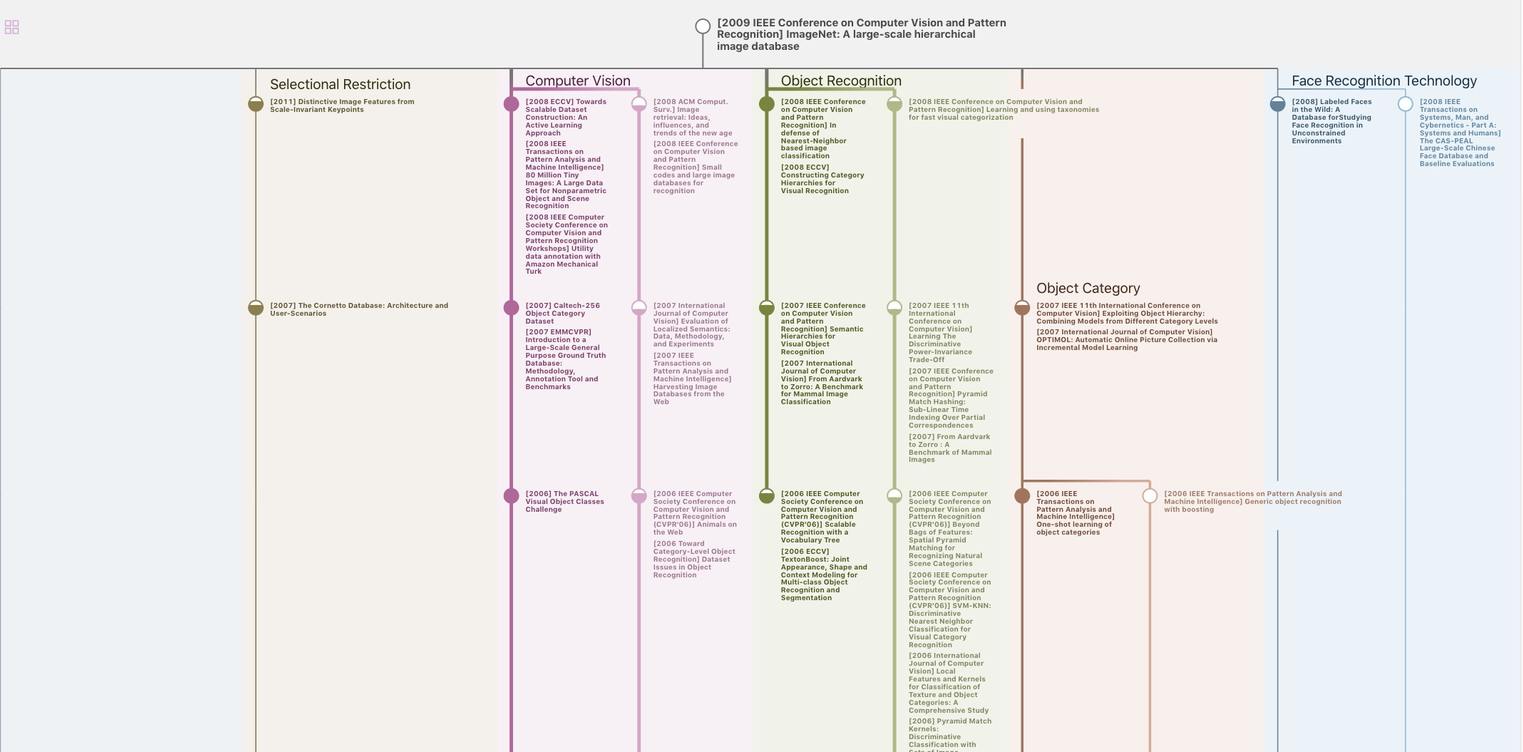
生成溯源树,研究论文发展脉络
Chat Paper
正在生成论文摘要