Efficient Insertion Control for Precision Assembly Based on Demonstration Learning and Reinforcement Learning
IEEE Transactions on Industrial Informatics(2021)
摘要
Multiple peg-in-hole insertion control is one of the challenging tasks in precision assembly for its complex contact dynamics. In this article, an insertion policy learning method is proposed for multiple peg-in-hole precision assembly. The insertion policy learning process is separated into two phases: initial policy learning and residual policy learning. In initial policy learning, a state-to-action policy mapping model based on the Gaussian mixture model (GMM) is established. And Gaussian mixture regression (GMR) is used to generalize the policy reuse. In residual policy learning, a reinforcement learning method named normalized advantage function (NAF) is employed to refine the insertion policy via agent's exploration in the insertion environment. Moreover, an adaptive action exploration (AAE) strategy is designed to improve the performance of exploration, and the prioritized experience replay strategy is introduced to make the residual policy learning from historical experience more efficient. Besides, the hierarchical reward function is designed considering the contact dynamics as well as the efficiency and safety of precision insertion. Finally, comprehensive experiments are conducted to validate the effectiveness of the proposed insertion policy learning method.
更多查看译文
关键词
Learning (artificial intelligence),Task analysis,Learning systems,Gaussian distribution,Informatics,Automation,Data models
AI 理解论文
溯源树
样例
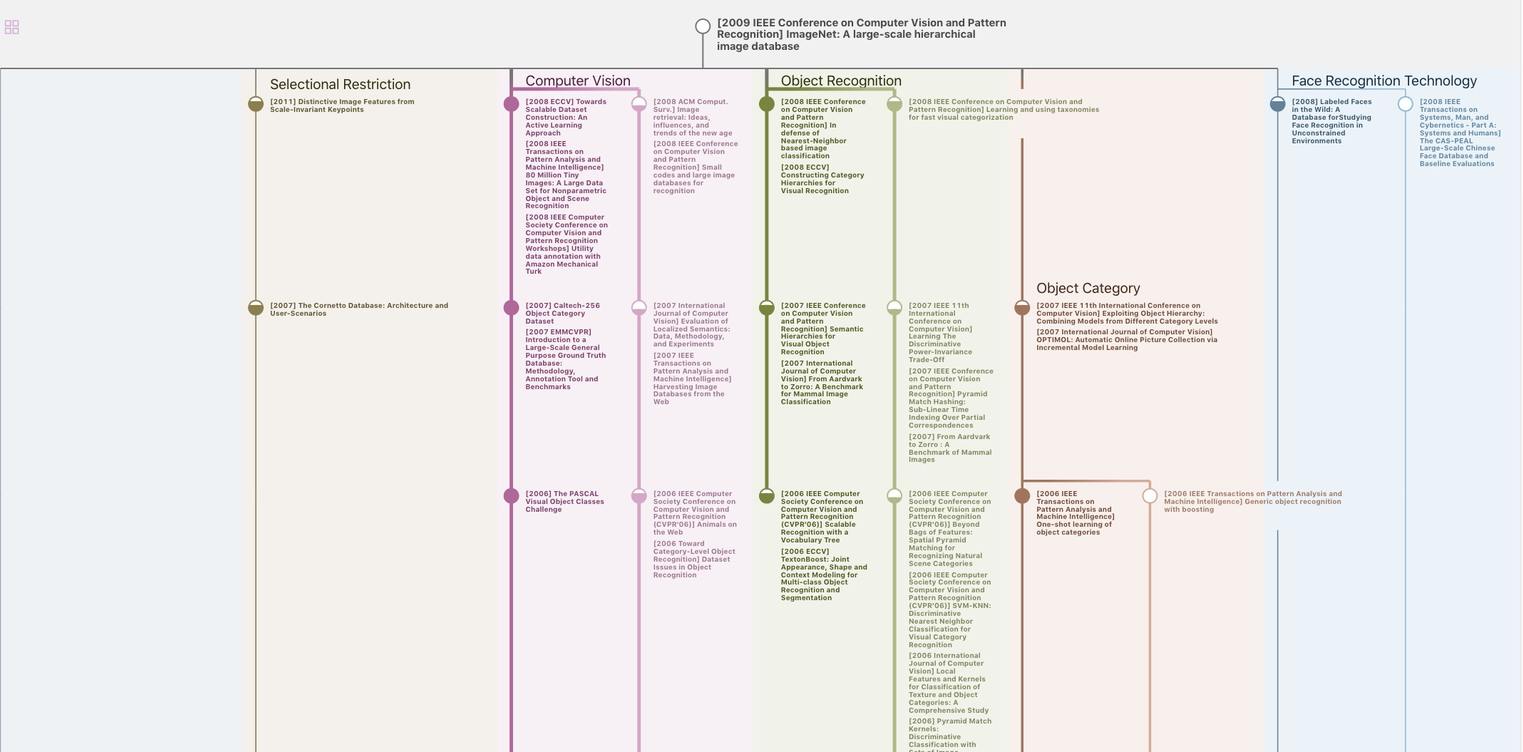
生成溯源树,研究论文发展脉络
Chat Paper
正在生成论文摘要