Terrain Identification for Humanoid Robots Applying Convolutional Neural Networks
IEEE/ASME Transactions on Mechatronics(2021)
摘要
Stable and efficient walking strategies for humanoid robots usually relies on assumptions regarding terrain characteristics. If the robot is able to classify the ground type at the footstep moment, it is possible to take preventive actions to avoid falls and to reduce energy consumption. In this article, a new terrain identification method that makes use of a convolutional neural network to classify the terrain type is proposed. The input of this network is a bidimensional matrix-a signature image of the impact-composed of raw data from inertial and torque sensors, sampled after the impact between the foot and ground. Six different terrains types were selected for testing the proposed approach: these terrains were tested both in simulation using the Gazebo 9.0 environment and with one real robot, designed to satisfy the requirements for the RoboCup Humanoid Competition. In both robots the ROS framework was used, and the LIPM was the selected approach for the biped walking pattern generation. Results showed that the proposed method was able to classify the terrain type with an accuracy greater than 98% for both real and virtual situations, surpassing current state-of-the-art terrain classification methods for legged robots.
更多查看译文
关键词
Mobile robots,neural network applications,robot sensing systems,terrain classification
AI 理解论文
溯源树
样例
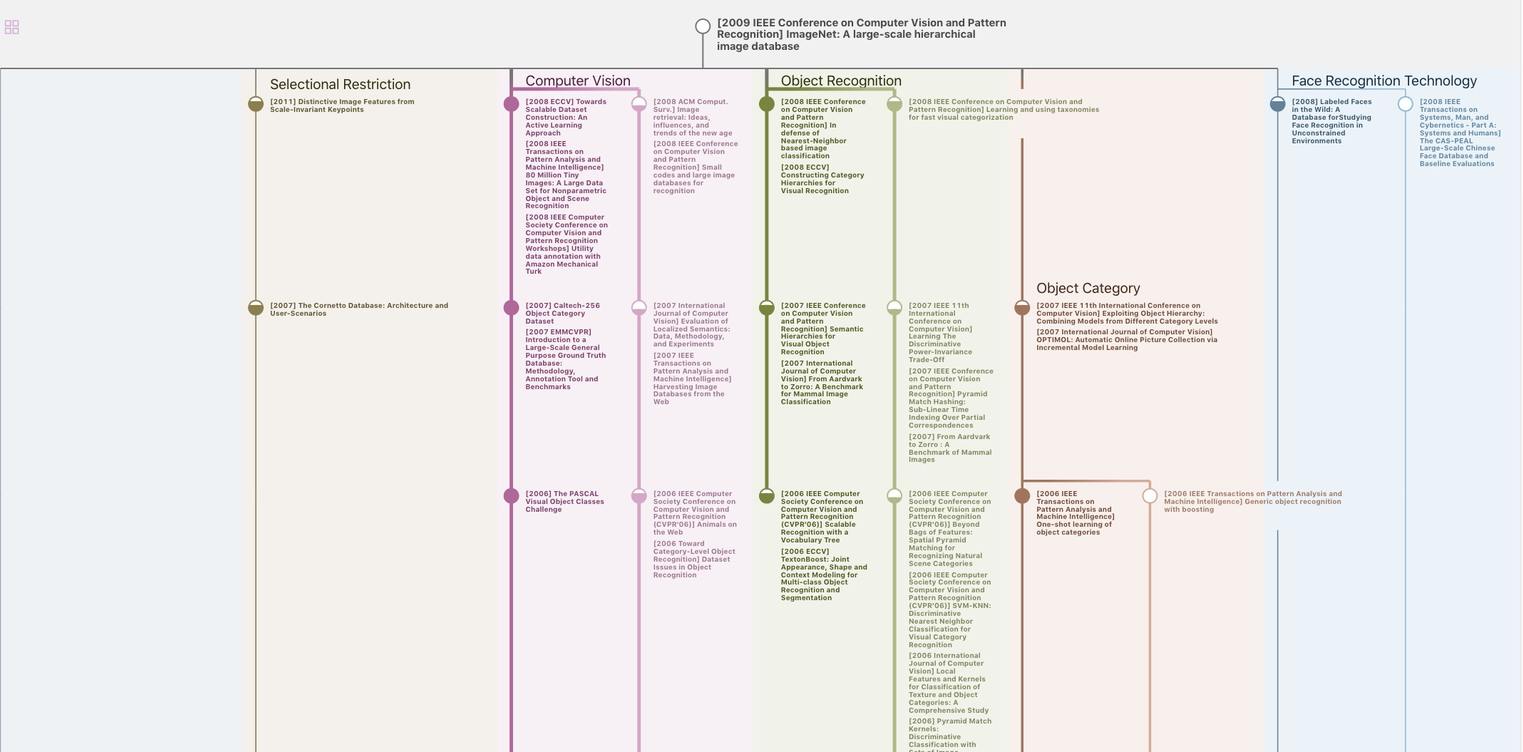
生成溯源树,研究论文发展脉络
Chat Paper
正在生成论文摘要